Penalized likelihood ratio test for a biomarker threshold effect in clinical trials based on generalized linear models
CANADIAN JOURNAL OF STATISTICS-REVUE CANADIENNE DE STATISTIQUE(2023)
Abstract
In a clinical trial, the responses to the new treatment may vary among patient subsets with different characteristics in a biomarker. It is often necessary to examine whether there is a cutpoint for the biomarker that divides the patients into two subsets of those with more favourable and less favourable responses. More generally, we approach this problem as a test of homogeneity in the effects of a set of covariates in generalized linear regression models. The unknown cutpoint results in a model with nonidentifiability and a nonsmooth likelihood function to which the ordinary likelihood methods do not apply. We first use a smooth continuous function to approximate the indicator function defining the patient subsets. We then propose a penalized likelihood ratio test to overcome the model irregularities. Under the null hypothesis, we prove that the asymptotic distribution of the proposed test statistic is a mixture of chi-squared distributions. Our method is based on established asymptotic theory, is simple to use, and works in a general framework that includes logistic, Poisson, and linear regression models. In extensive simulation studies, we find that the proposed test works well in terms of size and power. We further demonstrate the use of the proposed method by applying it to clinical trial data from the Digitalis Investigation Group (DIG) on heart failure.
MoreTranslated text
Key words
Biomarker cutpoint,clinical trials,generalized linear models,penalized likelihood ratio test,predictive biomarker
AI Read Science
Must-Reading Tree
Example
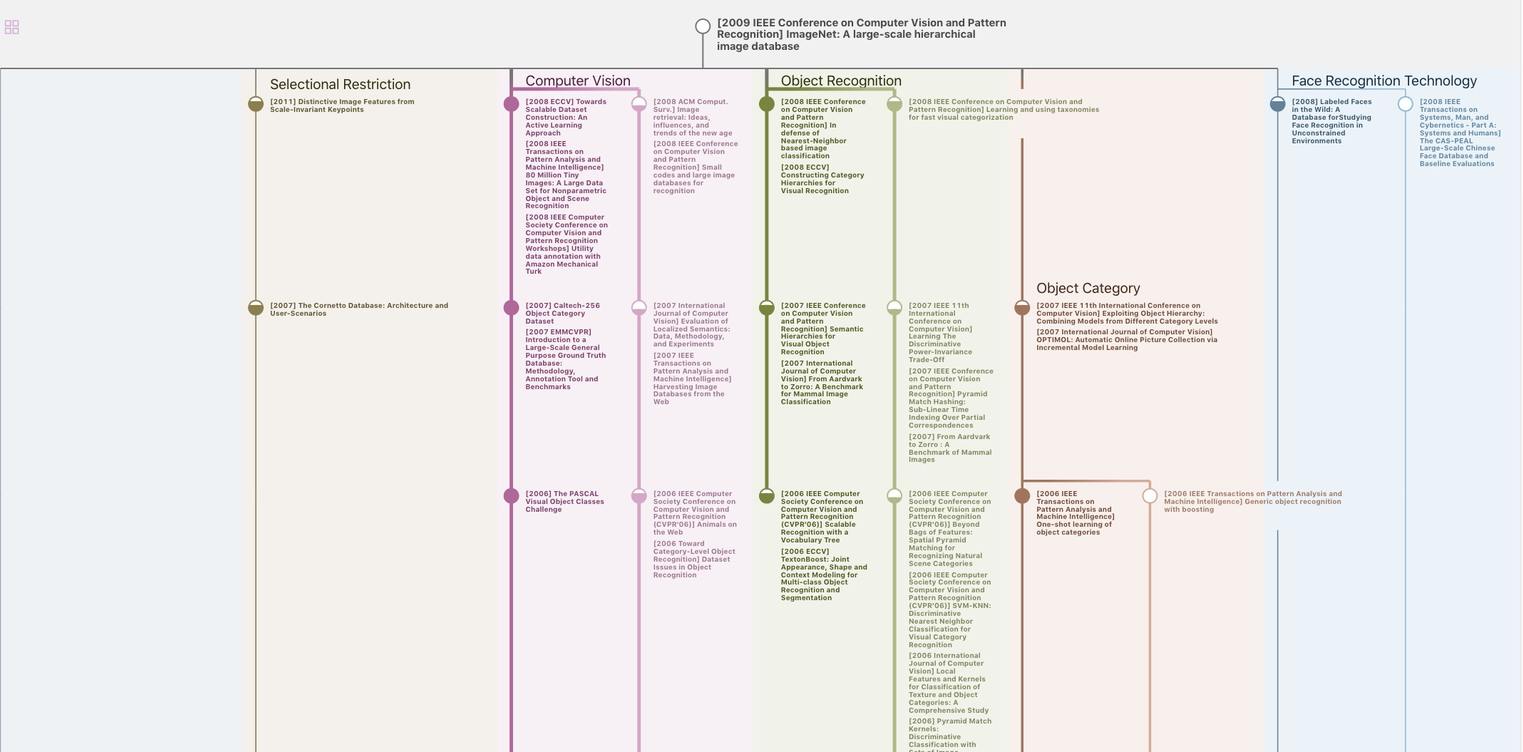
Generate MRT to find the research sequence of this paper
Chat Paper
Summary is being generated by the instructions you defined