Monitoring of the refractory lining in a shielded electric arc furnace: An online multitarget regression trees approach
STRUCTURAL CONTROL & HEALTH MONITORING(2022)
摘要
Being able to predict future temperatures on the wall lining is key when controlling and scheduling maintenance for large industrial smelting furnaces. In this paper, we propose and test a machine learning approach for predicting lining temperatures in a ferronickel smelting furnace. This approach was deployed and evaluated in a real-world scenario, i.e., in one of Cerro Matoso S.A.'s (CMSA) industrial plant furnaces. Different techniques were tested, and finally, a multitarget regression (MTR) model showed the best performance. Previous state of the art focused on predicting only one target sensor; in contrast, our model is capable of predicting up to 12 targets. Two MTR models were tested: the incremental structured output prediction tree (iSOUP-Tree) and the stacked single-target Hoeffding tree regressor (SST-HT). The SST-HT method had the best behavior in terms of the average mean absolute error (AMAE) and average root mean square error (ARMSE). The results indicate that the developed MTR models can accurately predict the measured temperature on multiple point sensors. Results of this work are expected to help the process of structural control and health monitoring of the furnace linings located at CMSA's plant.
更多查看译文
关键词
HoeffdingTree, machine learning, multitarget regression, random forest, refractory lining temperature, SHM, smelting furnace, temperature prediction
AI 理解论文
溯源树
样例
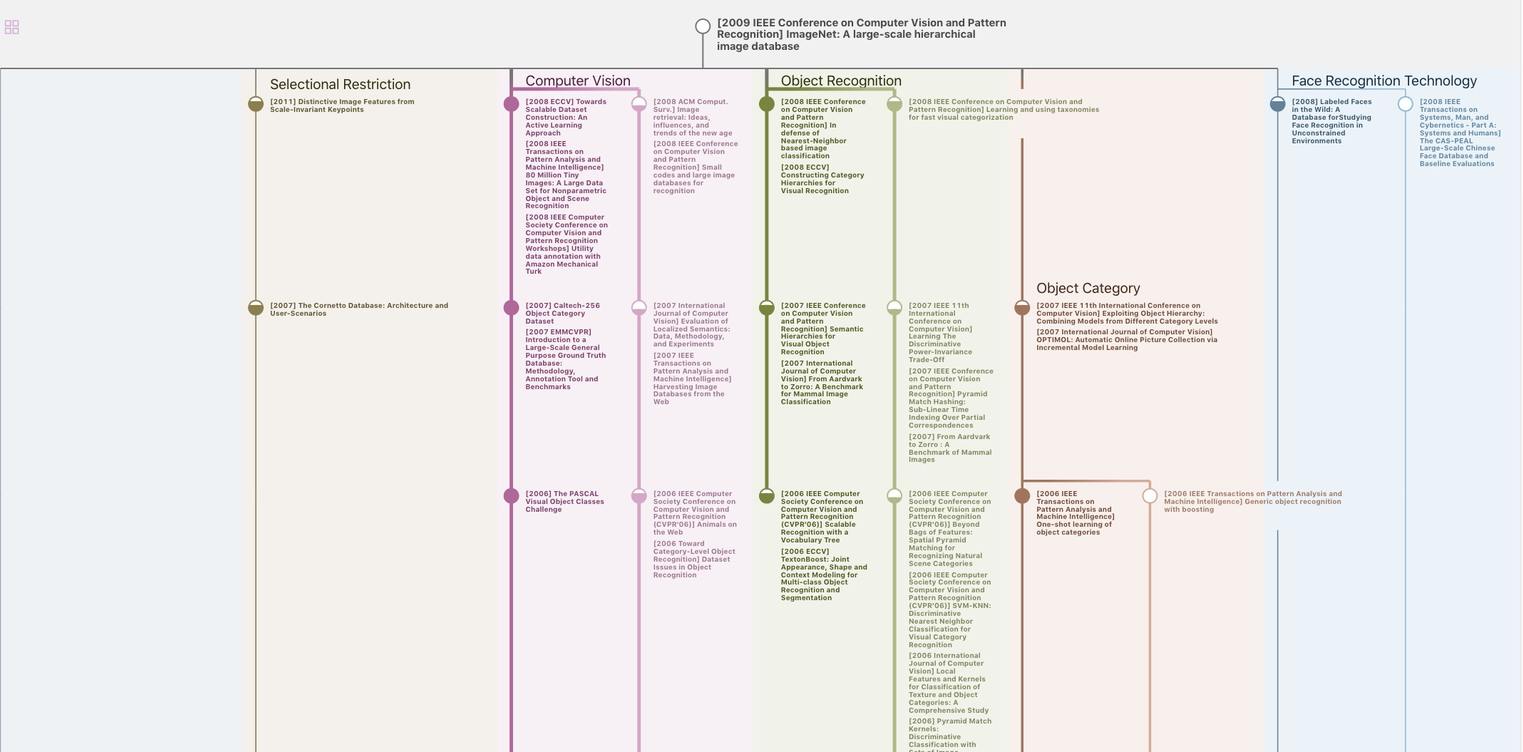
生成溯源树,研究论文发展脉络
Chat Paper
正在生成论文摘要