Machine learning imaging applications in the differentiation of true tumour progression from treatment-related effects in brain tumours: A systematic review and meta-analysis
JOURNAL OF MEDICAL IMAGING AND RADIATION ONCOLOGY(2022)
摘要
Introduction Chemotherapy and radiotherapy can produce treatment-related effects, which may mimic tumour progression. Advances in Artificial Intelligence (AI) offer the potential to provide a more consistent approach of diagnosis with improved accuracy. The aim of this study was to determine the efficacy of machine learning models to differentiate treatment-related effects (TRE), consisting of pseudoprogression (PsP) and radiation necrosis (RN), and true tumour progression (TTP). Methods The systematic review was conducted in accordance with PRISMA-DTA guidelines. Searches were performed on PubMed, Scopus, Embase, Medline (Ovid) and ProQuest databases. Quality was assessed according to the PROBAST and CLAIM criteria. There were 25 original full-text journal articles eligible for inclusion. Results For gliomas: PsP versus TTP (16 studies, highest AUC = 0.98), RN versus TTP (4 studies, highest AUC = 0.9988) and TRE versus TTP (3 studies, highest AUC = 0.94). For metastasis: RN vs. TTP (2 studies, highest AUC = 0.81). A meta-analysis was performed on 9 studies in the gliomas PsP versus TTP group using STATA. The meta-analysis reported a high sensitivity of 95.2% (95%CI: 86.6-98.4%) and specificity of 82.4% (95%CI: 67.0-91.6%). Conclusion TRE can be distinguished from TTP with good performance using machine learning-based imaging models. There remain issues with the quality of articles and the integration of models into clinical practice. Future studies should focus on the external validation of models and utilize standardized criteria such as CLAIM to allow for consistency in reporting.
更多查看译文
AI 理解论文
溯源树
样例
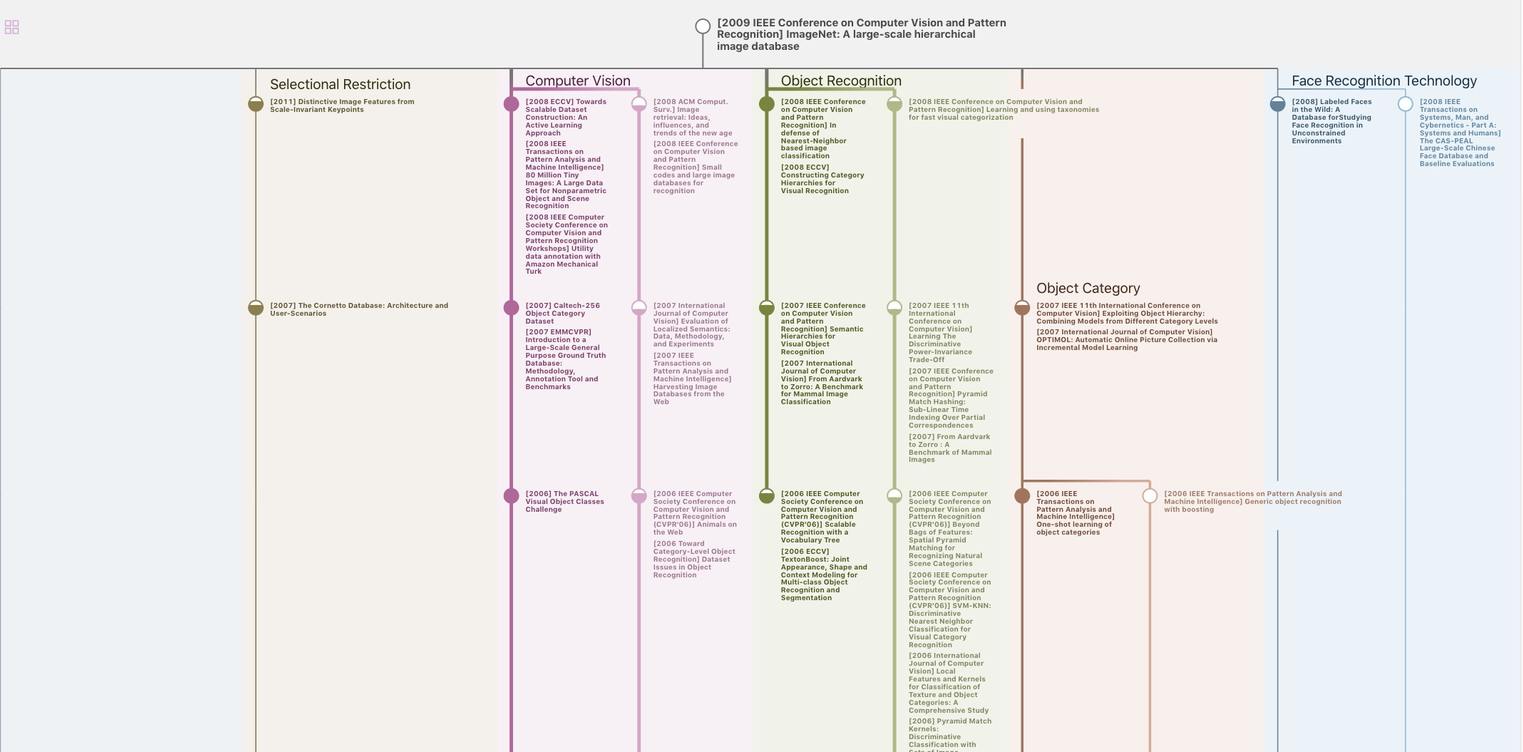
生成溯源树,研究论文发展脉络
Chat Paper
正在生成论文摘要