Interpolation of Irregularly Sampled Noisy Seismic Data with the Nonconvex Regularization and Proximal Method
PURE AND APPLIED GEOPHYSICS(2022)
摘要
Seismic data interpolation is an essential tool for providing complete seismic data when field data are incomplete due to the influence of obstacles, topography and acquisition cost. Among the existing interpolation methods, sparsity inversion-based methods are commonly used for noisy data interpolation. These methods assume that seismic data can be sparsely expressed in a transformed domain, and a sparse inversion should be solved to obtain sparse coefficients. The L 1 norm is often chosen as the regular operator since it is a convex function and can measure sparsity of solutions. Nonetheless, nonconvex regularization represented by the L 1/2 norm has better numerical properties than those of the L 1 norm regularization, since the L 1/2 norm is a closer expression of sparsity. Based on the idea of nonconvex regularization, a novel nonconvex regularization model was developed to realize seismic interpolation. The L 1 norm and a nonconvex, hyperbolic tangent-based function were combined as the regularization constraint, and a revised proximal method was proposed to efficiently solve the inversion model. A revised Newton direction of the nonconvex term was used to ensure that the proposed method gave stable results. Synthetic and field data examples demonstrate that the proposed technique is especially useful for interpolating missing traces in recorded seismic data sets, and it is robust even when data are contaminated with noise.
更多查看译文
关键词
Inversion, nonconvex regularization, seismic interpolation, sparse optimization, sparsity
AI 理解论文
溯源树
样例
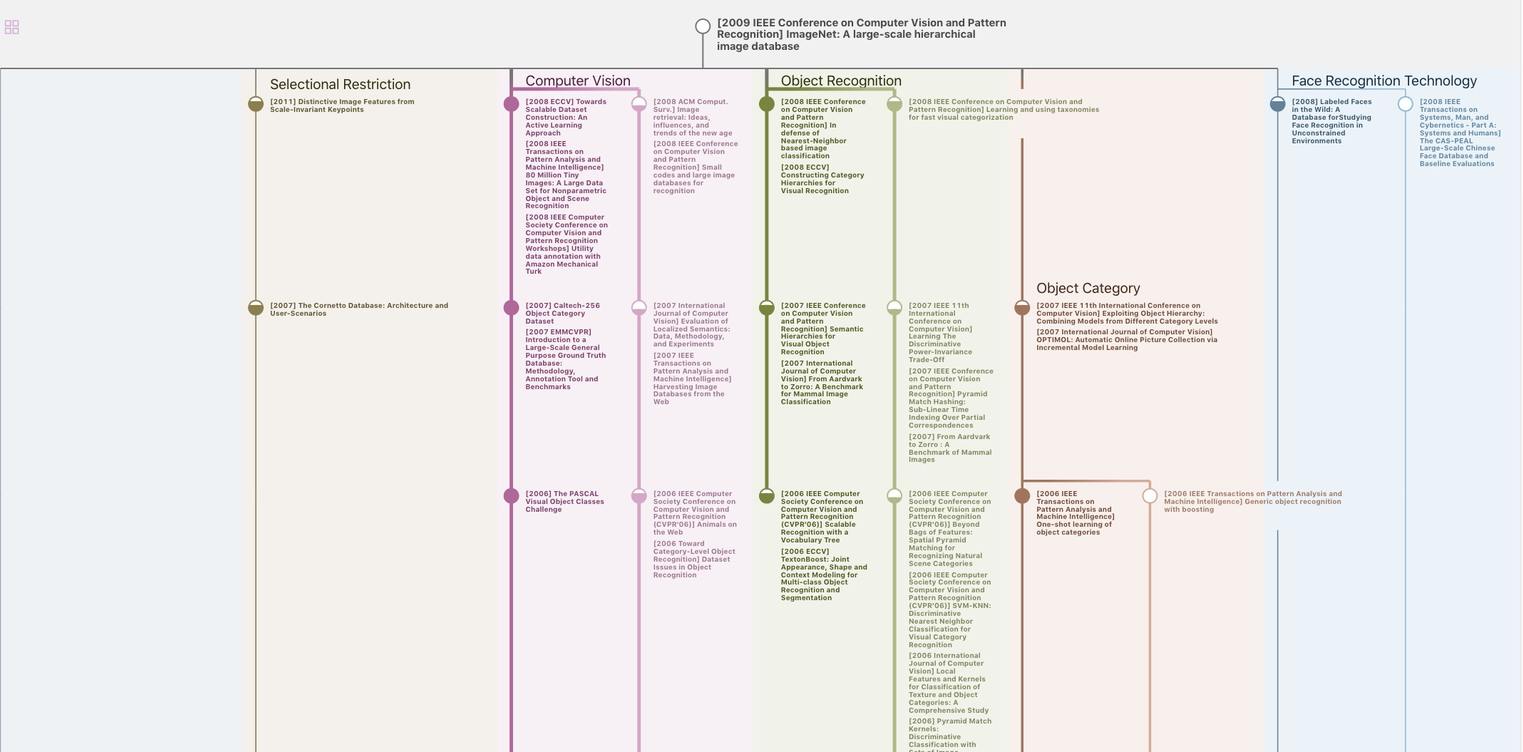
生成溯源树,研究论文发展脉络
Chat Paper
正在生成论文摘要