A Novel Cross-Domain Intelligent Fault Diagnosis Method Based on Entropy Features and Transfer Learning
IEEE TRANSACTIONS ON INSTRUMENTATION AND MEASUREMENT(2021)
摘要
Using transfer learning (TL) for fault detection and diagnosis has been a hot topic in prognostic and health management (PHM) field. In this article, a systematic framework is established to solve the cross-domain fault diagnosis for rotary machines of the same type using entropy-based TL. The multiscale symbolic dynamic entropy (MSDE) is first proposed to extract features from vibration signals. Then, TL model is trained to get a mappingmatrix, which can preserve the structure properties of prior distribution and minimize the discrepancies between different datasets. The mapped features are called multiscale transfer symbolic dynamic entropy (MTSDE). Finally, the support vector machine (SVM) classifier is utilized to accomplish the cross-domain intelligent fault-type recognition. To demonstrate the superiority of the proposed MTSDE method, the comparative experiments of nine methods are carried out under different datasets including gearboxes and bearings. Experimental results demonstrate that our proposed MTSDE method performs best in recognizing various fault types comparing with other nine methods. To the best knowledge of the authors, this is the first attempt of using entropy-based TL for cross-domain fault diagnosis of rotating machinery.
更多查看译文
关键词
Entropy method, fault diagnosis, locality preserving projection (LPP), rotating machines, transfer learning (TL)
AI 理解论文
溯源树
样例
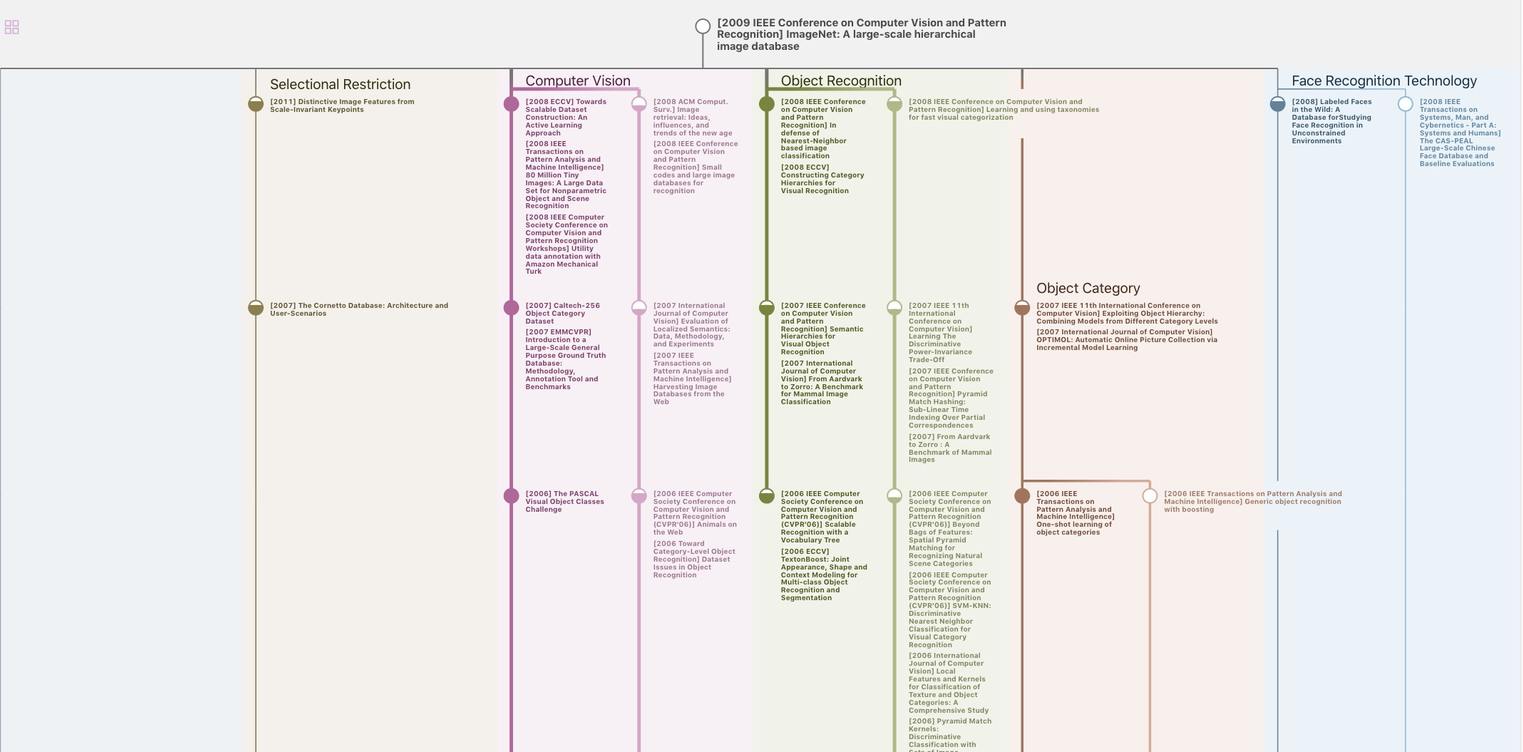
生成溯源树,研究论文发展脉络
Chat Paper
正在生成论文摘要