Prediction of Coastal Water Temperature Using Statistical Models
Estuaries and Coasts(2022)
摘要
Water temperature plays an important role in the equilibrium of the aquatic system and the overall health of aquaculture biota. Two machine learning models (random forests and artificial neural network) and regression model (multiple linear regression) were developed to estimate coastal sea surface temperatures (SST) in the Estuary and Gulf of St. Lawrence (Eastern Canada) after being trained on in situ data from available stations. The SST prediction is formulated as a regression model in function of a selected set of predictors. Different measures of correlation were used to examine the evolution of the SST as a function of meteorological (air temperature and wind speed), oceanographic (tidal range), and hydrological (flow) variables as well as teleconnection patterns in order to select the best inputs for the tested models. This study tested the abilities of commonly used regression model (Multiple Linear Regression) and machine learning models (Random Forests and Artificial Neural Network) to predict the daily SST, given the most relevant predictors. The results showed the importance of the selected predictors in SST prediction accuracy using the machine learning models, given that they capture the complex and non-linear relationship between the targeted SST and explanatory variables. The ANN model had shown the lowest root-mean-square error (RMSE) ranging between 0.82 °C and 2.64 °C, with an overall RMSE of 1.72 °C across all the stations against 1.96 °C using the RF.
更多查看译文
关键词
Coastal water temperature,Machine learning models,Statistical modeling
AI 理解论文
溯源树
样例
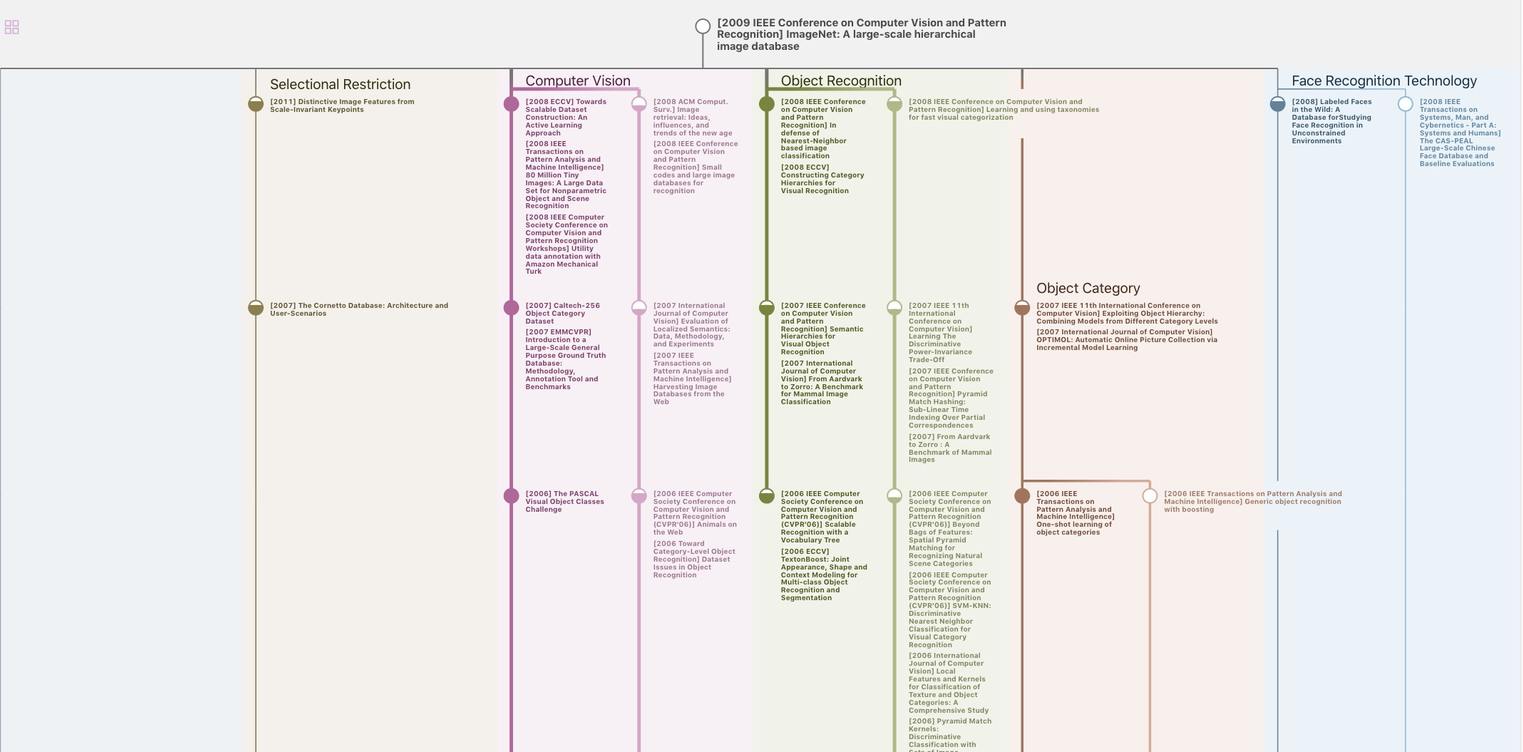
生成溯源树,研究论文发展脉络
Chat Paper
正在生成论文摘要