Discriminative Geometric-Structure-Based Deep Hashing for Large-Scale Image Retrieval.
IEEE transactions on cybernetics(2022)
摘要
Deep hashing reaps the benefits of deep learning and hashing technology, and has become the mainstream of large-scale image retrieval. It generally encodes image into hash code with feature similarity preserving, that is, geometric-structure preservation, and achieves promising retrieval results. In this article, we find that existing geometric-structure preservation manner inadequately ensures feature discrimination, while improving feature discrimination of hash code essentially determines hash learning retrieval performance. This fact principally spurs us to propose a discriminative geometric-structure-based deep hashing method (DGDH), which investigates three novel loss terms based on class centers to induce the so-called discriminative geometrical structure. In detail, the margin-aware center loss assembles samples in the same class to the corresponding class centers for intraclass compactness, then a linear classifier based on class center serves to boost interclass separability, and the radius loss further puts different class centers on a hypersphere to tentatively reduce quantization errors. An efficient alternate optimization algorithm with guaranteed desirable convergence is proposed to optimize DGDH. We theoretically analyze the robustness and generalization of the proposed method. The experiments on five popular benchmark datasets demonstrate superior image retrieval performance of the proposed DGDH over several state of the arts.
更多查看译文
关键词
Quantization (signal),Binary codes,Image retrieval,Semantics,Feature extraction,Deep learning,Convolutional neural networks,Deep supervised hashing (DSH),discriminative ability,geometric structure,image retrieval
AI 理解论文
溯源树
样例
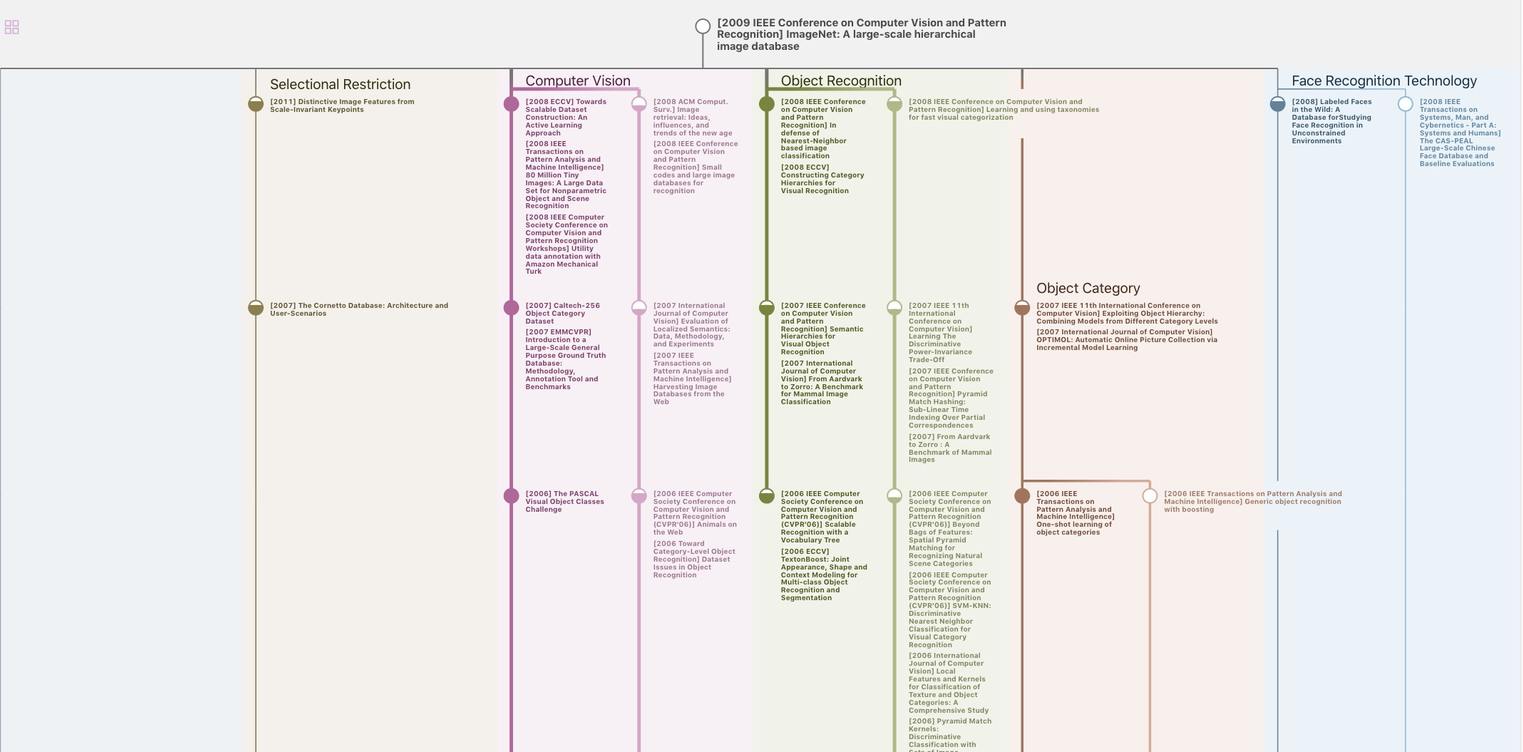
生成溯源树,研究论文发展脉络
Chat Paper
正在生成论文摘要