An Encoder-Decoder Deep Learning Framework for Building Footprints Extraction from Aerial Imagery
ARABIAN JOURNAL FOR SCIENCE AND ENGINEERING(2023)
Abstract
Building footprints segmentation in high-resolution satellite images has wide range of applications in disaster management, land cover analysis and urban planning. However, automatic extraction of building footprints offers many challenges due to large variations in building sizes, complex structures and cluttered background. Due to these challenges, current state-of-the-art methods are not efficient enough to completely extract buildings footprints and boundaries of different buildings. To this end, we propose an encoder-decoder framework that automatically extracts building footprints from satellite images. Specifically, the encoder part of the network uses a dense network that consists of dense convolutional and transition blocks to capture global multi-scale features. On the other hand, the decoder part of network uses sequence of deconvolution layers to recover the lost spatial information and obtains a dense segmentation map, where the white pixels represent buildings and black pixels represent background/other objects. In addition, we train the network in end-to-end fashion by using hybrid loss that enhances the performance of the framework. We use two publicly available benchmark datasets to gauge the performance of framework. From the experiments, we demonstrate that proposed method achieves comparatively best performance on challenging datasets.
MoreTranslated text
Key words
Building footprint extraction,Segmentation,Remote sensing
AI Read Science
Must-Reading Tree
Example
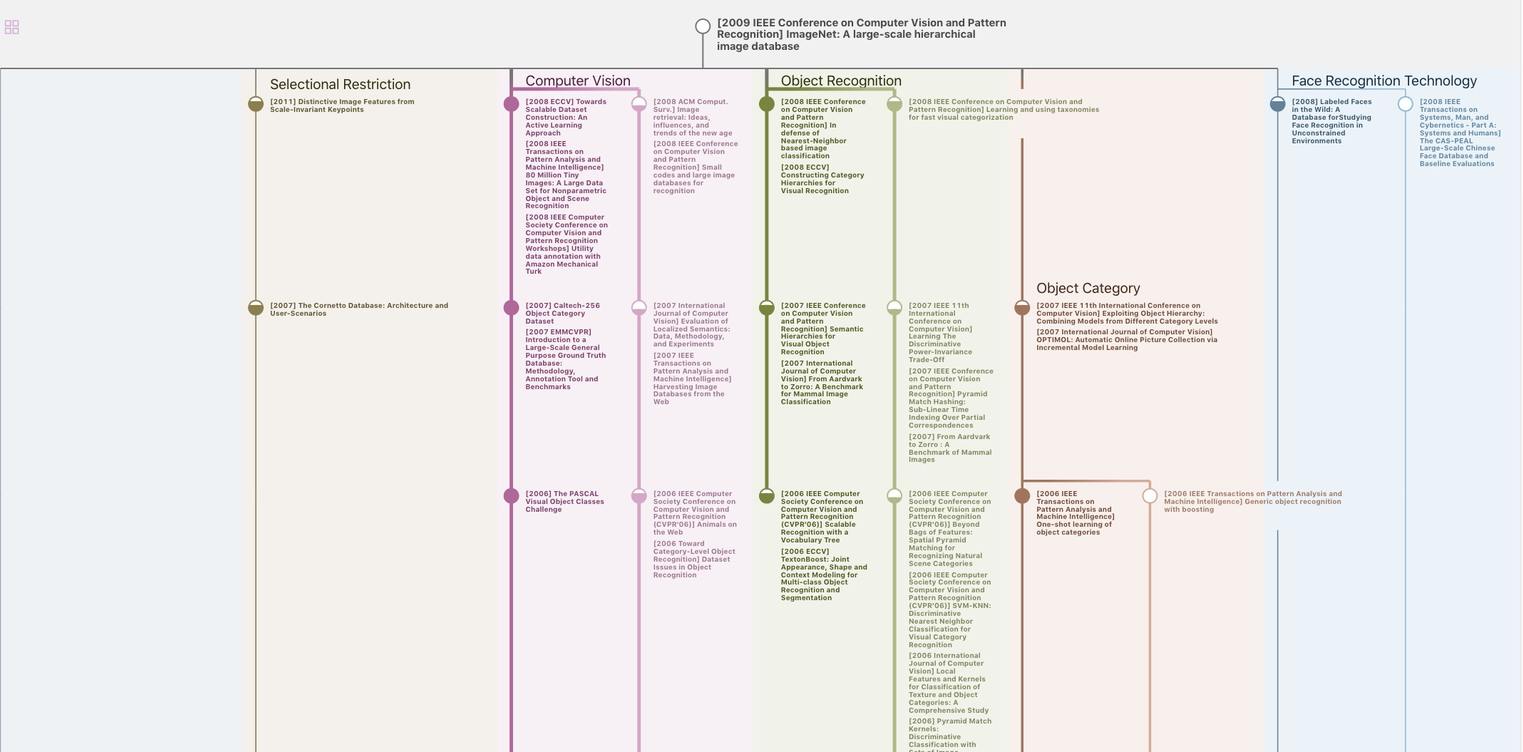
Generate MRT to find the research sequence of this paper
Chat Paper
Summary is being generated by the instructions you defined