Concordance of Computed Tomography Regional Body Composition Analysis Using a Fully Automated Open-Source Neural Network versus a Reference Semi-Automated Program with Manual Correction
SENSORS(2022)
摘要
Quick, efficient, fully automated open-source programs to segment muscle and adipose tissues from computed tomography (CT) images would be a great contribution to body composition research. This study examined the concordance of cross-sectional areas (CSA) and densities for muscle, visceral adipose tissue (VAT), subcutaneous adipose tissue (SAT), and intramuscular adipose tissue (IMAT) from CT images at the third lumbar (L3) between an automated neural network (test method) and a semi-automatic human-based program (reference method). Concordance was further evaluated by disease status, sex, race/ethnicity, BMI categories. Agreement statistics applied included Lin's Concordance (CCC), Spearman correlation coefficient (SCC), Sorensen dice-similarity coefficient (DSC), and Bland-Altman plots with limits of agreement (LOA) within 1.96 standard deviation. A total of 420 images from a diverse cohort of patients (60.35 +/- 10.92 years; body mass index (BMI) of 28.77 +/- 7.04 kg/m(2); 55% female; 53% Black) were included in this study. About 30% of patients were healthy (i.e., received a CT scan for acute illness or pre-surgical donor work-up), while another 30% had a diagnosis of colorectal cancer. The CCC, SCC, and DSC estimates for muscle, VAT, SAT were all greater than 0.80 (>0.80 indicates good performance). Agreement analysis by diagnosis showed good performance for the test method except for critical illness (DSC 0.65-0.87). Bland-Altman plots revealed narrow LOA suggestive of good agreement despite minimal proportional bias around the zero-bias line for muscle, SAT, and IMAT CSA. The test method shows good performance and almost perfect concordance for L3 muscle, VAT, SAT, and IMAT per DSC estimates, and Bland-Altman plots even after stratification by sex, race/ethnicity, and BMI categories. Care must be taken to assess the density of the CT images from critically ill patients before applying the automated neural network (test method).
更多查看译文
关键词
computed tomography, body composition, validation, agreement, adipose tissue, muscle, automated segmentation, artificial intelligence
AI 理解论文
溯源树
样例
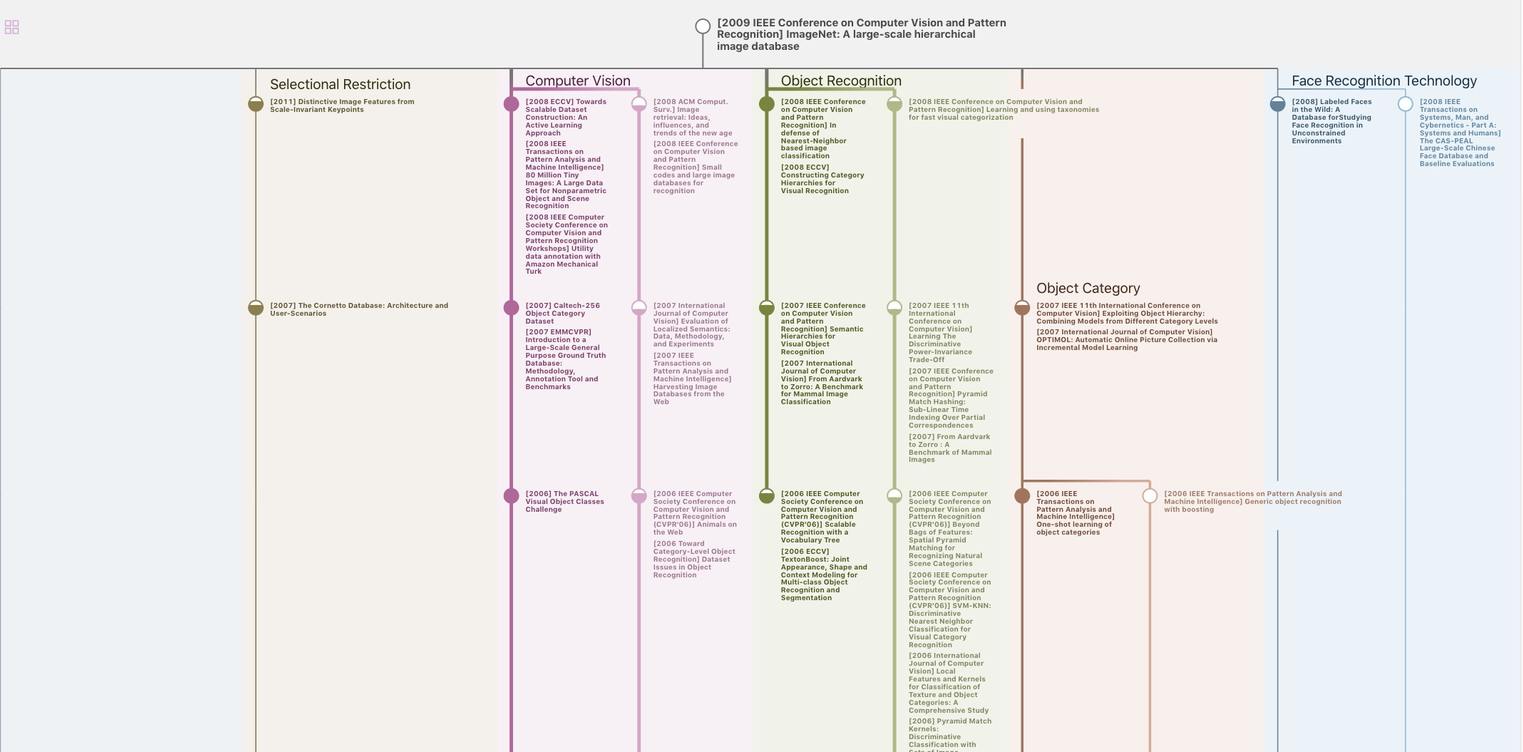
生成溯源树,研究论文发展脉络
Chat Paper
正在生成论文摘要