Point Cloud Registration Based on Multi-Scale Feature and Point Distance Constraint
LASER & OPTOELECTRONICS PROGRESS(2021)
摘要
The existing matching algorithm has a low registration accuracy of point clouds with low overlap, and is more sensitive to different scales. In order to achieve a better registration effect, it is necessary to preprocess or adjust more parameters to the point cloud. Fast point feature histogram (FPFH) has a low complexity, and retains most of the features of the point cloud. Therefore, we propose an improved registration algorithm based on FPFH of the point cloud. First, extracting key points with multi-scale features based on FPFH to adapt to different scales point cloud datasets, while reducing the number of parameters that require adjustment. Then, the corresponding point relationship after the initial screening of FPFH matching is accurately extracted, the distance constraint condition in the point cloud is added, which reduces sensitivity of the algorithm to overlap and the preliminary transformation matrix of registration is obtained. Finally, the iterative closest point algorithm is subjected to finetuning to achieve the purpose of accurate registration. The experimental results show that the algorithm has good registration accuracy on different overlap and different scales of point cloud datasets.
更多查看译文
关键词
machine vision, point cloud registration, fast point feature histogram, iterative closest point, Euclidean distance
AI 理解论文
溯源树
样例
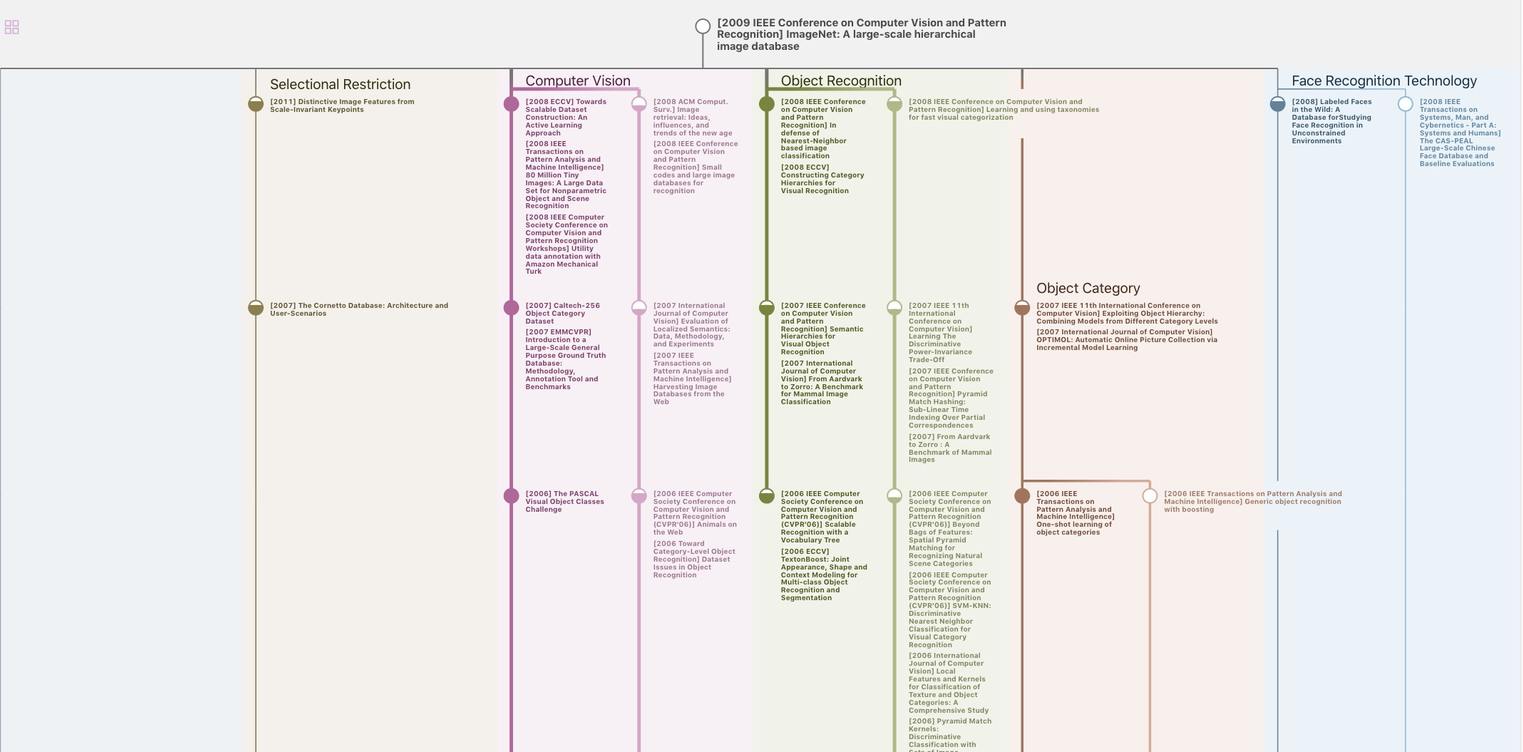
生成溯源树,研究论文发展脉络
Chat Paper
正在生成论文摘要