Unsupervised Segmentation in NSCLC: How to Map the Output of Unsupervised Segmentation to Meaningful Histological Labels by Linear Combination?
APPLIED SCIENCES-BASEL(2022)
摘要
Background: Segmentation is, in many Pathomics projects, an initial step. Usually, in supervised settings, well-annotated and large datasets are required. Regarding the rarity of such datasets, unsupervised learning concepts appear to be a potential solution. Against this background, we tested for a small dataset on lung cancer tissue microarrays (TMA) if a model (i) first can be in a previously published unsupervised setting and (ii) secondly can be modified and retrained to produce meaningful labels, and (iii) we finally compared this approach to standard segmentation models. Methods: (ad i) First, a convolutional neuronal network (CNN) segmentation model is trained in an unsupervised fashion, as recently described by Kanezaki et al. (ad ii) Second, the model is modified by adding a remapping block and is retrained on an annotated dataset in a supervised setting. (ad iii) Third, the segmentation results are compared to standard segmentation models trained on the same dataset. Results: (ad i-ii) By adding an additional mapping-block layer and by retraining, models previously trained in an unsupervised manner can produce meaningful labels. (ad iii) The segmentation quality is inferior to standard segmentation models trained on the same dataset. Conclusions: Unsupervised training in combination with subsequent supervised training offers for histological images here no benefit.
更多查看译文
关键词
histopathology, lung cancer, supervised segmentation, unsupervised segmentation
AI 理解论文
溯源树
样例
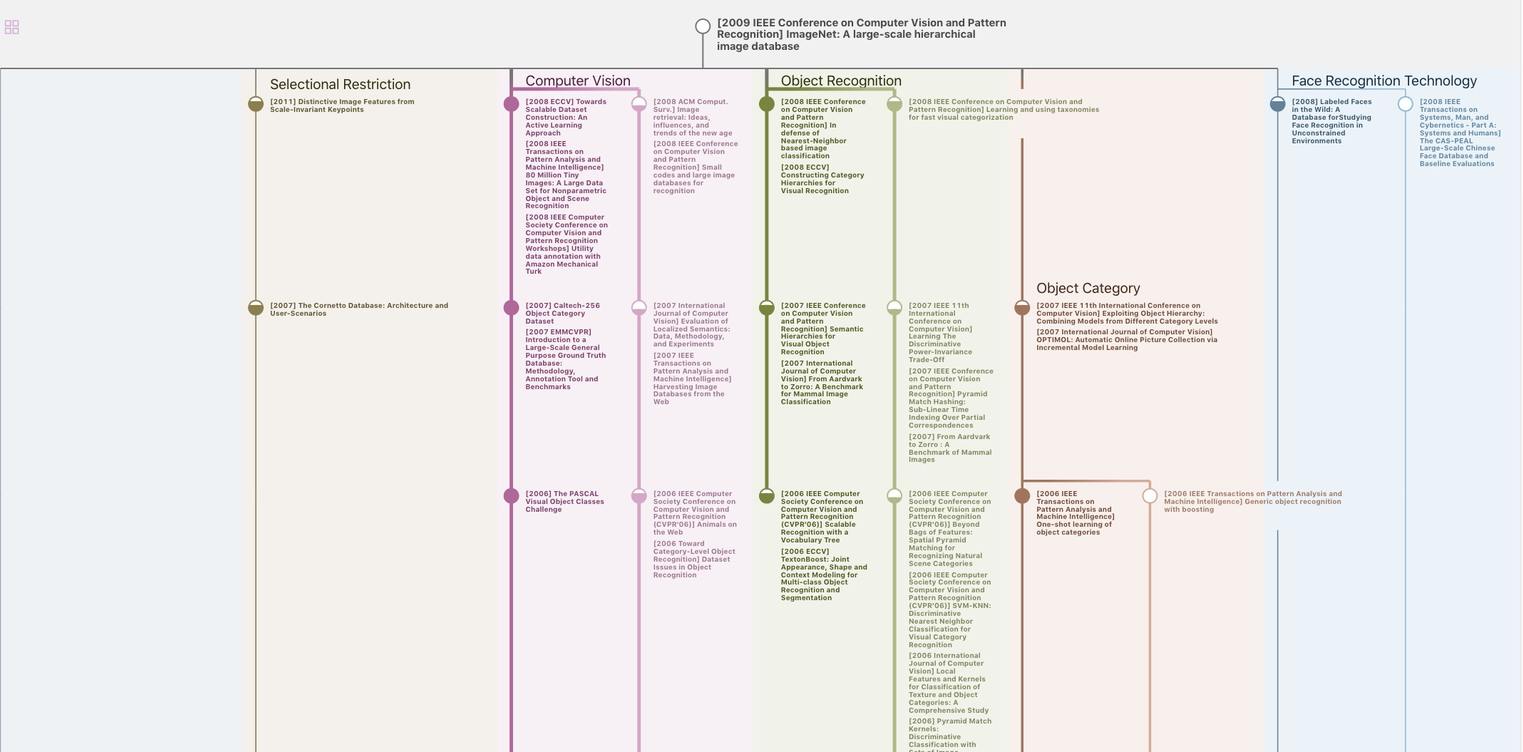
生成溯源树,研究论文发展脉络
Chat Paper
正在生成论文摘要