Validation of Deep Learning Segmentation of CT Images of Fiber-Reinforced Composites
JOURNAL OF COMPOSITES SCIENCE(2022)
摘要
Micro-computed tomography (mu CT) is a valuable tool for visualizing microstructures and damage in fiber-reinforced composites. However, the large sets of data generated by mu CT present a barrier to extracting quantitative information. Deep learning models have shown promise for overcoming this barrier by enabling automated segmentation of features of interest from the images. However, robust validation methods have not yet been used to quantify the success rate of the models and the ability to extract accurate measurements from the segmented image. In this paper, we evaluate the detection rate for segmenting fibers in low-contrast CT images using a deep learning model with three different approaches for defining the reference (ground-truth) image. The feasibility of measuring sub-pixel feature dimensions from the mu CT image, in certain cases where the mu CT image intensity is dependent on the feature dimensions, is assessed and calibrated using a higher-resolution image from a polished cross-section of the test specimen in the same location as the mu CT image.
更多查看译文
关键词
ceramic-matrix composites, ceramic fibers, structural characterization, mechanical testing
AI 理解论文
溯源树
样例
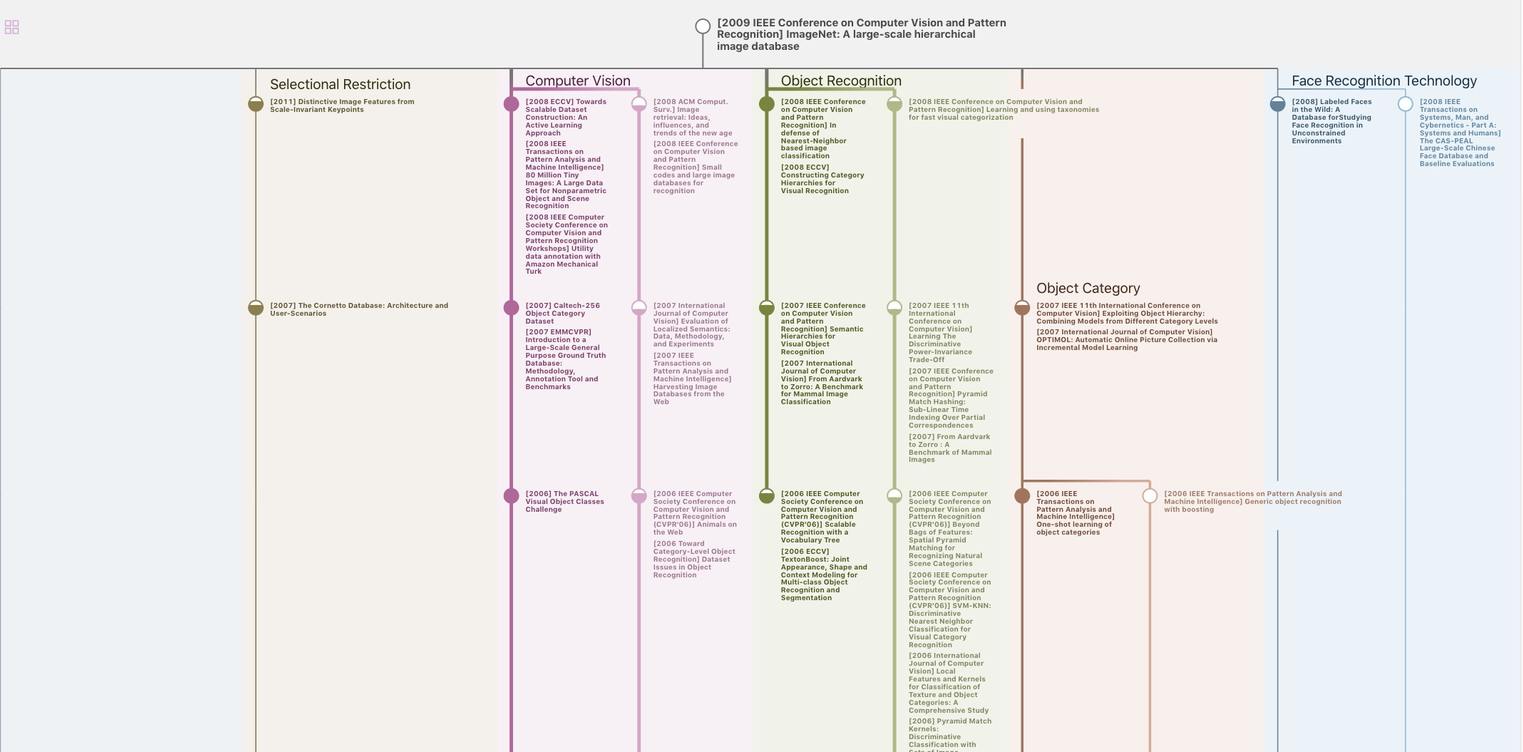
生成溯源树,研究论文发展脉络
Chat Paper
正在生成论文摘要