ASN-SMOTE: a synthetic minority oversampling method with adaptive qualified synthesizer selection
Complex & Intelligent Systems(2022)
摘要
Oversampling is a promising preprocessing technique for imbalanced datasets which generates new minority instances to balance the dataset. However, improper generated minority instances, i.e., noise instances, may interfere the learning of the classifier and impact it negatively. Given this, in this paper, we propose a simple and effective oversampling approach known as ASN-SMOTE based on the k -nearest neighbors and the synthetic minority oversampling technology (SMOTE). ASN-SMOTE first filters noise in the minority class by determining whether the nearest neighbor of each minority instance belongs to the minority or majority class. After that, ASN-SMOTE uses the nearest majority instance of each minority instance to effectively perceive the decision boundary, inside which the qualified minority instances are selected adaptively for each minority instance by the proposed adaptive neighbor selection scheme to synthesize new minority instance. To substantiate the effectiveness, ASN-SMOTE has been applied to three different classifiers and comprehensive experiments have been conducted on 24 imbalanced benchmark datasets. ASN-SMOTE is also extensively compared with nine notable oversampling algorithms. The results show that ASN-SMOTE achieves the best results in the majority of datasets. The ASN-SMOTE implementation is available at: https://www.github.com/yixinkai123/ASN-SMOTE/ .
更多查看译文
关键词
Imbalanced dataset, Oversampling, Synthetic minority oversampling, KNN, SMOTE, Noise filtering
AI 理解论文
溯源树
样例
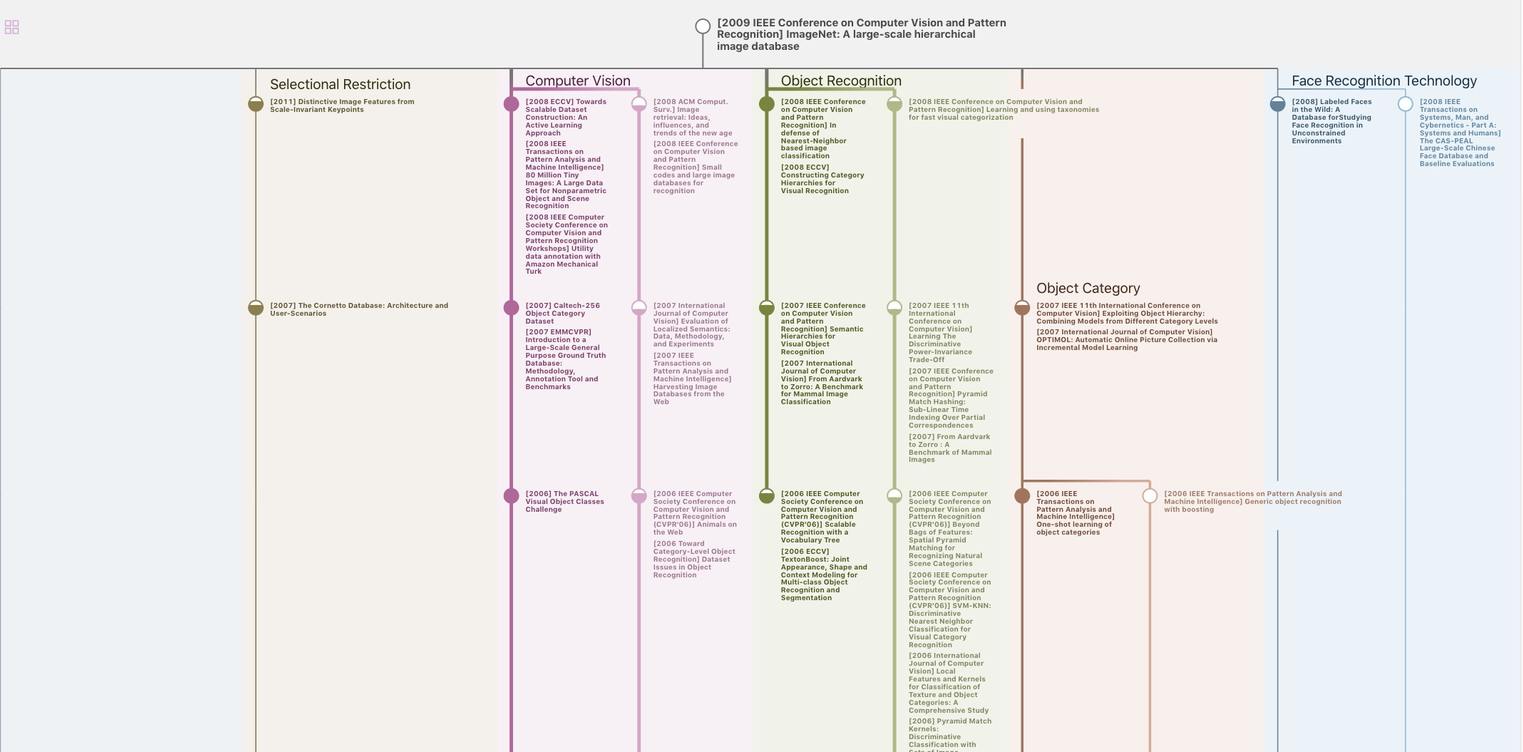
生成溯源树,研究论文发展脉络
Chat Paper
正在生成论文摘要