PairCon-SLAM: Distributed, Online, and Real-Time RGBD-SLAM in Large Scenarios
IEEE TRANSACTIONS ON INSTRUMENTATION AND MEASUREMENT(2021)
摘要
This article proposed a PairCon-simultaneous localization and mapping algorithm to construct dense maps of large-scale scenarios in real time, which is operated in an integral platform containing two personal computers (PCs). This platform can operate the mapping thread independently in a PC to guarantee sufficient memory resources. In this context, the synchronous visualization of 3-D maps can be achieved in such a platform. In contrast, traditional algorithms are limited by the short operation distance, under the requirement of simultaneous data collection and 3-D maps construction in a PC. In addition, the memory resource provided by a single PC is limited, which restricts the constructing scale of maps. Thus, we use a separate PC to construct maps independently to relieve the distance constraint and exploit the socket method to conduct the transmission of data with the point cloud. Meanwhile, we introduce the ReBlur algorithm into the semi-direct method to reduce the error accumulation of the odometer in the tracking thread, which improves the robustness performance. In addition, the method combining the memory management and DBow2 algorithm is adopted to improve the accuracy of loop detection. In the considered system, the quality of maps and the performance of the odometer are evaluated by ICL-NUIM and the datasets, such as TUM, DIODE, and so on, respectively. Finally, under the simulation environment of AirSim and Gazebo, we construct maps based on the image data of other scenarios, which is used to show the quality of the construction.
更多查看译文
关键词
Big-scale-scene, dataset, mapping, RGBD-simultaneous localization and mapping (SLAM), socket
AI 理解论文
溯源树
样例
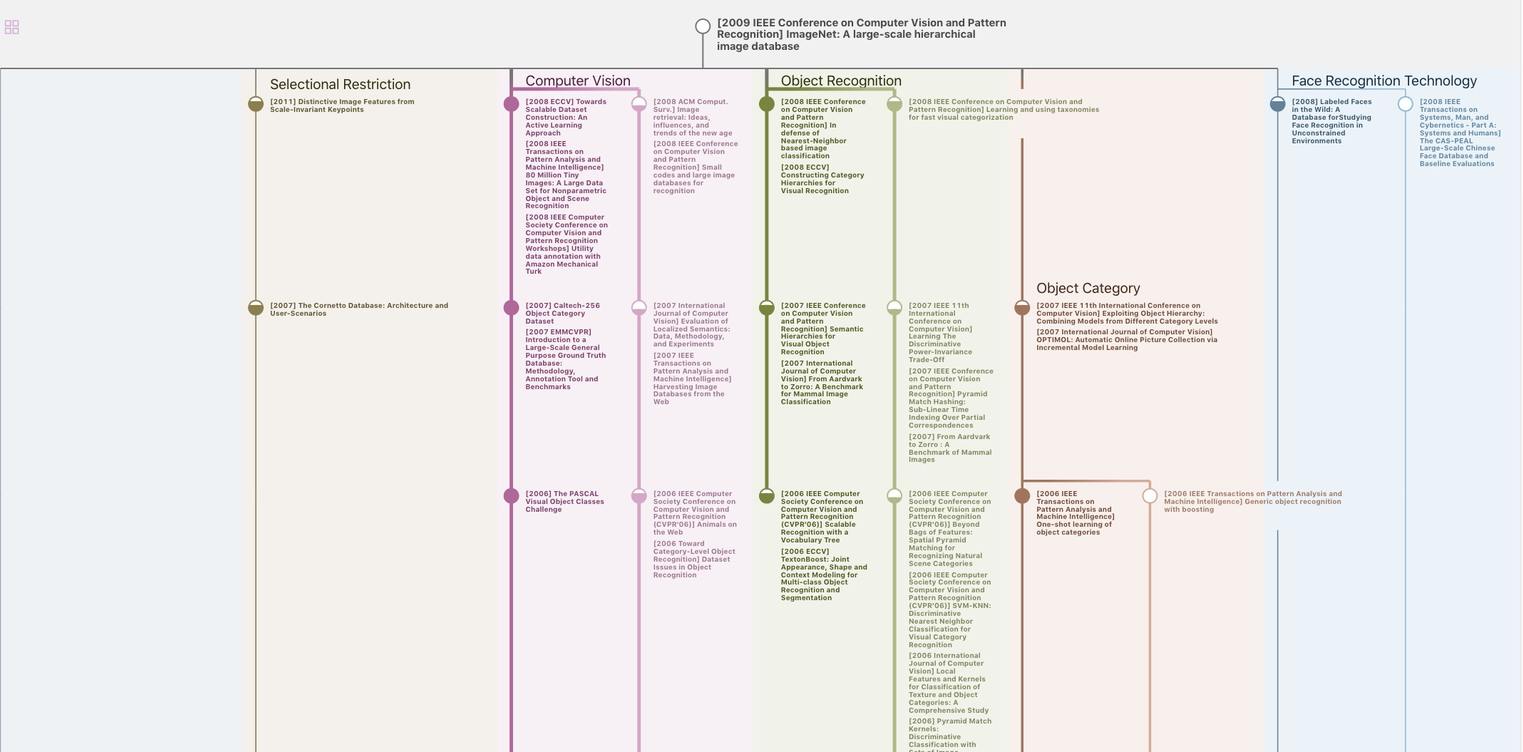
生成溯源树,研究论文发展脉络
Chat Paper
正在生成论文摘要