Generalized Input-Output Hidden-Markov-Models for Supervising Industrial Processes
3RD INTERNATIONAL CONFERENCE ON INDUSTRY 4.0 AND SMART MANUFACTURING(2022)
摘要
Often in industrial processes, batches of material are processed sequentially and repeatedly through a deterministic sequence of process steps. The possibly large number of sensor measurements collected throughout such industrial processes require supervisory modeling techniques that allow for characterizing the operating conditions (or states) of these process steps. This would allow for inference and predictions over the operating conditions of the current and upcoming process steps (e.g., abnormal behavior, failures, etc.) which may significantly assist the operators of the process. Traditionally, such inference questions in repeated processes can be handled in a rather straightforward manner via Input-Output Hidden Markov Models (IOHMM). However, standard IOHMM are limited to repeated and identical processes, while industrial processes may comprise multiple non-identical process steps and possibly with non-standard interdependencies between process steps. For this reason, in this paper we propose a generalization of standard IOHMM that is more appropriate for modeling industrial processes. Furthermore, we derive the update recursions for training such models and we show analytically that such update recursions guarantee an increase in the likelihood of the observation sequences. (C) 2022 The Authors. Published by Elsevier B.V.
更多查看译文
关键词
Input-Output Hidden Markov Models, Industrial processes, Diagnostics, Supervisory control, Inference
AI 理解论文
溯源树
样例
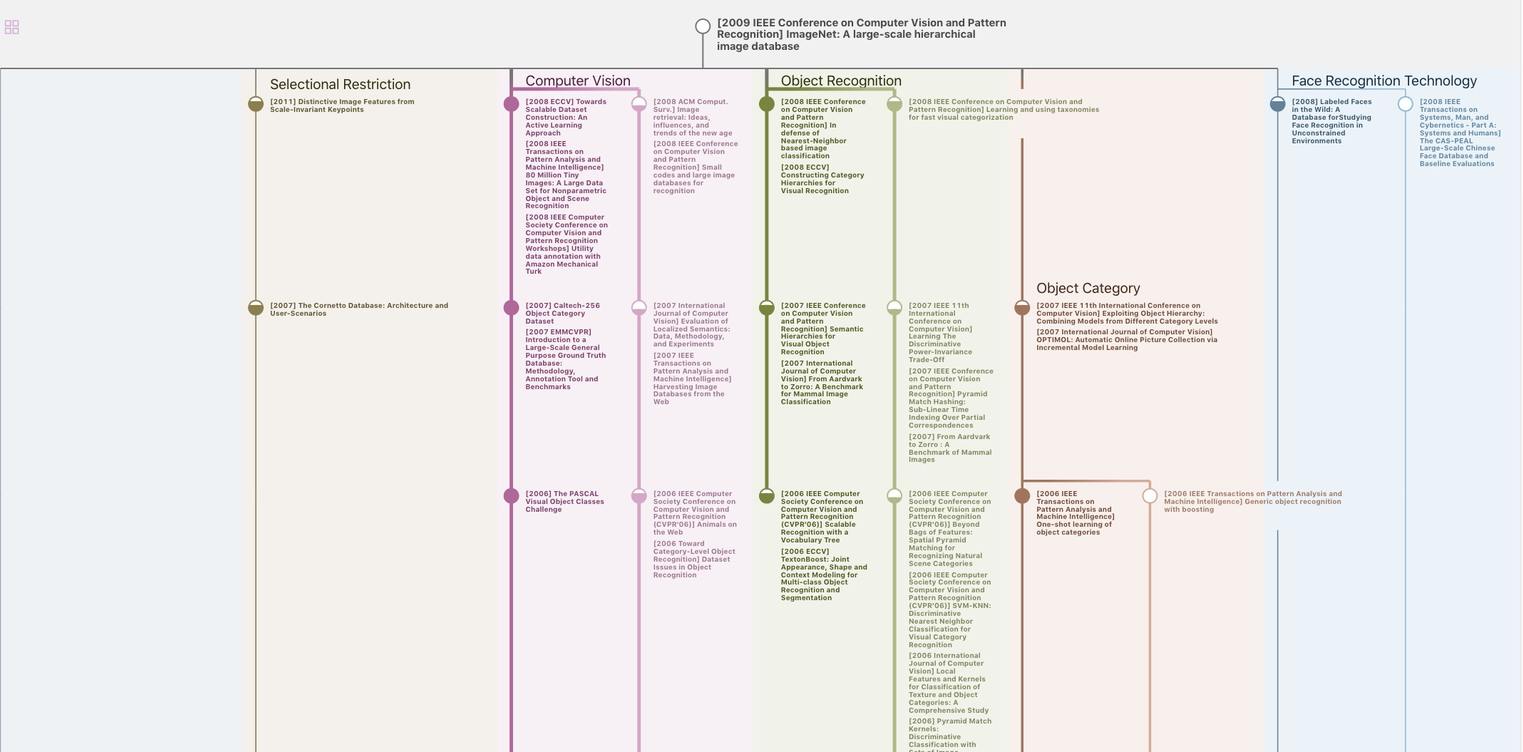
生成溯源树,研究论文发展脉络
Chat Paper
正在生成论文摘要