An Effective Tangent Stiffness of Train-Track-Bridge Systems Based on Artificial Neural Network
APPLIED SCIENCES-BASEL(2022)
摘要
Dynamic response analysis of a train-track-bridge (TTB) system is a challenging task for researchers and engineers, partially due to the complicated nature of the wheel-rail interaction (WRI). When Newton's method is used to solve implicit nonlinear finite element equations of a TTB system, consistent tangent stiffness (CTS) is essential to guarantee the quadratic convergence rate. However, the derivation and software implementation of CTS for the WRI element require significant efforts. Artificial neural network (ANN) can directly obtain a potentially good tangent stiffness by a trained relationship between input nodal displacement/velocity and output tangent stiffness. In this paper, the backpropagation neural-network-based tangent stiffness (BPTS) of the WRI element is derived and implemented into a general finite element software, OpenSees, and verified by dynamic response analysis of a high-speed train running on a seven span simply supported beam bridge. The accuracy and efficiency are compared between the use of BPTS and CTS. The results demonstrate that BPTS can not only save the significant efforts of deriving and software implementing CTS but also improve computational efficiency while ensuring good accuracy.
更多查看译文
关键词
wheel-rail interaction, BP neural network, tangent stiffness, train-track-bridge system
AI 理解论文
溯源树
样例
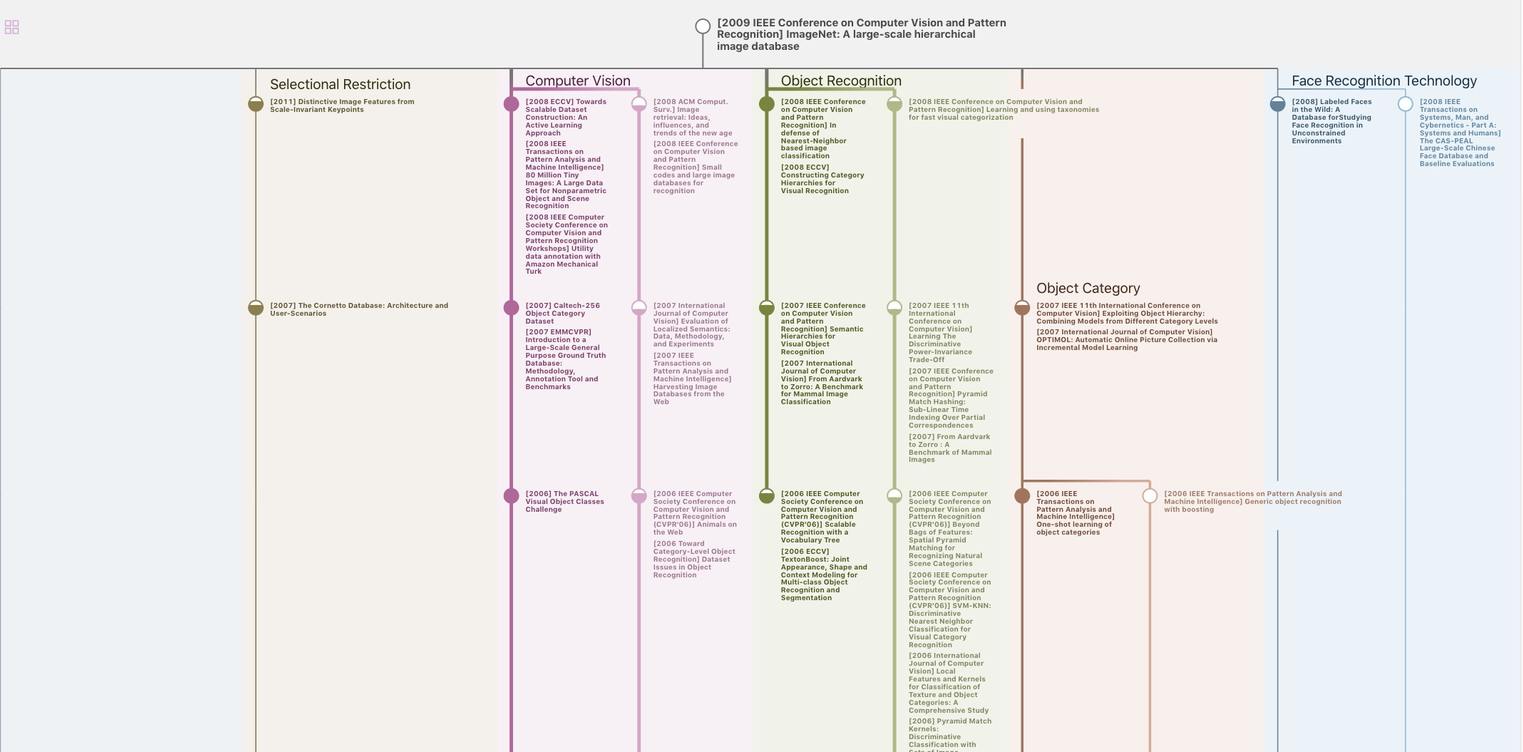
生成溯源树,研究论文发展脉络
Chat Paper
正在生成论文摘要