A Generative Model for Anomaly Detection in Time Series Data
Procedia Computer Science(2022)
摘要
Machine and deep learning models are receiving increasing attention in smart manufacturing to optimize processes or to identify anomalous behavior. To be able to compute time series data in generative networks is a challenging task and becomes more attractive to the present date, as there are a lot of use cases. Also, creating novel audio files or detecting failures in an industrial environment gains importance. In this paper, the generator of a conditional Generative Adversarial Network (GAN) is fed directly with high-frequency data. Its encoder-decoder structure is able to learn a representation of the signal. The code is kept sparse by an additional regularization net during training. Comparing the code of the input and the reconstructed signal allows the calculation of an anomaly score for each sample and to classify the input as normal or anomalous.
更多查看译文
关键词
Anomaly Detection,Time Series Data,Conditional GAN,Sparsity
AI 理解论文
溯源树
样例
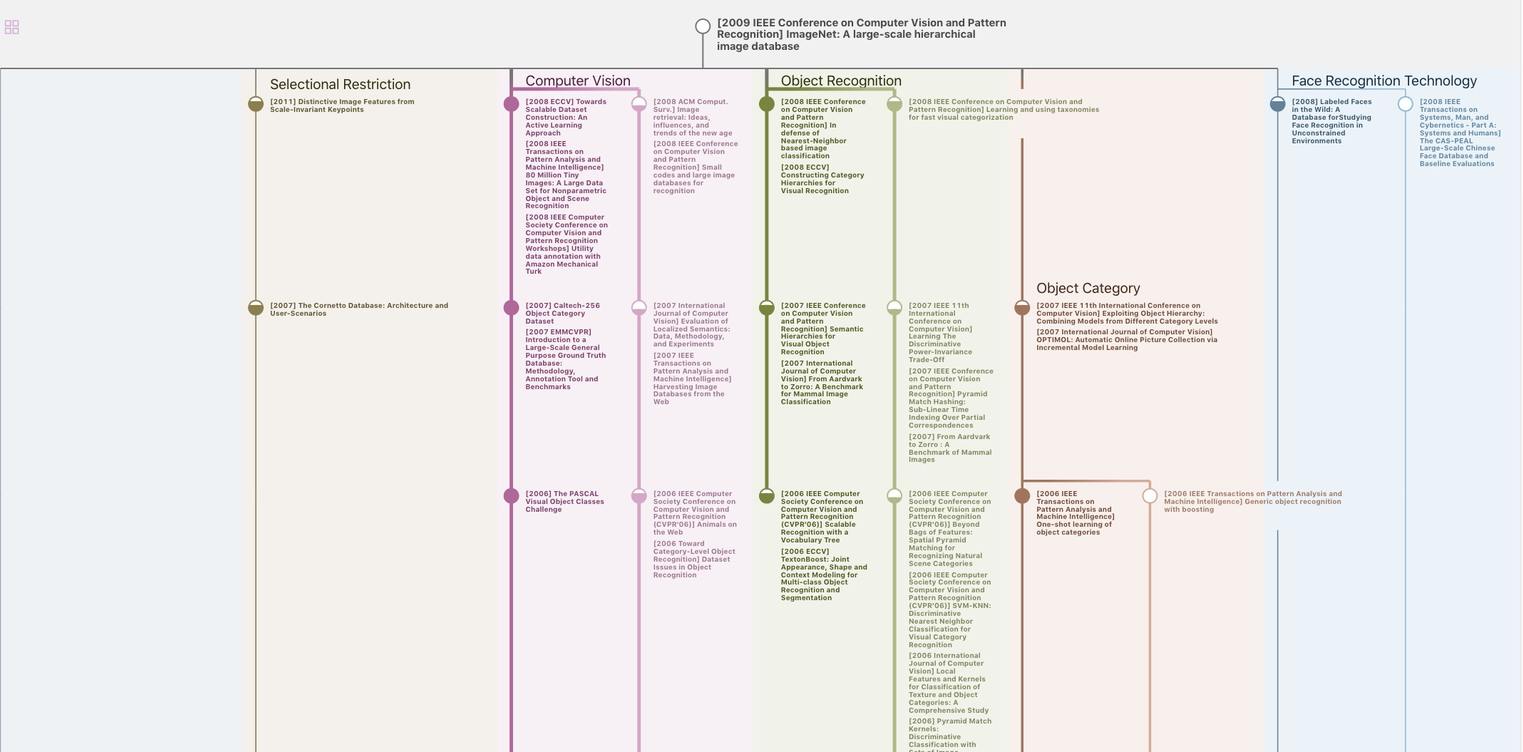
生成溯源树,研究论文发展脉络
Chat Paper
正在生成论文摘要