Neural Networks-Based Detection of Cyber-Physical Attacks Leading to Blackouts in Smart Grids
2021 13th IEEE PES Asia Pacific Power & Energy Engineering Conference (APPEEC)(2021)
摘要
Detection of cyberattacks leading to fail physical components has become a recent challenge in cyber-physical power systems. Cyber-physical attacks in terms of false data injections (FDIs) aiming to overflow multiple transmission lines are the worst type of attacks that might lead to cascading failures or blackouts. In this paper, an optimized single hidden layer neural network-based detection framework is developed to detect FDIs on targeted set of nodes leading to cascading failures. To increase the accuracy of the proposed single hidden layer neural network, Xavier's weight initialization method is adopted. Using an attack model, bad data was generated for one months to be used along with clean data for training of the proposed detection framework. Results on IEEE 118-bus benchmark confirm high accuracy with low computational complexity of the proposed algorithm in detection of cyber-physical attacks.
更多查看译文
关键词
Cyber-physical Attacks,Neural Networks,Smart grid security
AI 理解论文
溯源树
样例
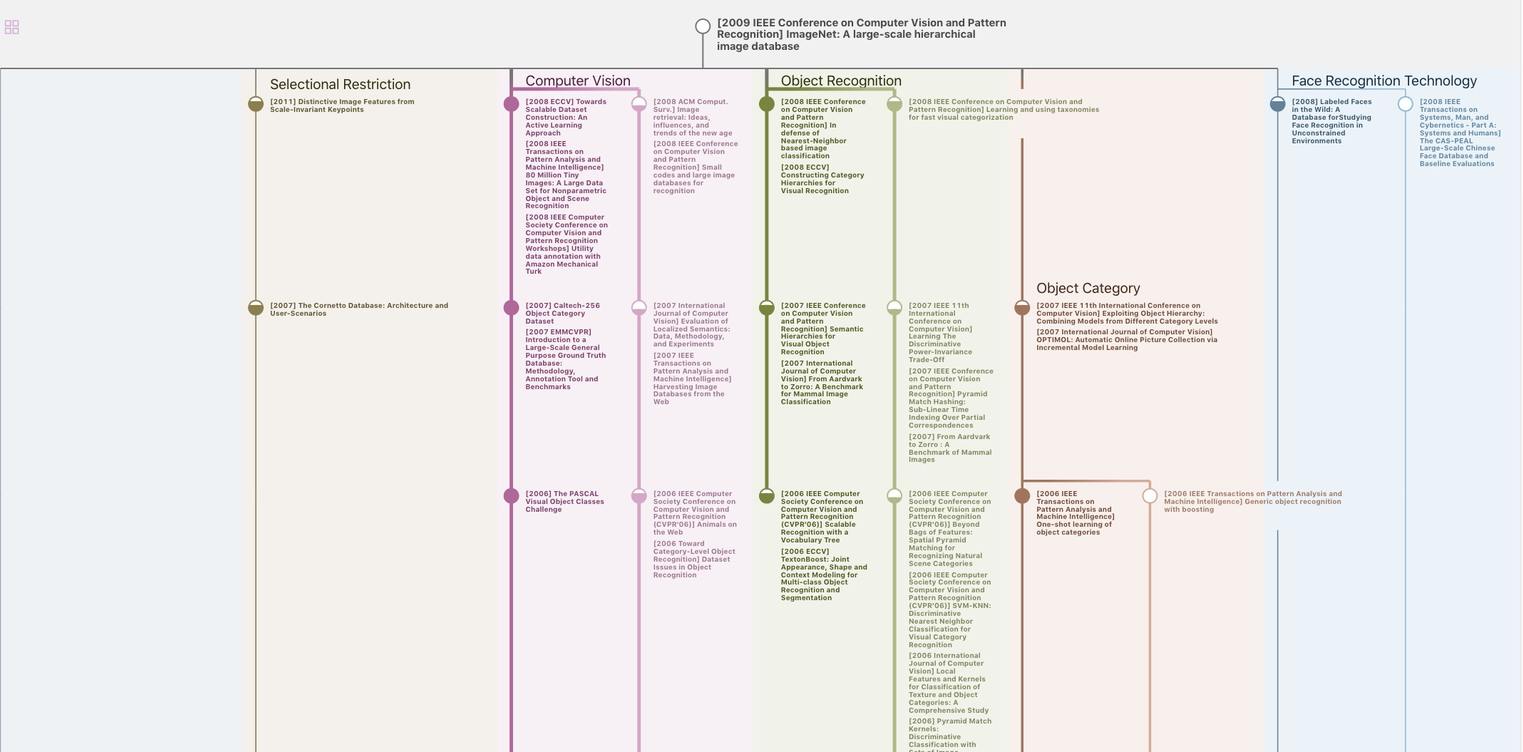
生成溯源树,研究论文发展脉络
Chat Paper
正在生成论文摘要