Autonomous Penetration Testing Based on Improved Deep Q-Network
APPLIED SCIENCES-BASEL(2021)
摘要
Penetration testing is an effective way to test and evaluate cybersecurity by simulating a cyberattack. However, the traditional methods deeply rely on domain expert knowledge, which requires prohibitive labor and time costs. Autonomous penetration testing is a more efficient and intelligent way to solve this problem. In this paper, we model penetration testing as a Markov decision process problem and use reinforcement learning technology for autonomous penetration testing in large scale networks. We propose an improved deep Q-network (DQN) named NDSPI-DQN to address the sparse reward problem and large action space problem in large-scale scenarios. First, we reasonably integrate five extensions to DQN, including noisy nets, soft Q-learning, dueling architectures, prioritized experience replay, and intrinsic curiosity model to improve the exploration efficiency. Second, we decouple the action and split the estimators of the neural network to calculate two elements of action separately, so as to decrease the action space. Finally, the performance of algorithms is investigated in a range of scenarios. The experiment results demonstrate that our methods have better convergence and scaling performance.
更多查看译文
关键词
penetration testing, reinforcement learning, cybersecurity, DQN algorithm
AI 理解论文
溯源树
样例
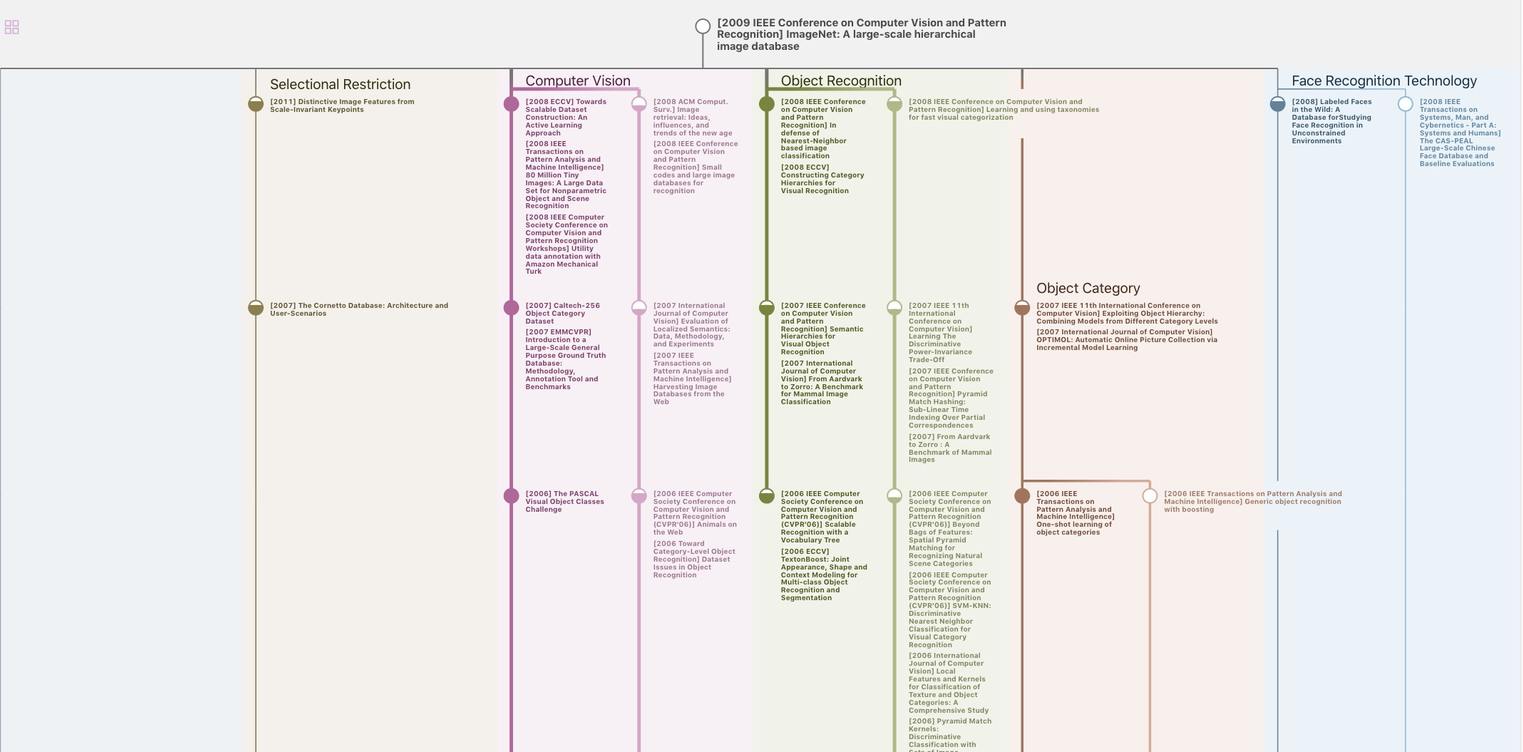
生成溯源树,研究论文发展脉络
Chat Paper
正在生成论文摘要