On an unsupervised method for parameter selection for the elastic net
MATHEMATICS IN ENGINEERING(2022)
摘要
Despite recent advances in regularization theory, the issue of parameter selection still remains a challenge for most applications. In a recent work the framework of statistical learning was used to approximate the optimal Tikhonov regularization parameter from noisy data. In this work, we improve their results and extend the analysis to the elastic net regularization. Furthermore, we design a data-driven, automated algorithm for the computation of an approximate regularization parameter. Our analysis combines statistical learning theory with insights from regularization theory. We compare our approach with state-of-the-art parameter selection criteria and show that it has superior accuracy.
更多查看译文
关键词
parameter selection, elastic net regularization, data-driven regularization, iterative thresholding, sub-gaussian vectors, matrix concentration inequalities
AI 理解论文
溯源树
样例
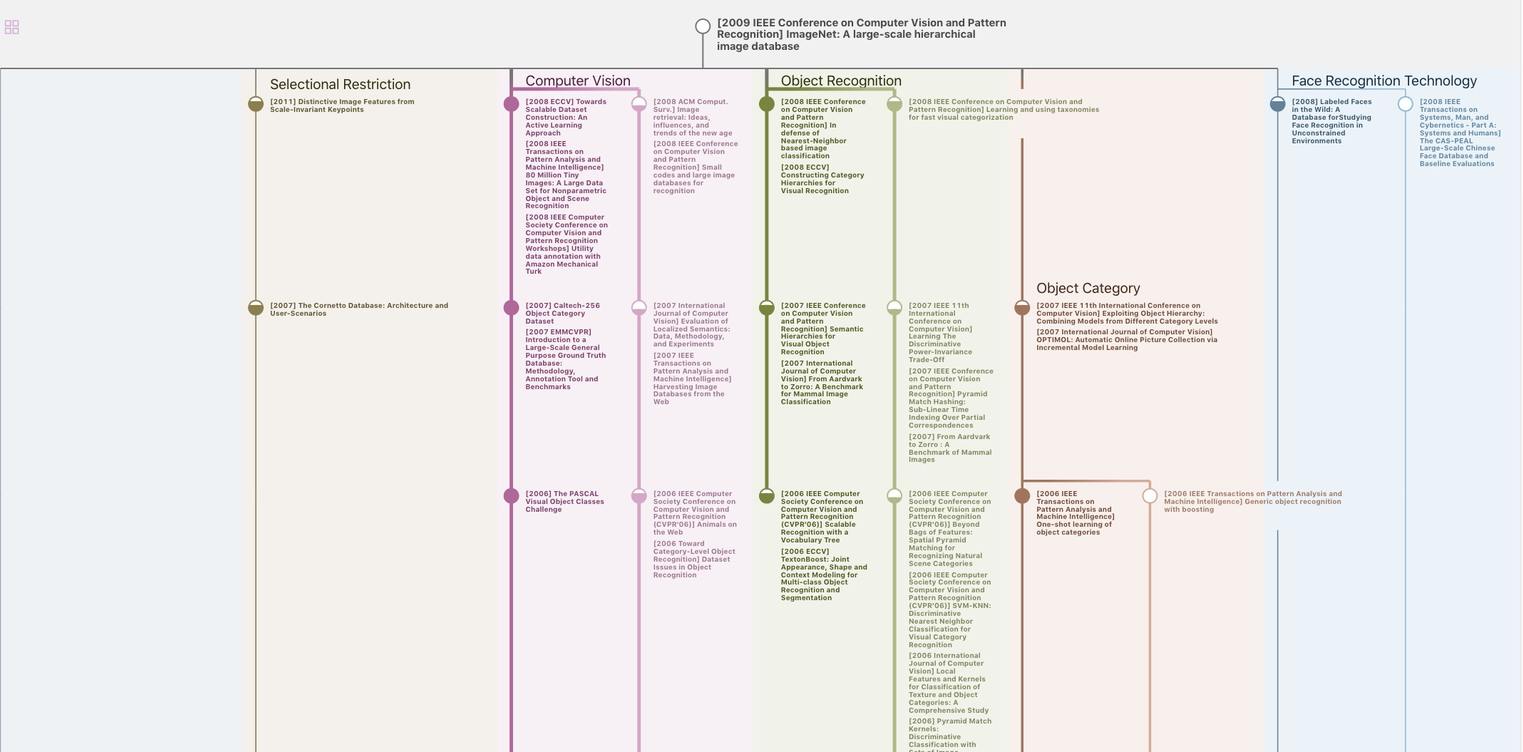
生成溯源树,研究论文发展脉络
Chat Paper
正在生成论文摘要