Meta-Learning Regrasping Strategies for Physical-Agnostic Objects
arxiv(2022)
摘要
Grasping inhomogeneous objects, practical use in real-world applications, remains a challenging task due to the unknown physical properties such as mass distribution and coefficient of friction. In this study, we propose a vision-based meta-learning algorithm to learn physical properties in an agnostic way. In particular, we employ Conditional Neural Processes (CNPs) on top of DexNet-2.0. CNPs learn physical embeddings rapidly from a few observations where each observation is composed of i) the cropped depth image, ii) the grasping height between the gripper and estimated grasping point, and iii) the binary grasping result. Our modified conditional DexNet-2.0 (DexNet-CNP) updates the predicted grasping quality iteratively from new observations, which can be executed in an online fashion. We evaluate our method in the Pybullet simulator using various shape primitive objects with different physical parameters. The results show that our model outperforms the original DexNet-2.0 and is able to generalize on unseen objects with different shapes.
更多查看译文
关键词
objects,meta-learning,physical-agnostic
AI 理解论文
溯源树
样例
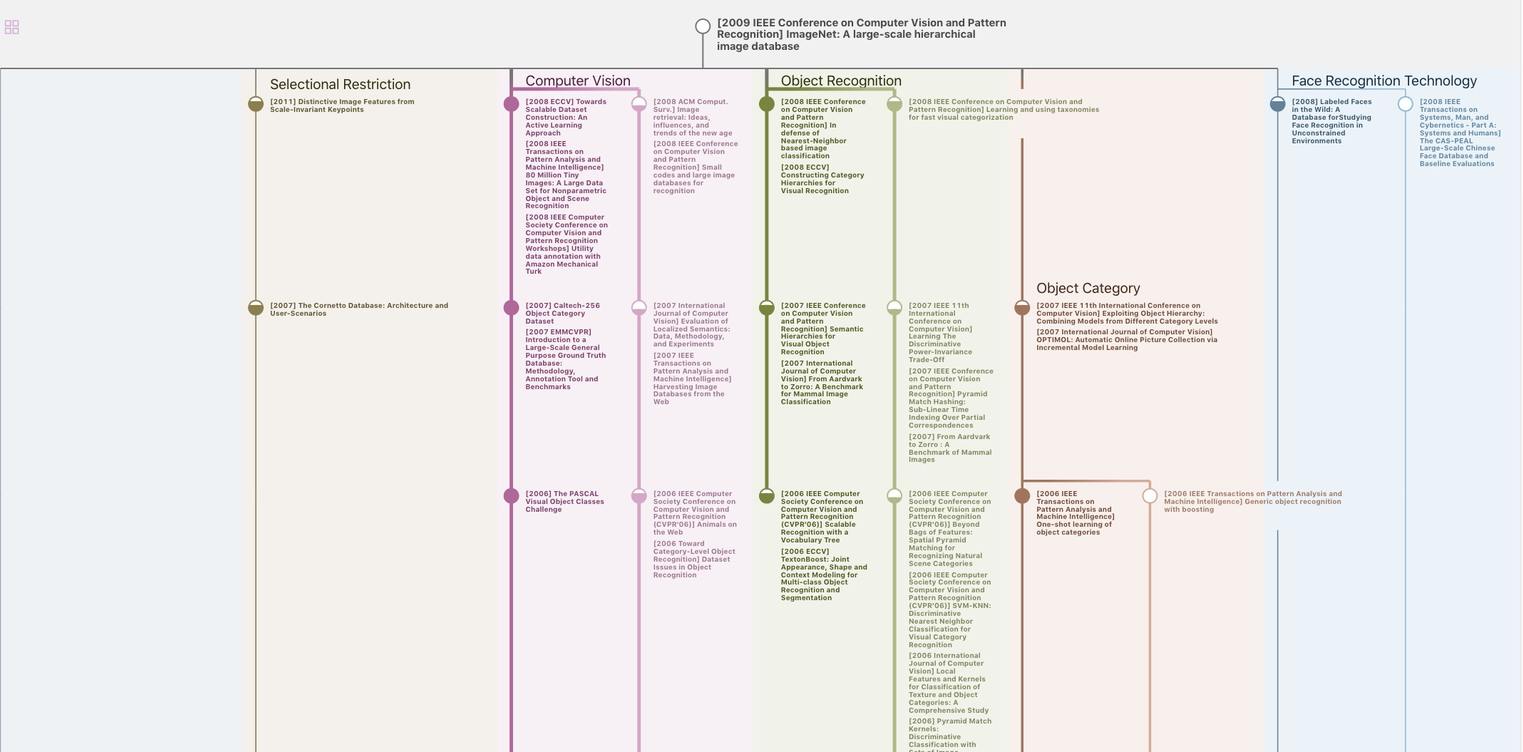
生成溯源树,研究论文发展脉络
Chat Paper
正在生成论文摘要