Machine learning prediction of the mechanical properties of -TiAl alloys produced using random forest regression model
Journal of Materials Research and Technology(2022)
摘要
The mechanical properties of a directionally solidified (DS) TiAl alloy were predicted through a random forest regression (RFR) machine learning algorithm. The prediction results were evaluated using the R-2 value. As a result, the R-2 values of prediction for the tensile strength, elongation, nanoindentation hardness, and interlamellar space were 0.9336, 0.9902, 0.8104, and 0.9810, respectively. To observe the correlation between the microstructure and mechanical properties, RFR prediction was conducted with a double input variable. This yielded a R-2 value of tensile strength of 0.9856, which was higher than the tensile strength derived with a single input variable. The R-2 value of nanoindentation hardness increased to 0.9902, which was higher than the nanoindentation hardness value with a single input variable. Through the use of the double input variables, the relationships among tensile strength and elongation, nanoindentation hardness, and interlamellar space were observed. Using feature importance, which could not be obtained in a previous study using the MLR algorithm, it was possible to determine which input variable had the most efficiency with respect to the output variable. Based on these research results, the speed and accuracy of new alloy development specifically in the design and processing, can be increased. In addition, metallurgical research on the relationship between the interlamellar space and mechanical properties was conducted, and the relationship was verified through the results of machine learning training. (C)& nbsp;2022 The Authors. Published by Elsevier B.V.& nbsp;
更多查看译文
关键词
Directionally solidified TiAl alloy,Microstructure control,Tensile strength,Interlamellar space,Prediction,Random forest regression
AI 理解论文
溯源树
样例
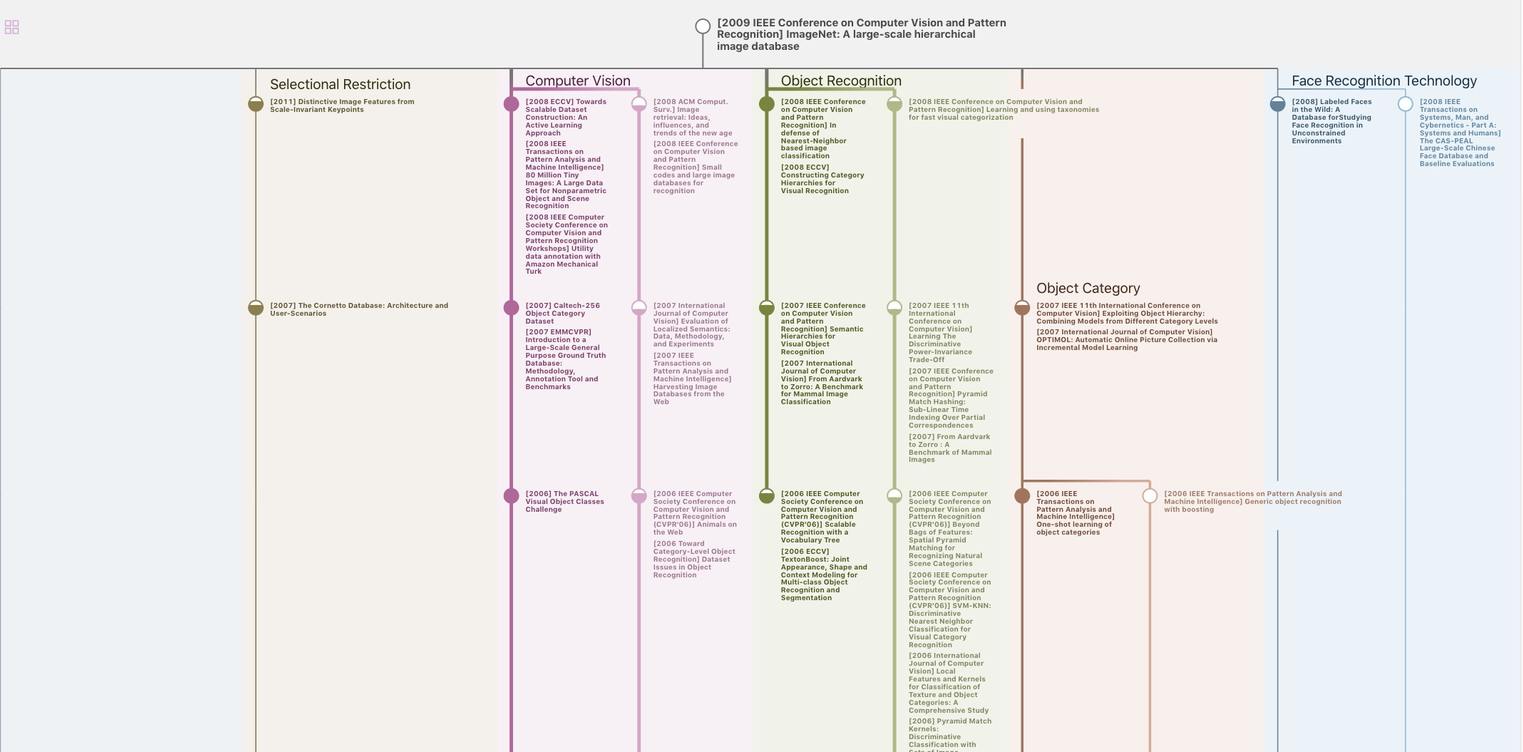
生成溯源树,研究论文发展脉络
Chat Paper
正在生成论文摘要