Zero-offset data estimation using CNN for applying 1D full waveform inversion
JOURNAL OF GEOPHYSICS AND ENGINEERING(2022)
摘要
Full waveform inversion (FWI) in the time domain has limitations due to large computing time and memory requirements. Some studies have addressed this problem by using machine learning techniques. Most FWI studies using machine learning directly estimate the subsurface velocity structure by training the seismic data generated through various synthetic models to obtain the subsurface velocity structure. In this study, we propose a method to convert the common midpoint (CMP) gather to zero-offset data at a CMP location using a convolutional neural network (CNN) to increase the computing efficiency for the FWI. As the training data, we use synthetic data generated by the seismic exploration geometry and the source signature of field data. Since the proposed method performs FWI using the converted zero-offset data, it can be performed more efficiently than FWI using the existing multichannel data. However, it is difficult to apply a seismic exploration geometry and a source signature that has not been used for training. To verify the proposed method, it is applied to a synthetic model not used for learning as well as focused field data. It is confirmed that a proper subsurface velocity structure was obtained.
更多查看译文
关键词
full waveform inversion, zero-offset data, convolutional neural network, deep learning
AI 理解论文
溯源树
样例
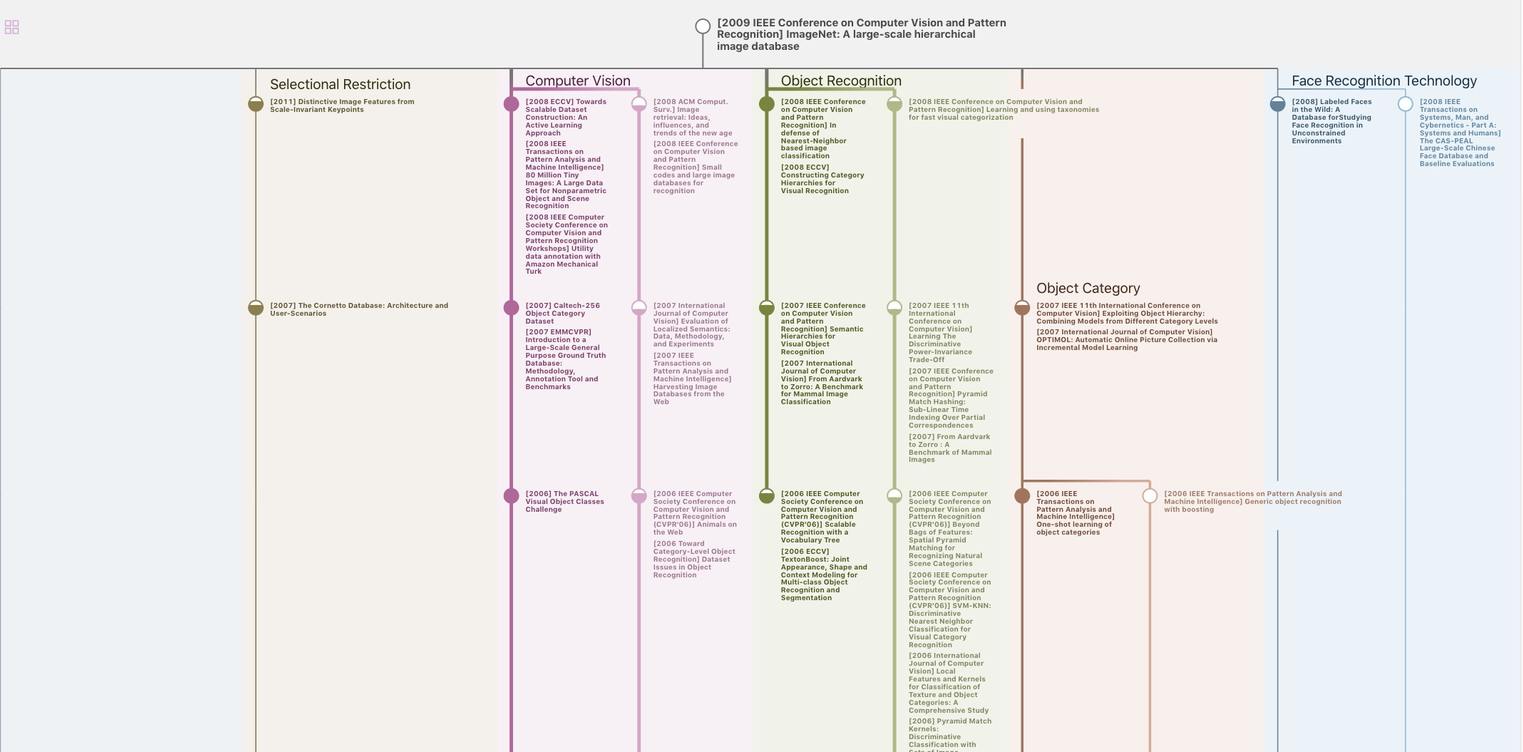
生成溯源树,研究论文发展脉络
Chat Paper
正在生成论文摘要