AI-Based Syntactic Complexity Metrics and Sight Interpreting Performance
INTELLIGENT HUMAN COMPUTER INTERACTION, IHCI 2021(2022)
摘要
Complex syntax may lead to increased cognitive effort during translation. However, it is unclear what kinds of syntactic complexity have a stronger impact on translation performance. In this paper, we employ several syntactic metrics which enable us to explore the impact of syntactic complexity on the quality in English-to-Chinese sight interpreting. We have operationalized syntactic complexity by six metrics, namely, Incomplete Dependency Theory metric (IDT), Dependency Locality Theory metric (DLT), Combined IDT and DLT metric (IDT+DLT), Left Embeddedness metric (LE), Nested Nouns Distancemetric (NND), and Bilingual Complexity Ratio metric (BRC). Three professional translators have manually annotated translation errors using MQM-derived error taxonomies, which includes accuracy, fluency, and style errors, each as critical or minor errors. We assessed inter-rater agreement by adopting weighted Fleiss' Kappa scores. We found that there are strong correlations between the IDT and IDT+DLT metrics and sight interpreting errors. We also found that language-specific syntactic differences between English and Chinese such as directions of branching and noun modifiers can have a strong influence on accuracy and critical errors.
更多查看译文
关键词
Language and speech interfaces, Cognitive interface design, AI and data, Syntactic complexity, Sight interpreting, Translation assessment
AI 理解论文
溯源树
样例
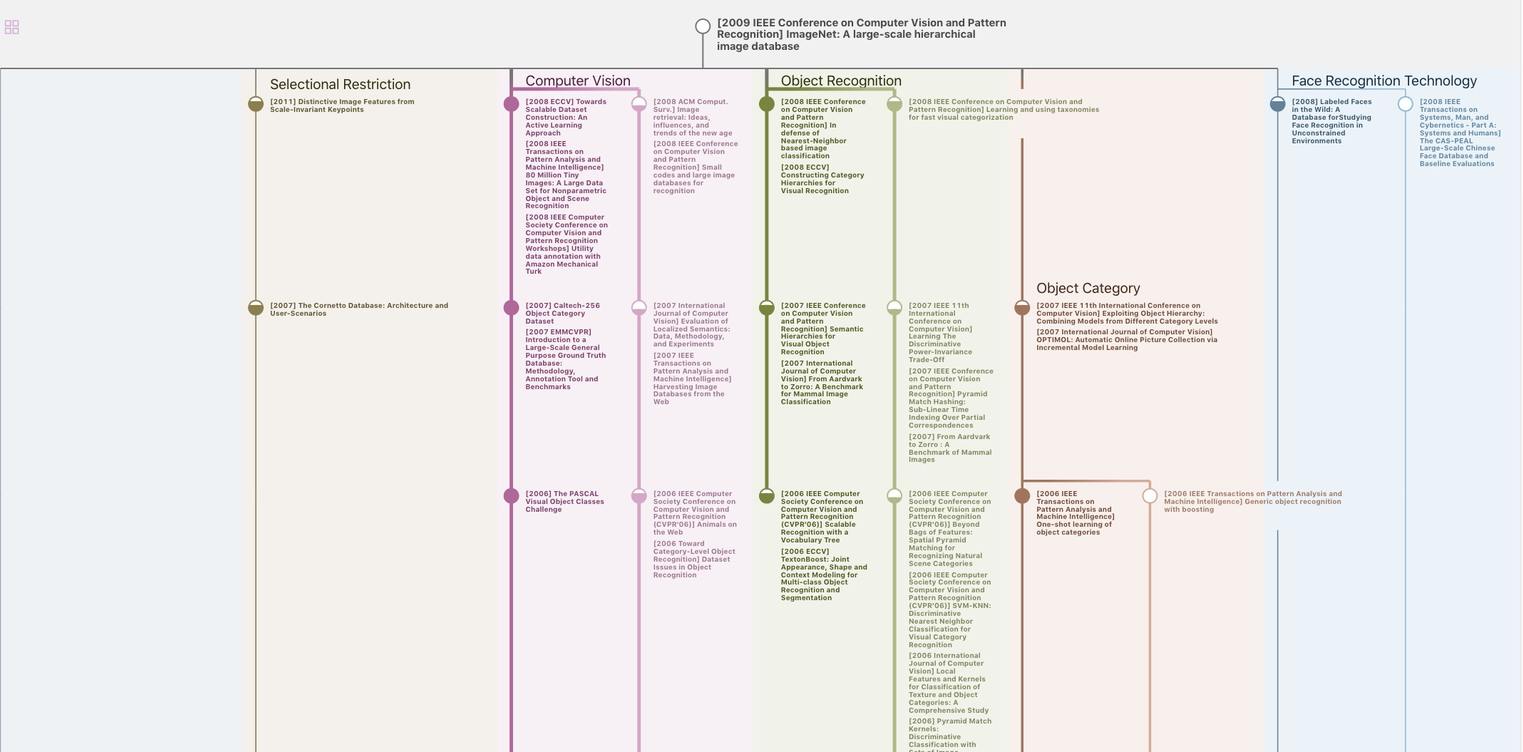
生成溯源树,研究论文发展脉络
Chat Paper
正在生成论文摘要