A correlation matrix-based tensor decomposition method for early prediction of sepsis from clinical data
BIOCYBERNETICS AND BIOMEDICAL ENGINEERING(2021)
摘要
Early detection of sepsis can assist in clinical triage and decision-making, resulting in early intervention with improved outcomes. This study aims to develop a machine learning framework to predict the onset of sepsis through EHR data by applying tensor decomposition on correlation matrices of clinical covariates for every record, arranged on an hourly basis for the length of stay (LOS) in intensive care unit. A third-order tensor theta(x,y,z) representing a clinical correlation among selected 24 covariates for a considered time frame of sepsis onset duration of 6 h, with a stride of 1 h is formed for each record. Such a fused tensor with dimensions theta is an element of R-24x24xLOS for every record undergoes Tucker decomposition with an optimal choice of rank. The factor matrices U-1, U-2, U-3 thus obtained after decomposition are excluded and only the core tensor sigma with a dimension sigma is an element of R-10x10xLOS has been retained, and used to provide latent features for prediction of sepsis onset. A five-fold cross-validation scheme is employed wherein the obtained 100 latent features from the reshaped core tensor, are fed to Light Gradient Boosting Machine Learning models (LightGBM) for binary classification, further alleviating the involved class imbalance. The machine-learning framework is designed via Bayesian optimization, yielding an average normalized utility score of 0.4314 on publicly available PhysioNet/Computing in Cardiology Challenge 2019 training data. The proposed tensor decomposition deciphers the higher-order interrelations among the considered clinical covariates for early prediction of sepsis and the results obtained are on par with existing state-of-the-art performances. (C) 2021 Nalecz Institute of Biocybernetics and Biomedical Engineering of the Polish Academy of Sciences. Published by Elsevier B.V. All rights reserved.
更多查看译文
关键词
Sepsis, Machine learning, Tensor factorization, Early prediction, Model-based diagnosis, PhysioNet
AI 理解论文
溯源树
样例
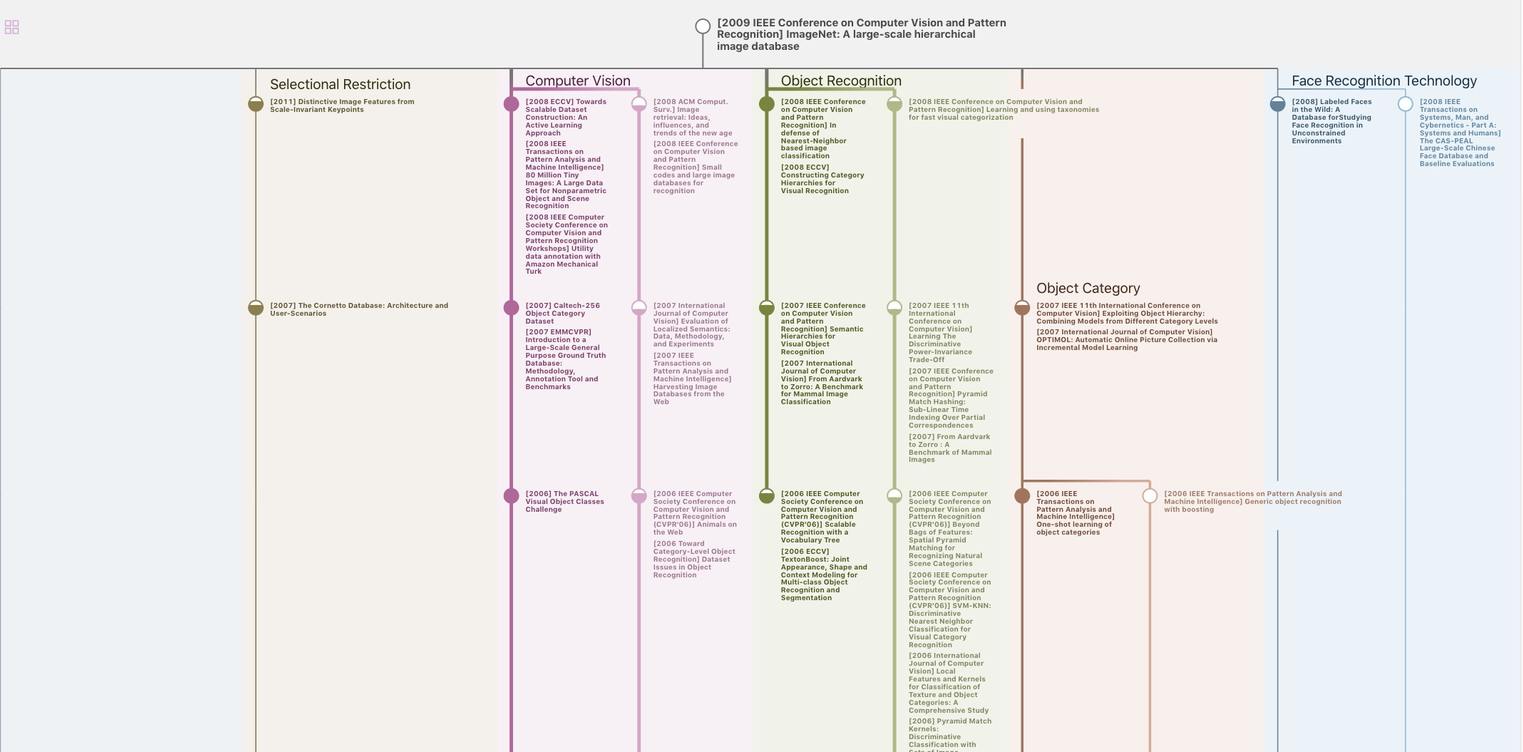
生成溯源树,研究论文发展脉络
Chat Paper
正在生成论文摘要