A Somatic Mutation Signature Predicts the Best Overall Response to Anti-programmed Cell Death Protein-1 Treatment in Epidermal Growth Factor Receptor/Anaplastic Lymphoma Kinase-Negative Non-squamous Non-small Cell Lung Cancer
FRONTIERS IN MEDICINE(2022)
Abstract
BackgroundWe aimed to exploit a somatic mutation signature (SMS) to predict the best overall response to anti-programmed cell death protein-1 (PD-1) therapy in non-small cell lung cancer (NSCLC). MethodsTumor samples of 248 patients with epidermal growth factor receptor (EGFR)/anaplastic lymphoma kinase (ALK)-negative non-squamous NSCLC treated with anti-PD-1 were molecularly tested by targeted next-generation sequencing or whole exome sequencing. On the basis of machine learning, we developed and validated a predictive model named SMS using the training (n = 83) and validation (n = 165) cohorts. ResultsThe SMS model comprising a panel of 15 genes (TP53, PTPRD, SMARCA4, FAT1, MGA, NOTCH1, NTRK3, INPP4B, KMT2A, PAK1, ATRX, BCOR, KDM5C, DDR2, and ARID1B) was built to predict best overall response in the training cohort. The areas under the curves of the training and validation cohorts were higher than those of tumor mutational burden and PD-L1 expression. Patients with SMS-high in the training and validation cohorts had poorer progression-free survival [hazard ratio (HR) = 6.01, P < 0.001; HR = 3.89, P < 0.001] and overall survival (HR = 7.60, P < 0.001; HR = 2.82, P < 0.001) than patients with SMS-low. SMS was an independent factor in multivariate analyses of progression-free survival and overall survival (HR = 4.32, P < 0.001; HR = 3.07, P < 0.001, respectively). ConclusionThis study revealed the predictive value of SMS for immunotherapy best overall response and prognosis in EGFR/ALK-negative non-squamous NSCLC as a potential biomarker in anti-PD-1 therapy.
MoreTranslated text
Key words
non-small cell lung cancer, anti-PD-1, best overall response, somatic mutations, EGFR, ALK negative
AI Read Science
Must-Reading Tree
Example
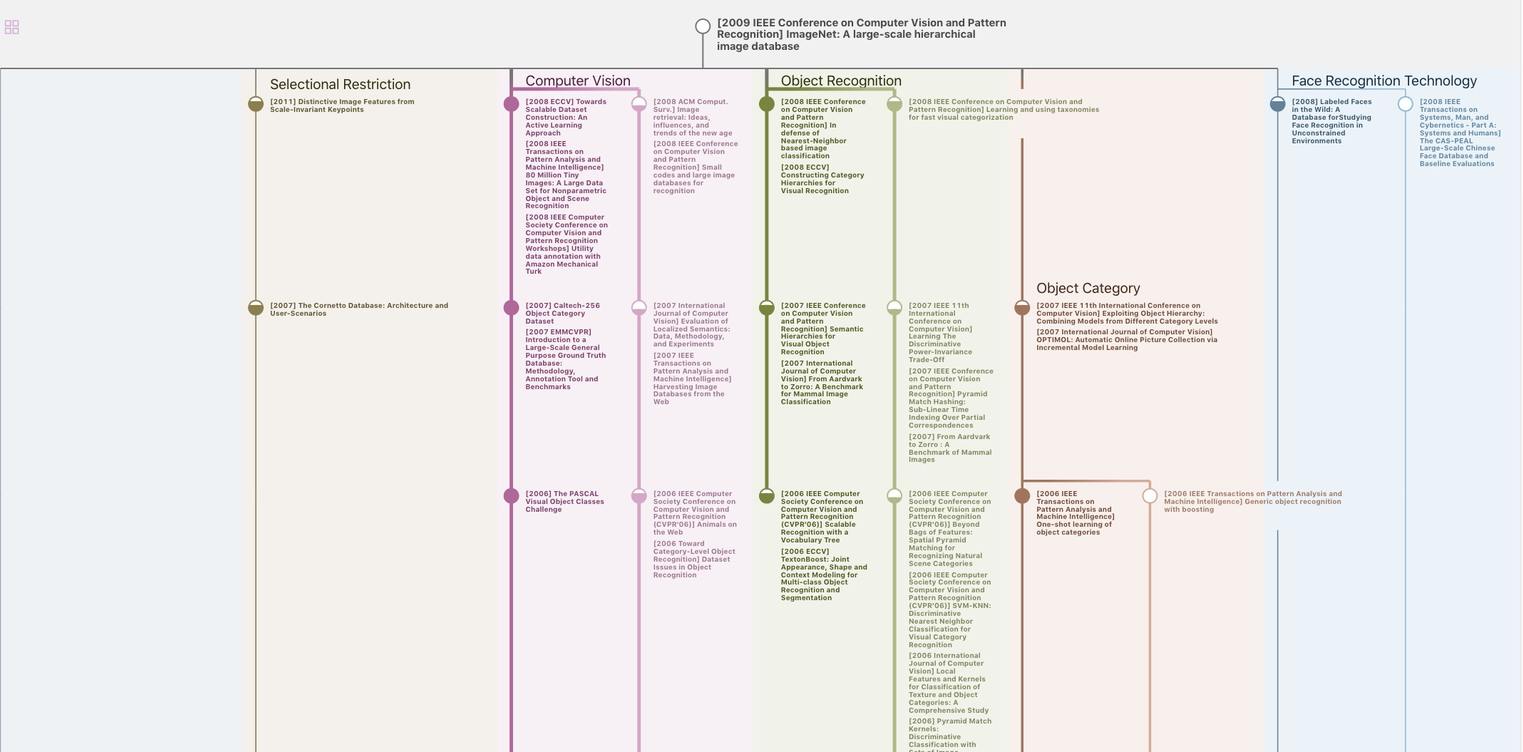
Generate MRT to find the research sequence of this paper
Chat Paper
Summary is being generated by the instructions you defined