Reinforcement Learning Based Q-Routing: Performance Evaluation on Cognitive Radio Network Topologies
Wireless Personal Communications(2022)
摘要
The present work explores the scope of cognitive radio networks (CRNs) to support the spectrum demand on future diverse application specific wireless services supported by long-range multi-hop relay-based transmission. The essential requirement is dynamic route establishment where the traditional non-adaptive routing algorithms are not sufficient to support it in dynamic wireless networks. To address this issue, reinforcement learning (RL) based self-adaptable Q-routing in the CRN with different network topologies is explored with an objective to obtain the best performance in the secondary throughput. The traditional Q-routing policy offers the worst-case time-complexity of 𝒪(ed) to find the optimal route. The excessive time complexity for the route establishment reduces the actual secondary data transmission time, this also increases the power consumption during transmission. To reduce the time complexity of Q-routing, the proposed work invokes a strict upper bound on the network topology, which reduces the time complexity to 𝒪(N^2) and increases the secondary network throughput. The further study also reports that the complexity can be reduced to 𝒪(N) if the distributed relays follow a tree topology in the network configuration. A large set of simulation results show that the tree structure-based Q-routing in CR enhances the network throughput by ∼ 6.83% , ∼ 7.21% over the other network topologies.
更多查看译文
关键词
Cognitive radio networks,Underlay mode,Multi-hop relay network,Reinforcement learning,Q-routing.
AI 理解论文
溯源树
样例
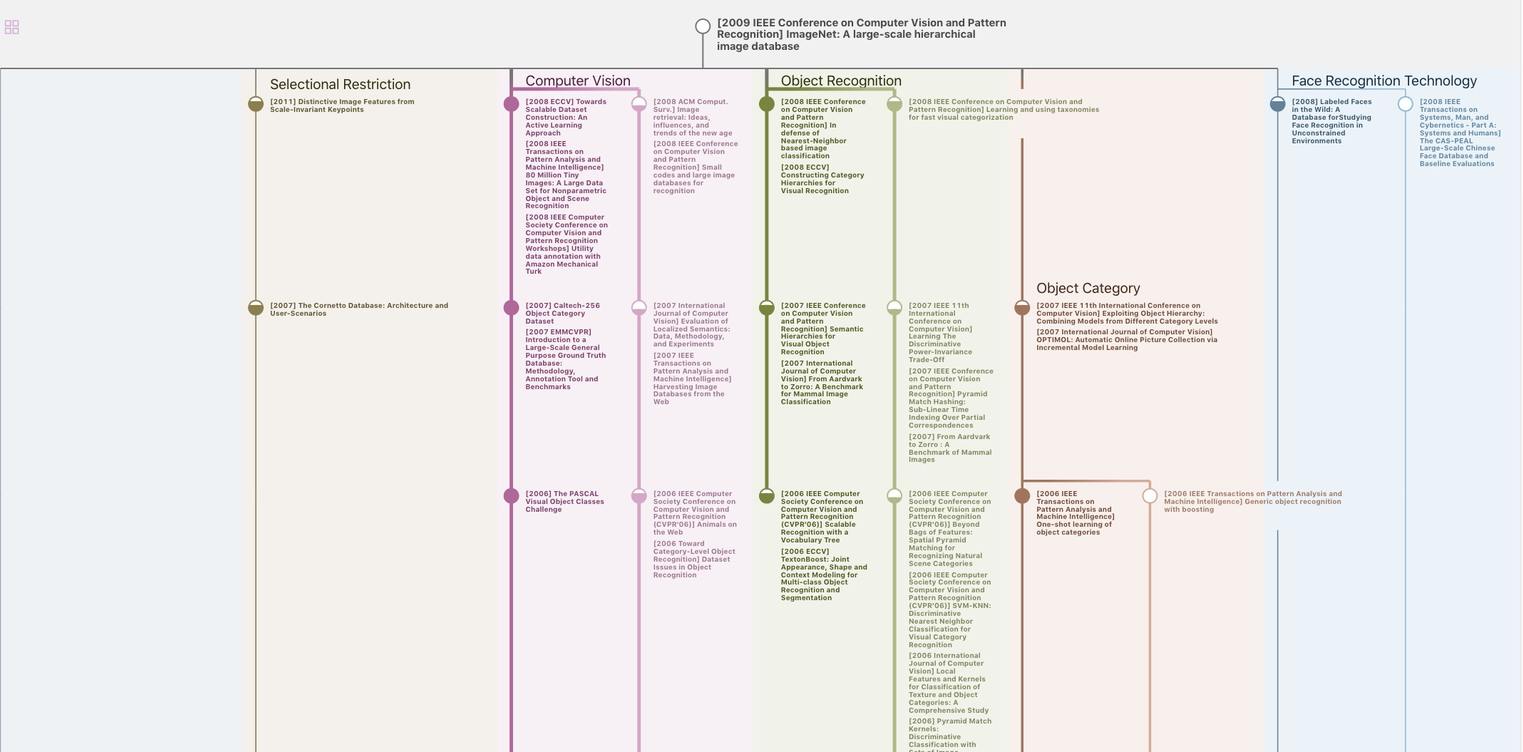
生成溯源树,研究论文发展脉络
Chat Paper
正在生成论文摘要