Towards Addressing Noise and Static Variations of Analog Computations Using Efficient Retraining
MACHINE LEARNING AND PRINCIPLES AND PRACTICE OF KNOWLEDGE DISCOVERY IN DATABASES, ECML PKDD 2021, PT I(2021)
摘要
One of the most promising technologies to solve the energy efficiency problem for artificial neural networks on embedded systems is analog computing, which, however, is fraught with noise due to summations of unwanted or disturbing energy, and static variations related to manufacturing. While these inaccuracies can have a negative effect on the accuracy, in particular for naively deployed networks, the robustness of the networks can be significantly enhanced by a retraining procedure that considers the particular hardware instance. However, this hardware-in-the-loop retraining is very slow and thus often the bottleneck hindering the development of larger networks. Furthermore, it is hardware-instance-specific and requires access to the instance in question. Therefore, we propose a representation of a hardware instance in software, based on simple, parallelization-friendly software structures, which could replace the hardware for the major fraction of retraining. The representation is based on lookup tables, splines as interpolated functions and additive Gaussian noise to cover static variations together with electrical noise of the multiplier array and column-wise integrators. The combined approach using the proposed representation together with some final epochs of hardware-in-the-loop retraining reduces the overall training time from over 10 h to less than 2 h compared to a full hardware-inthe-loop retraining, while notably increasing accuracy. This work highlights that including device-specific static variations and noise in the training process is essential for a time-efficient hardware-aware network training for analog computations, and that major parts can be extracted from the hardware instance and represented with simple and efficient software structures. This work is the first step towards hardware-specific but hardware-inaccessible training, addressing speed and accuracy.
更多查看译文
关键词
Analog hardware representation, Hardware-aware training, Static variations, Electrical noise, Analog computations
AI 理解论文
溯源树
样例
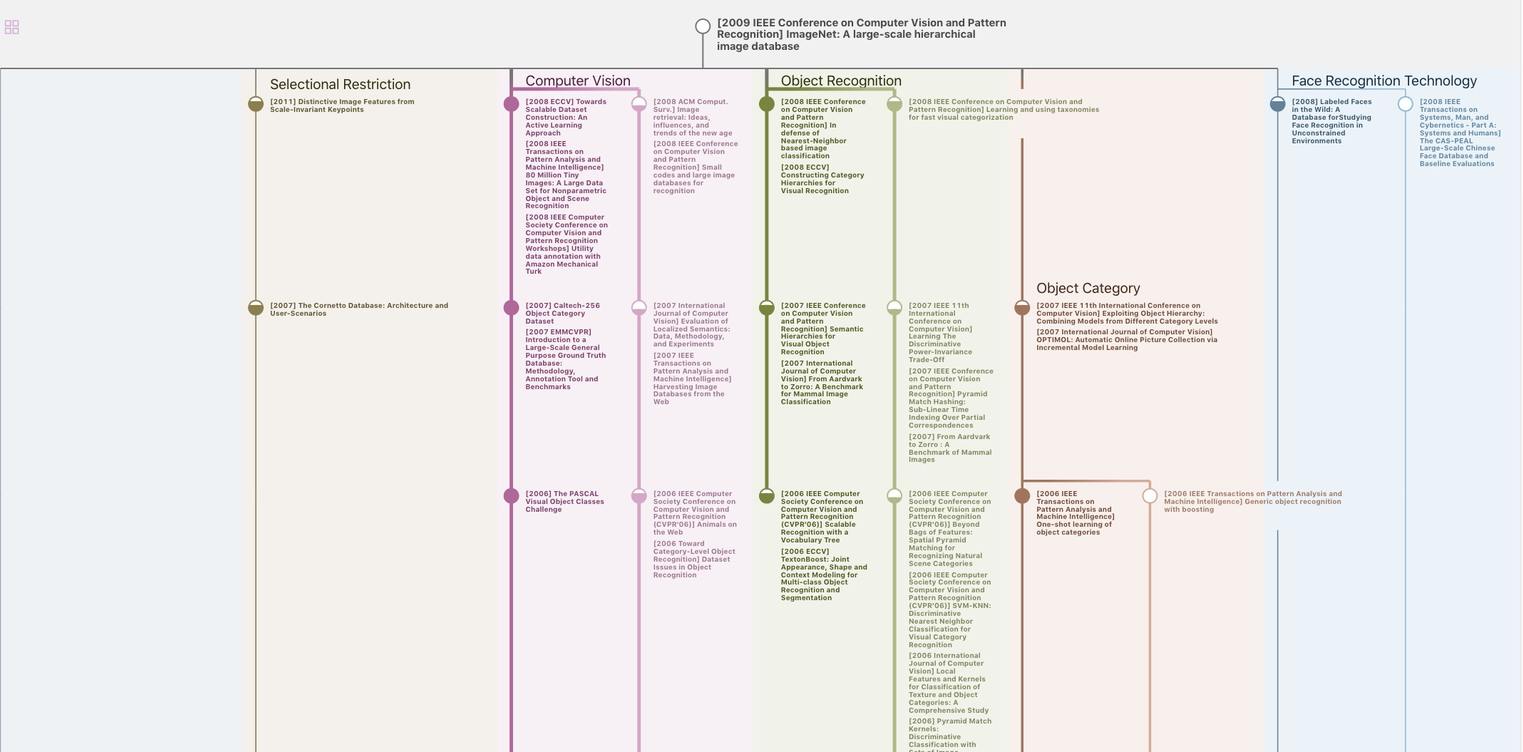
生成溯源树,研究论文发展脉络
Chat Paper
正在生成论文摘要