Performance Comparison of Training Datasets for System Call-Based Malware Detection with Thread Information
IEICE TRANSACTIONS ON INFORMATION AND SYSTEMS(2021)
摘要
The number of malware, including variants and new types, is dramatically increasing over the years, posing one of the greatest cyber-security threats nowadays. To counteract such security threats, it is crucial to detect malware accurately and early enough. The recent advances in machine learning technology have brought increasing interest in malware detection. A number of research studies have been conducted in the field. It is well known that malware detection accuracy largely depends on the training dataset used. Creating a suitable training dataset for efficient malware detection is thus crucial. Different works usually use their own dataset; therefore, a dataset is only effective for one detection method, and strictly comparing several methods using a common training dataset is difficult. In this paper, we focus on how to create a training dataset for efficiently detecting malware. To achieve our goal, the first step is to clarify the information that can accurately characterize malware. This paper concentrates on threads, by treating them as important information for characterizing malware. Specifically, on the basis of the dynamic analysis log from the Alkanet, a system call tracer, we obtain the thread information and classify the thread information processing into four patterns. Then the malware detection is performed using the number of transitions of system calls appearing in the thread as a feature. Our comparative experimental results showed that the primary thread information is important and useful for detecting malware with high accuracy.
更多查看译文
关键词
malware detection, machine learning, system calls, thread
AI 理解论文
溯源树
样例
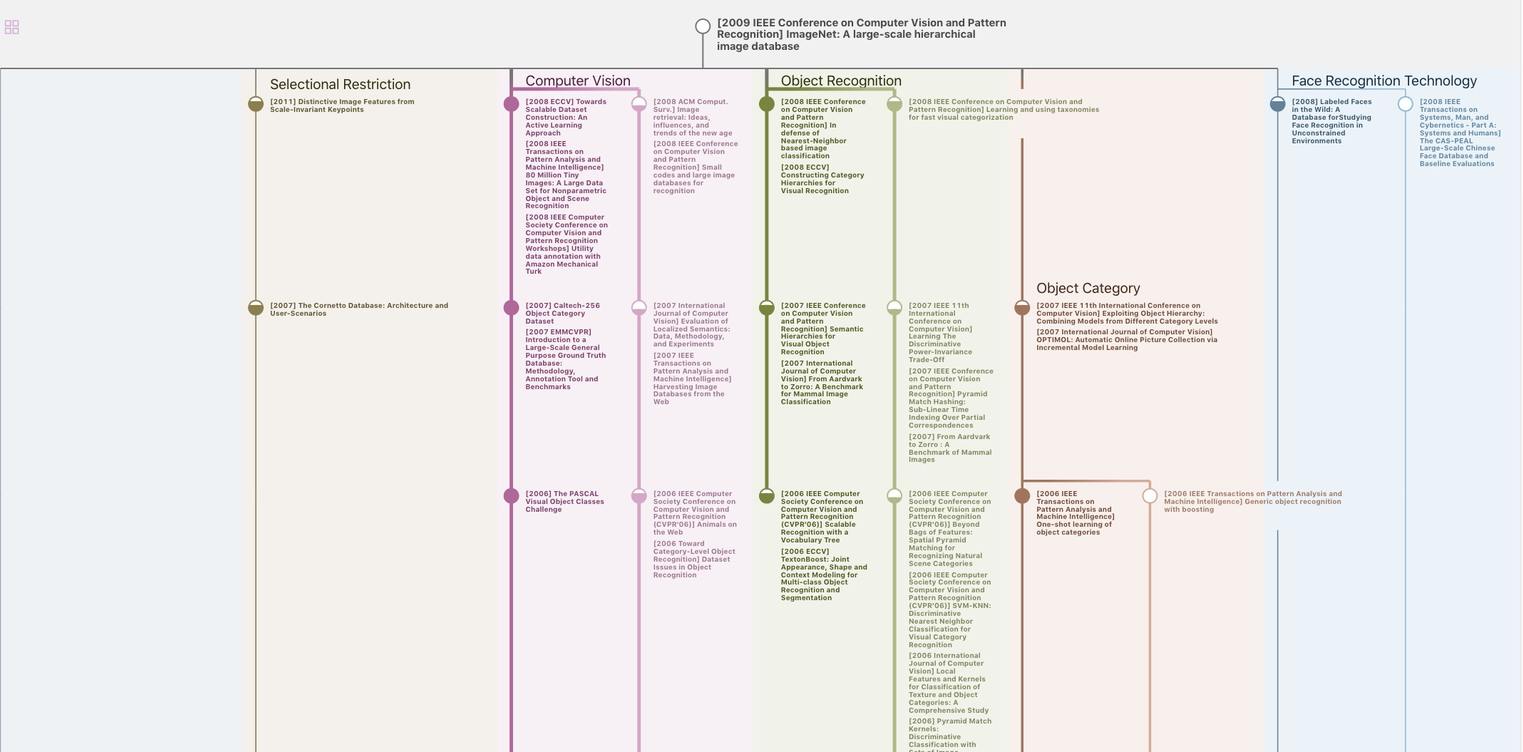
生成溯源树,研究论文发展脉络
Chat Paper
正在生成论文摘要