Optimising the photovoltaic parameters in donor–acceptor–acceptor ternary polymer solar cells using Machine Learning framework
Solar Energy(2022)
摘要
Clean energy is the need of the hour, considering the huge carbon footprint due to over-reliance on fossil fuels coupled with the exorbitantly rising global energy demand. Solar energy offers an abundant source of clean energy that can be harnessed using photovoltaic devices. Amongst the various generations of solar technologies, organic photovoltaics (OPVs) have emerged as an exigent technology. In this work, a diffuse-interface physics-based formulation has been utilised to generate a dataset, that has been used to decipher the complex process–microstructure–property relationship in Bulk-Heterojunction ternary OPVs comprising one donor and two acceptors. This has been achieved by using state-of-the-art Machine Learning (ML) technique wherein regression models have been fitted for correlating the solar cell parameters, namely power conversion efficiency (PCE), short-circuit current density (Jsc), and open-circuit potential (Voc) with BHJ morphology. The regression models for the electronic properties have further been employed to optimise a new set of morphologies, generated by finely spanning the ternary spinodal region, thus bypassing the need to carry out computationally intensive structure–property simulations. The electronic properties of the optimal morphology as predicted by the ML model have been validated with physics-based simulations. This work sets the motivation for exploiting an in silico paradigm to accelerate the optimisation of processing parameters to derive the maximal OPV performance.
更多查看译文
关键词
Ternary organic photovoltaics,Machine Learning,Optimisation
AI 理解论文
溯源树
样例
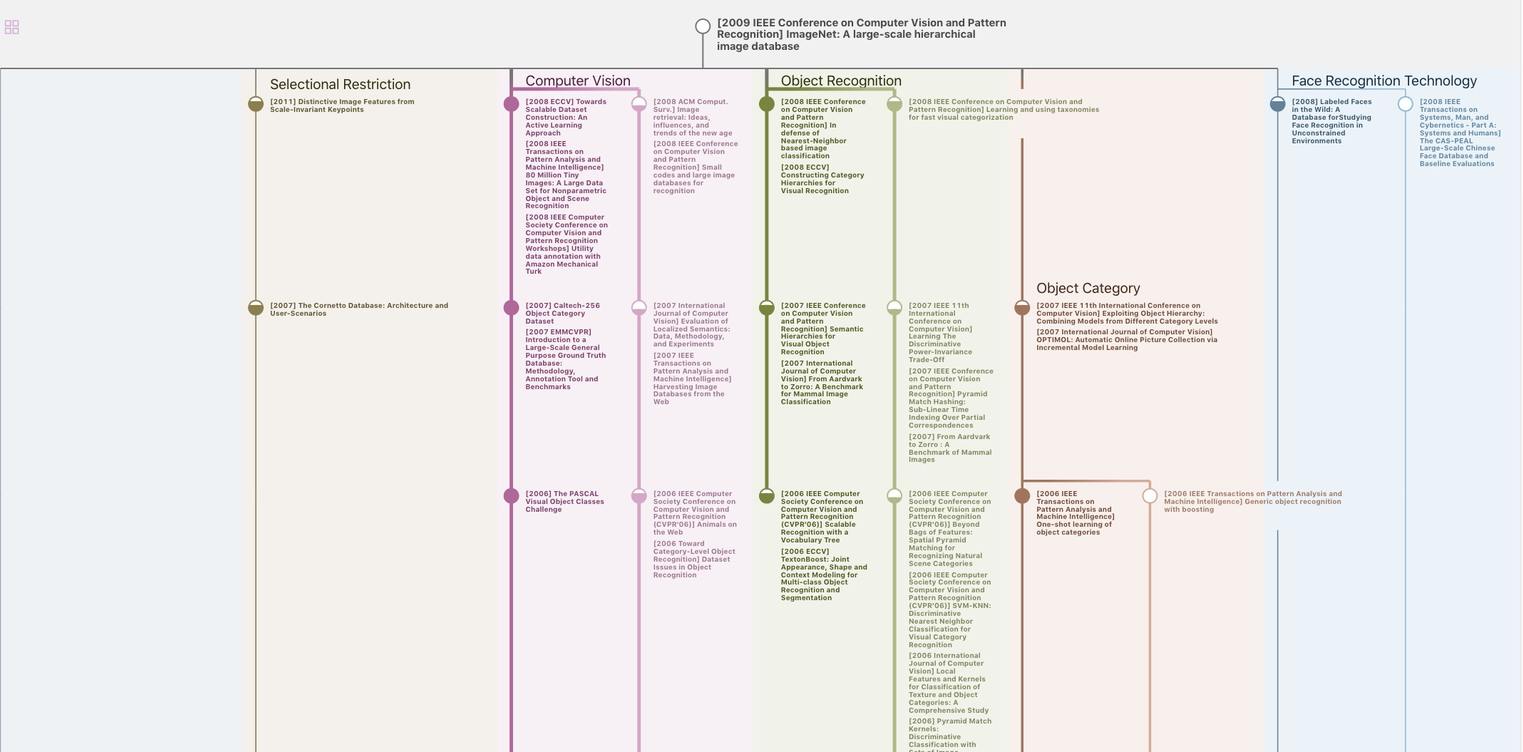
生成溯源树,研究论文发展脉络
Chat Paper
正在生成论文摘要