Factor selection of product quotation with incomplete covering rough set
INTERNATIONAL JOURNAL OF PRODUCTION RESEARCH(2023)
摘要
Factor selection is crucial for any enterprise to make a quick and accurate quotation decision. For the objects of quotation with missing values, traditional rough sets construct their relations (e.g. tolerance, cover) either with objects having known values, or only with those that also include the missing values of the attribute domains. Such classifications may not work well for reduction in many real-world problems. In this paper, by measuring the similarity of objects, an incomplete covering rough set is proposed to derive a cover for attribute reduction. Firstly, a similarity relation is defined by an approximation degree that tunes the relation in line with the semantics of objects, and then a cover is induced. Secondly, a reduct is derived by the relations of objects with respect to covers; the properties of reduction are proven. Finally, an approach is developed by discernibility matrix. The experimental results of the UCI (University of California Irvine) Repository and real-life quotation data sets show the incomplete covering rough set outperforms the compared rough set in the accuracy of factor selection within the comparable computation time. It is also demonstrated that the proposed quotation model is effective in quote prediction with various proportions of missing data.
更多查看译文
关键词
Factor selection, quotation, attribute reduction, covering rough set, incomplete information system
AI 理解论文
溯源树
样例
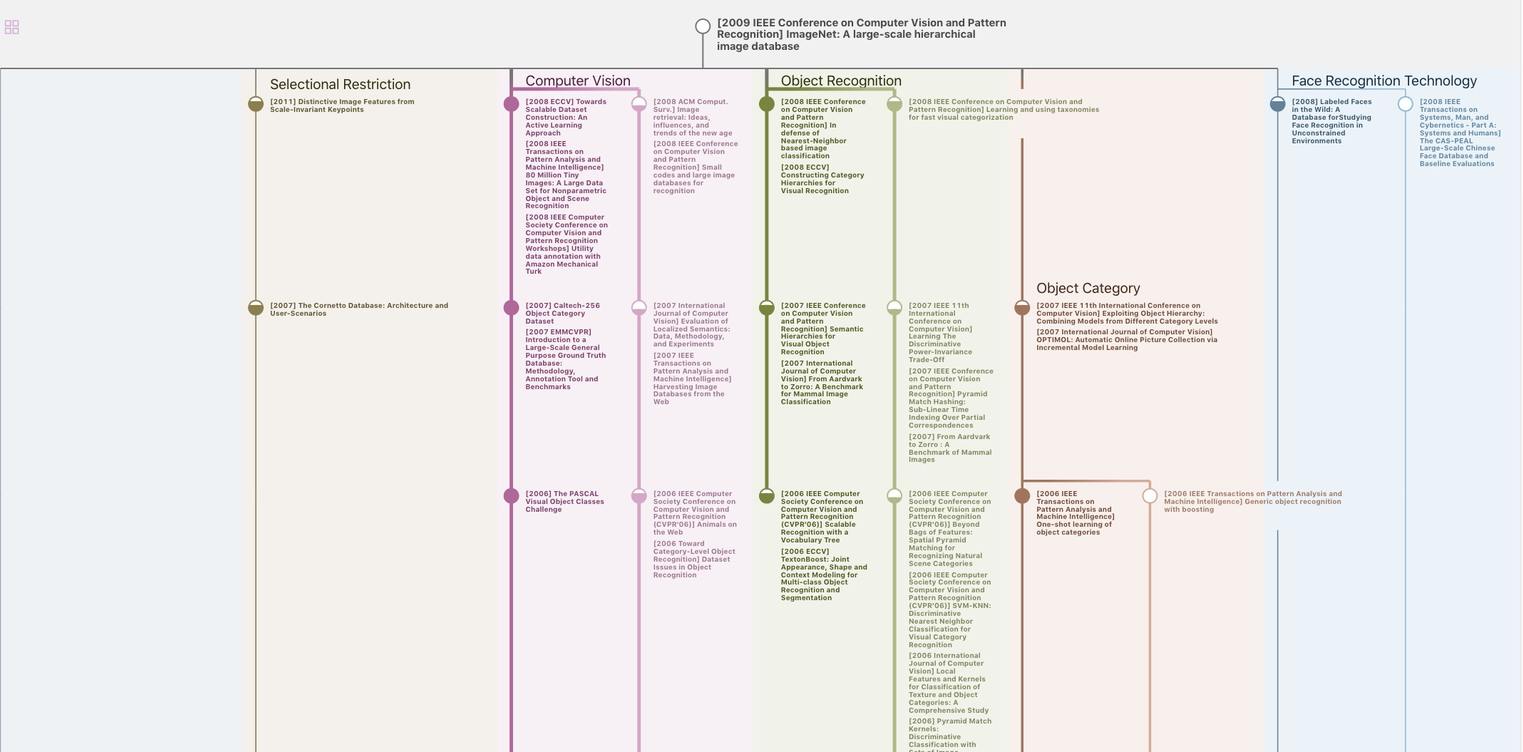
生成溯源树,研究论文发展脉络
Chat Paper
正在生成论文摘要