RL-CSL: A Combinatorial Optimization Method Using Reinforcement Learning and Contrastive Self-Supervised Learning
IEEE TRANSACTIONS ON EMERGING TOPICS IN COMPUTATIONAL INTELLIGENCE(2023)
摘要
Reinforcement learning-based methods have shown great potential in solving combinatorial optimization problems. However, the related research has not been mature in terms of both models and training methods. This paper proposes a method based on reinforcement learning and contrastive self-supervised learning. To be specific, the proposed method uses an attention model to learn a policy for generating solutions and combines a contrastive self-supervised learning model to learn the attention encoder in the way of node-by-node. Correspondingly, a two-phase learning method, including node-wise learning and solution-wise learning, is adopted to train the attention model and the contrastive self-supervised model jointly and collaboratively. The performance of the proposed method has been verified by numerical experiments on various combinatorial optimization problems.
更多查看译文
关键词
Learning systems,Optimization,Reinforcement learning,Computational modeling,Costs,Vehicle routing,Computational intelligence,Combinatorial optimization,reinforcement learning,attention model,self-supervised learning,contrastive learning
AI 理解论文
溯源树
样例
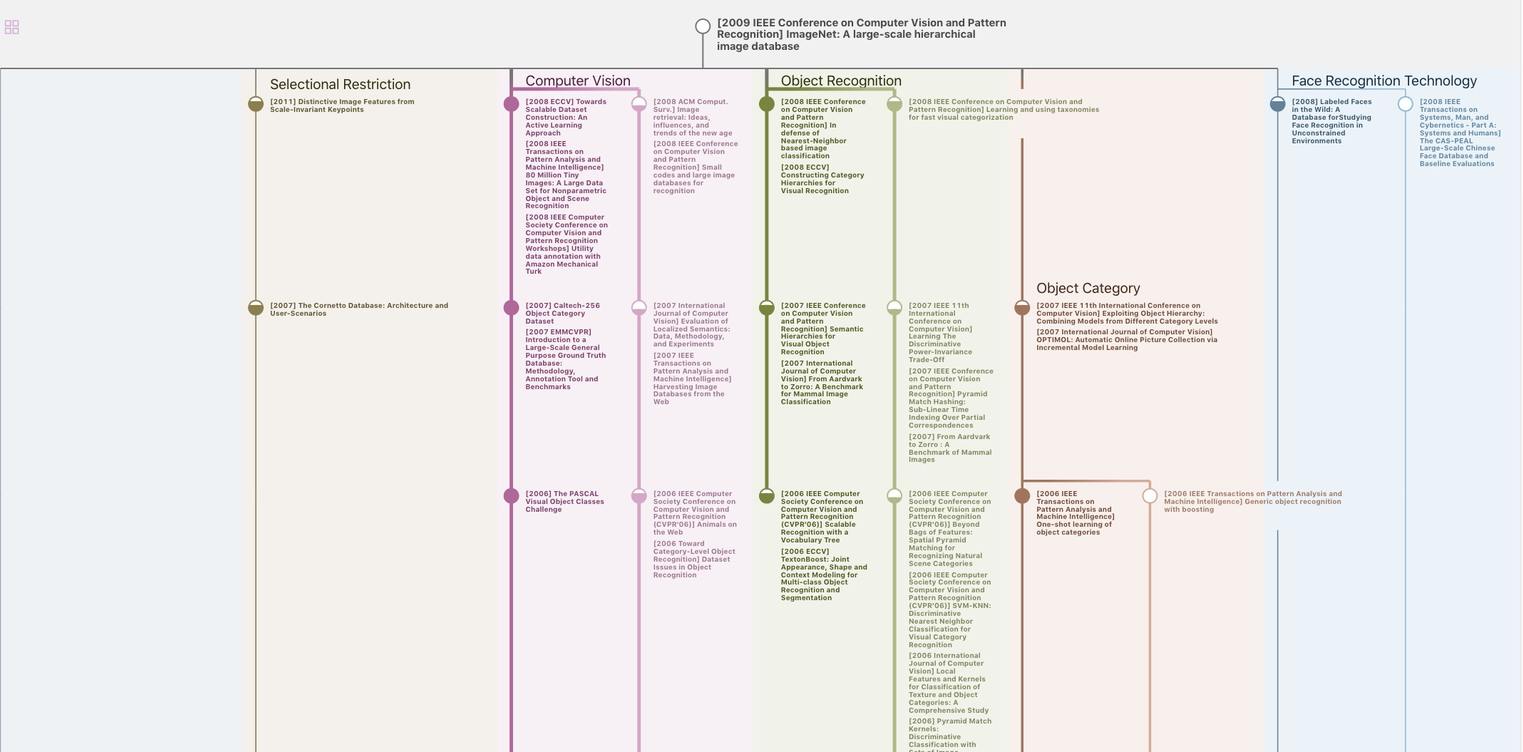
生成溯源树,研究论文发展脉络
Chat Paper
正在生成论文摘要