Multi-objective flower pollination algorithm: a new technique for EEG signal denoising
NEURAL COMPUTING & APPLICATIONS(2022)
摘要
The electroencephalogram (EEG) signal denoising problem has been considered a challenging task because of several artifact noises, such as eye blinking, eye movement, muscle activity, and power line interference, which can corrupt the original EEG signal during the recording time. Therefore, to remove these noises, the EEG signals must be processed to obtain efficient EEG features. Accordingly, several techniques have been proposed to reduce EEG noises, such as EEG signal denoising using wavelet transform (WT). The success of WT depends on the best configuration of its control parameters, which are often experimentally set. In this study, a multi-objective flower pollination algorithm (MOFPA) with WT (MOFPA-WT) is proposed to solve the EEG signal denoising problem. The novelty of this study is to find optimal EEG signal denoising parameters using MOFPA based on two measurement criteria for the denoised signals, namely minimum mean squared error (MSE) and maximum signal-to-noise ratio (SNR). The MOFPA-WT is tested using a standard EEG signal processing dataset, namely the EEG motor movement/imagery dataset. The performance of MOFPA-WT is evaluated using five criteria, namely SNR, SNR improvement, MSE, root mean squared error (RMSE), and percentage root mean square difference (PRD). Experiments are conducted using FPA with MSE, SNR, and MSE and SNR to show the effect of the multi-objective aspects on the performance of the proposed MOFPA-WT. Results show that FPA with MSE and SNR exhibits more subjective results than FPA with MSE and FPA with SNR. The convergence rate and Pareto front are also studied for the proposed MOFPA-WT.
更多查看译文
关键词
Electroencephalogram, Signal denoising, Wavelet transform, Multi-objective, Flower pollination algorithm
AI 理解论文
溯源树
样例
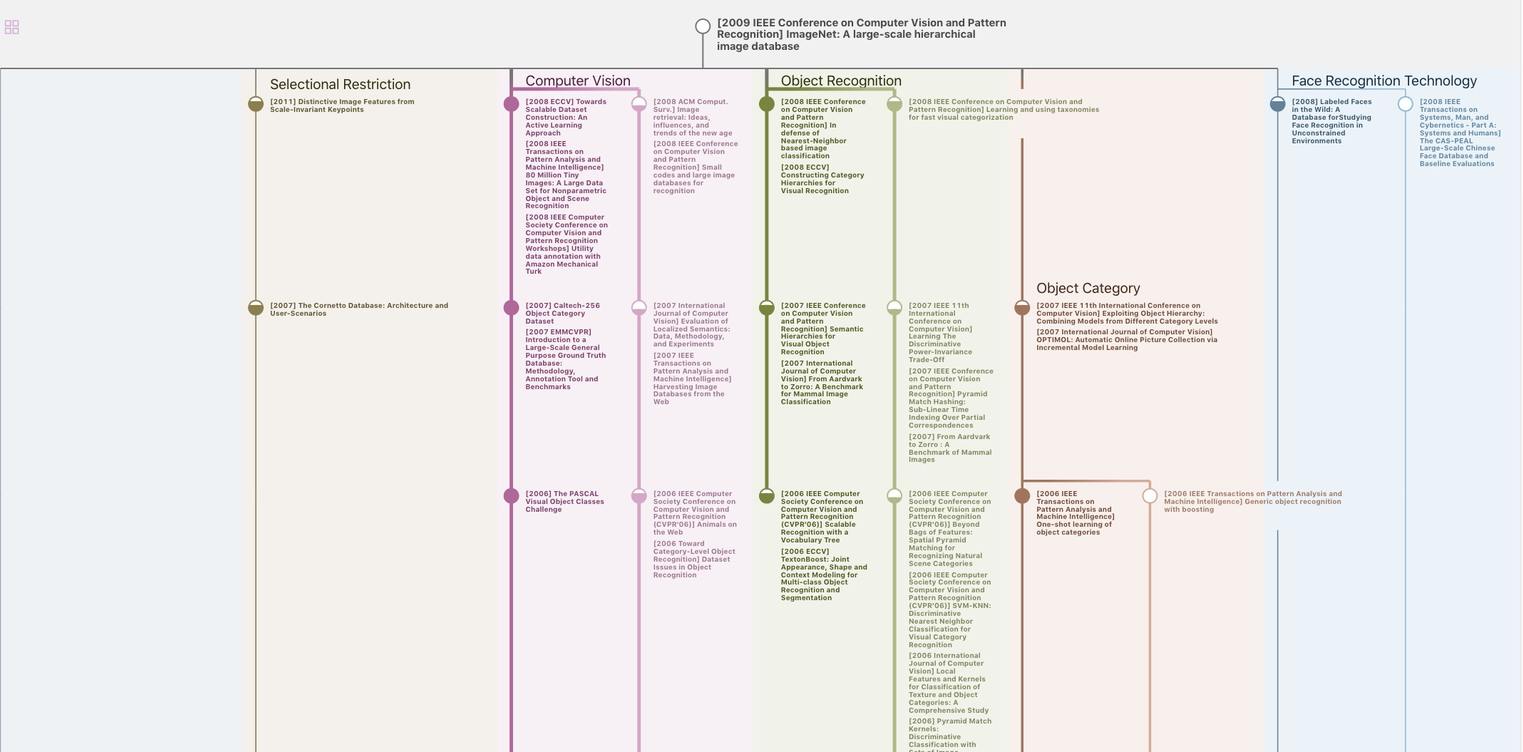
生成溯源树,研究论文发展脉络
Chat Paper
正在生成论文摘要