Graph Homomorphism Features: Why Not Sample?
MACHINE LEARNING AND PRINCIPLES AND PRACTICE OF KNOWLEDGE DISCOVERY IN DATABASES, ECML PKDD 2021, PT I(2021)
摘要
Recent research in the domain of computed graph embeddings has shown that graph homomorphism numbers constitute expressive features that are well-suited for machine learning tasks such as graph classification. In this work-in-progress paper, we attempt to make this methodology scalable by obtaining additive approximations to graph homomorphism densities via a simple sampling algorithm. We show in experiments that these approximate homomorphism densities perform as well as homomorphism numbers on standard graph classification datasets. Moreover, we show that, unlike algorithms that compute homomorphism numbers, our sampling algorithm is highly scalable to larger graphs.
更多查看译文
关键词
Graph embedding, Graph homomorphism, Graph classification
AI 理解论文
溯源树
样例
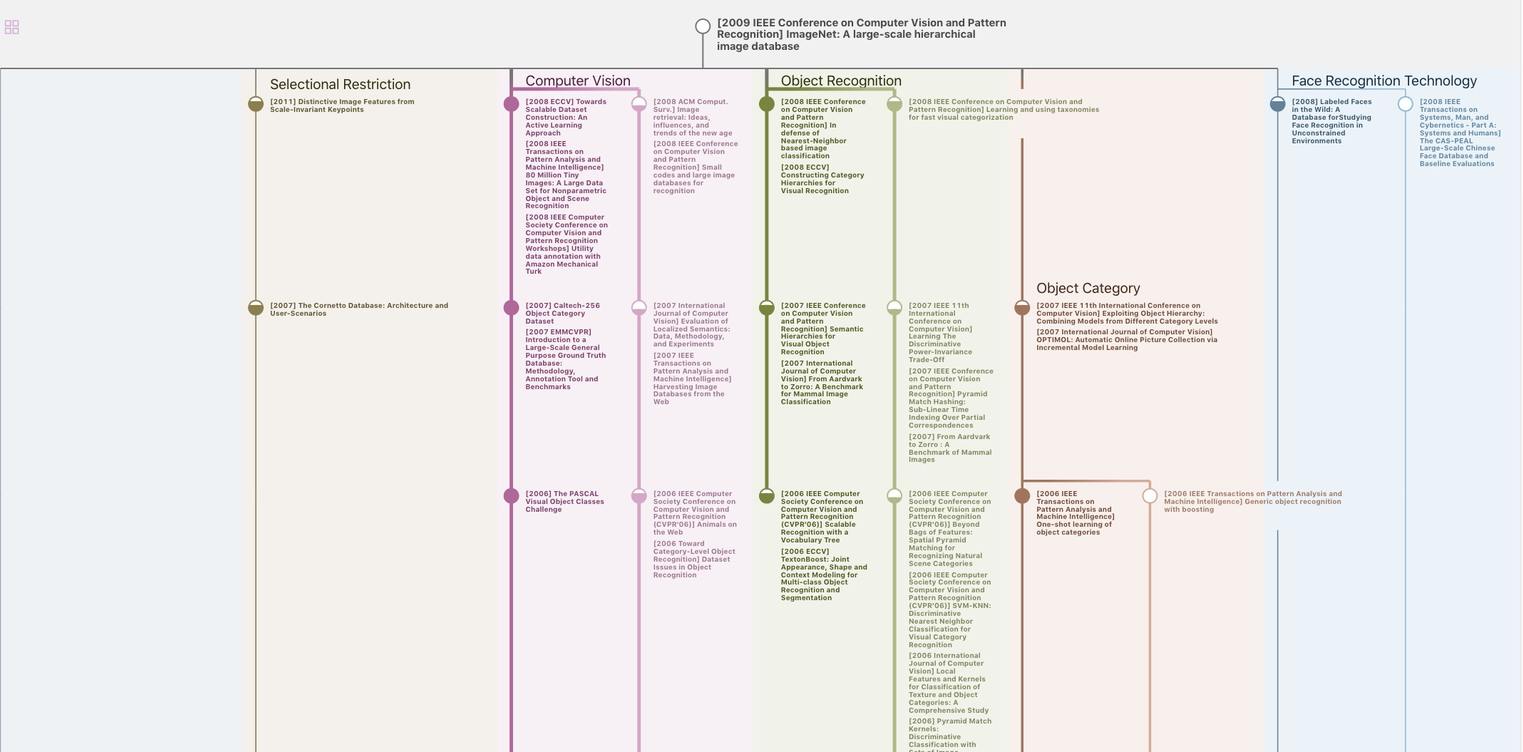
生成溯源树,研究论文发展脉络
Chat Paper
正在生成论文摘要