Providing direction for mechanistic inferences in radical cascade cyclization using a Transformer model
Organic Chemistry Frontiers(2022)
摘要
Even in modern organic chemistry, predicting or proposing a reaction mechanism and speculating on reaction intermediates remains challenging. For example, it is challenging to predict the regioselectivity of radical addition in radical cascade cyclization, which finds wide application in life sciences and pharmaceutical industries. In this work, radical cascade cyclization is considered to demonstrate that Transformer, a sequence-to-sequence deep learning model, is capable of predicting the reaction intermediates. A major challenge is that the number of intermediates involved in the different reactions is variable. By defining “key intermediates”, this thorny problem was avoided. We curated a database of 874 chemical equations and corresponding 1748 key intermediates and used the dataset to fine-tune a model pretrained based on the USPTO dataset. The format of the dataset is very different between pretraining and fine-tuning. Correspondingly, the resulting Transformer model achieves remarkable accuracy in predicting the structures and stereochemistry of the key intermediates. The interpretability produced by attention weights of the resulting Transformer model shows a mindset similar to that of an experienced chemist. Hence, our study provides a novel approach to help chemists discover the mechanisms of organic reactions.
更多查看译文
关键词
Computational Chemistry
AI 理解论文
溯源树
样例
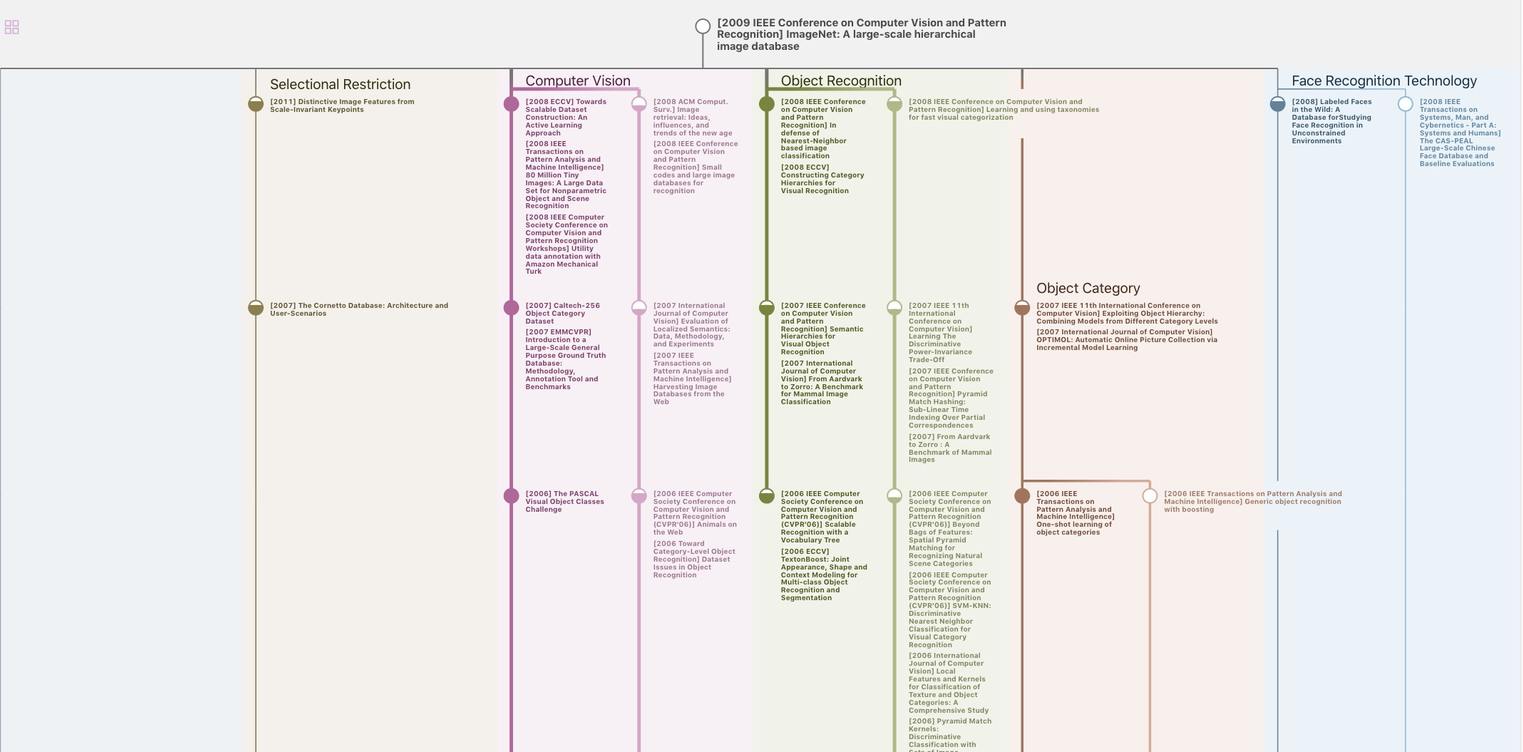
生成溯源树,研究论文发展脉络
Chat Paper
正在生成论文摘要