A survey of applied machine learning techniques for optical orthogonal frequency division multiplexing based networks
TRANSACTIONS ON EMERGING TELECOMMUNICATIONS TECHNOLOGIES(2022)
摘要
In this survey, we analyze the newest machine learning (ML) techniques applied in modern optical orthogonal frequency division multiplexing (O-OFDM) systems for access, core networks, and multi-channel transmission. From rudimentary to more advanced approaches, ML is proven to be a gold standard technique for signal quality improvement when low transmitter modulation extinction ratio dominates in coherent O-OFDM, and when stochastic-induced nonlinearities are present such as parametric noise amplification in long-haul transmission and the interplay between polarization-mode dispersion and the Kerr-induced nonlinearity. In addition, ML algorithms can effectively tackle determinist nonlinear distortions in O-OFDM networks, as well as inter-subcarrier nonlinear effects (ie, inter-subcarrier four-wave mixing and cross-phase modulation). In essence, ML techniques could be potentially beneficial for any multi-carrier approach (eg, filter bank modulation). The survey illustrates an extensive ML taxonomy for O-OFDM based networks, covering supervised, reinforcement learning and unsupervised ML categories. The transmission performance of various ML-assisted O-OFDM systems is presented taking into account the ML computational complexity toward real-time implementation. We also highlight the strict operating conditions for such systems under which a ML algorithm should perform classification, regression or clustering. Finally, the survey opens research issues and future directions toward ML implementation in radio-over-fiber (RoF) and 5G new radio (NR) systems.
更多查看译文
关键词
machine learning,networks
AI 理解论文
溯源树
样例
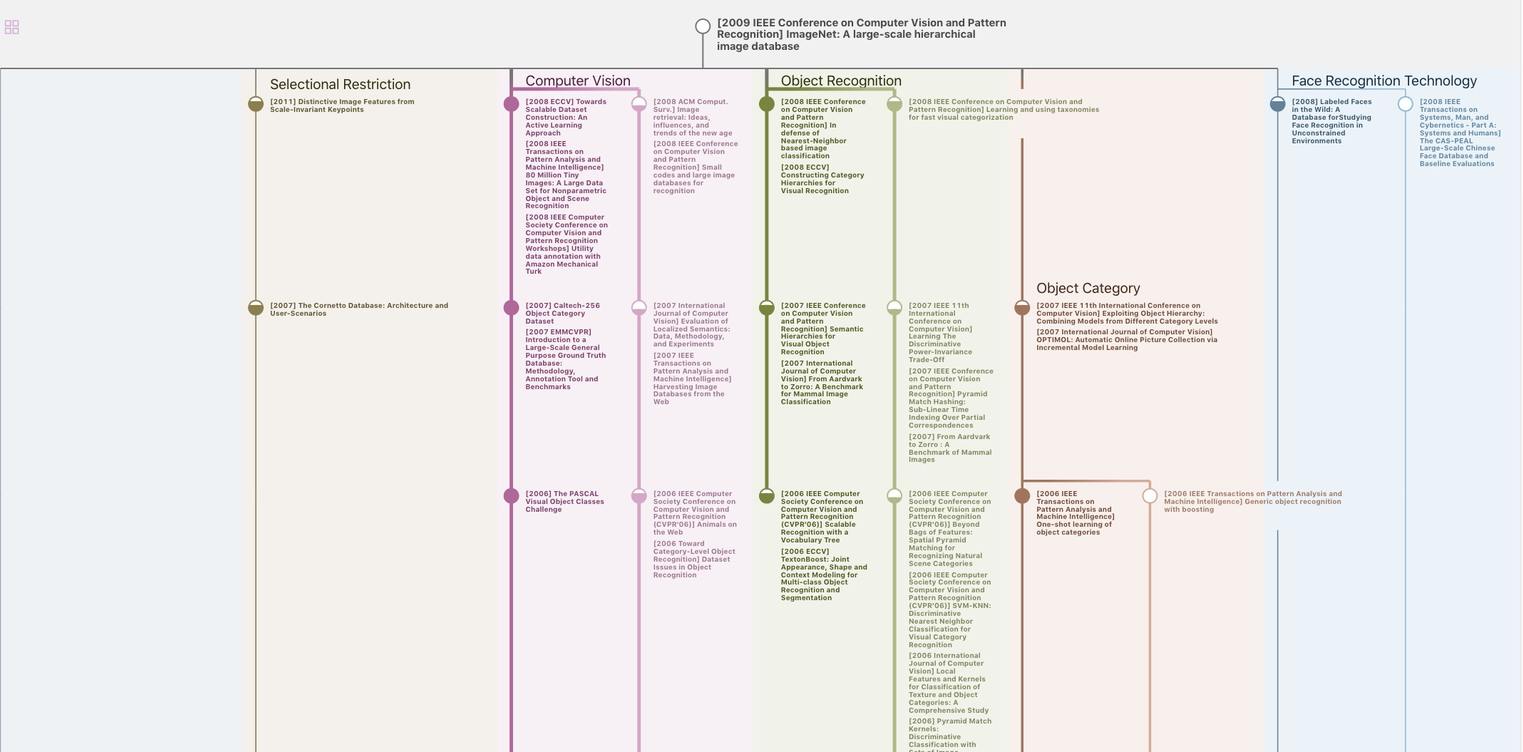
生成溯源树,研究论文发展脉络
Chat Paper
正在生成论文摘要