DDoS Detection in SDN using Machine Learning Techniques
CMC-COMPUTERS MATERIALS & CONTINUA(2022)
摘要
Software-defined network (SDN) becomes a new revolutionary paradigm in networks because it provides more control and network operation over a network infrastructure. The SDN controller is considered as the operating system of the SDN based network infrastructure, and it is responsible for executing the different network applications and maintaining the network services and functionalities. Despite all its tremendous capabilities, the SDN face many security issues due to the complexity of the SDN architecture. Distributed denial of services (DDoS) is a common attack on SDN due to its centralized architecture, especially at the control layer of the SDN that has a network-wide impact. Machine learning is now widely used for fast detection of these attacks. In this paper, some important feature selection methods for machine learning on DDoS detection are evaluated. The selection of optimal features reflects the classification accuracy of the machine learning techniques and the performance of the SDN controller. A comparative analysis of feature selection and machine learning classifiers is also derived to detect SDN attacks. The experimental results show that the Random forest (RF) classifier trains the more accurate model with 99.97% accuracy using features subset by the Recursive feature elimination (RFE) method.
更多查看译文
关键词
Machine learning, software-defined network, distributed denial of services, feature selection, protection, artificial neural network, decision trees, naive bayes, security
AI 理解论文
溯源树
样例
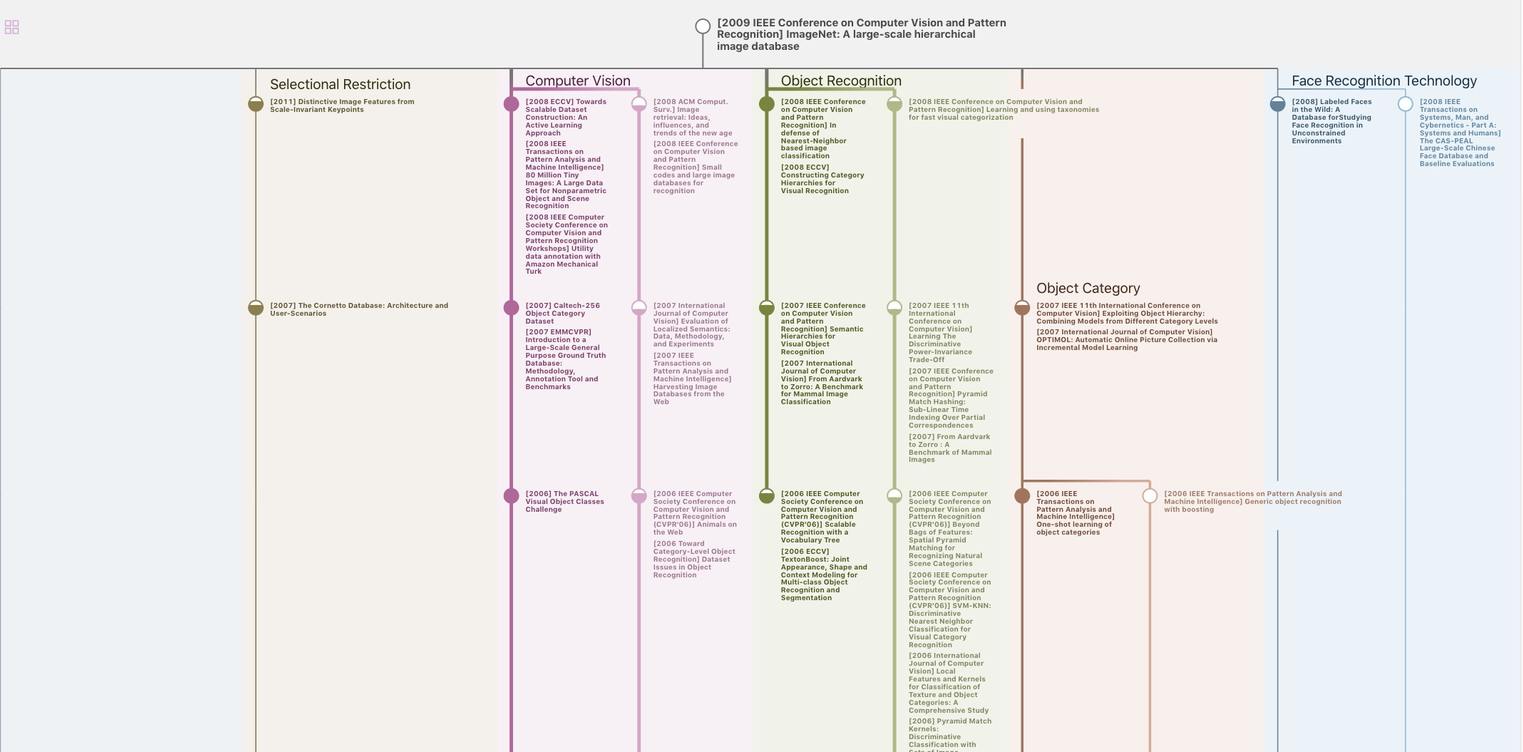
生成溯源树,研究论文发展脉络
Chat Paper
正在生成论文摘要