Toward smart composites: Small-scale, untethered prediction and control for soft sensor/actuator systems
JOURNAL OF COMPOSITE MATERIALS(2022)
摘要
We present formulation and open-source tools to achieve in-material model predictive control of sensor/actuator systems using learned forward kinematics and on-device computation. Microcontroller units that compute the prediction and control task while colocated with the sensors and actuators enable in-material untethered behaviors. In this approach, small parameter size neural network models learn forward kinematics offline. Our open-source compiler, nn4mc, generates code to offload these predictions onto MCUs. A Newton-Raphson solver then computes the control input in real time. We first benchmark this nonlinear control approach against a PID controller on a mass-spring-damper simulation. We then study experimental results on two experimental rigs with different sensing, actuation and computational hardware: a tendon-based platform with embedded LightLace sensors and a HASEL-based platform with magnetic sensors. Experimental results indicate effective high-bandwidth tracking of reference paths (>= 120 Hz) with a small memory footprint (<= 6.4% of flash memory). The measured path following error does not exceed 2mm in the tendon-based platform. The simulated path following error does not exceed I mm in the HASEL-based platform. The mean power consumption of this approach in an ARM Cortex-M4f device is 45.4 mW. This control approach is also compatible with Tensorflow Lite models and equivalent on-device code. In-material intelligence enables a new class of composites that infuse autonomy into structures and systems with refined artificial proprioception.
更多查看译文
关键词
soft robotics, feedback control, proprioception, soft sensors, soft actuators, firmware, machine learning
AI 理解论文
溯源树
样例
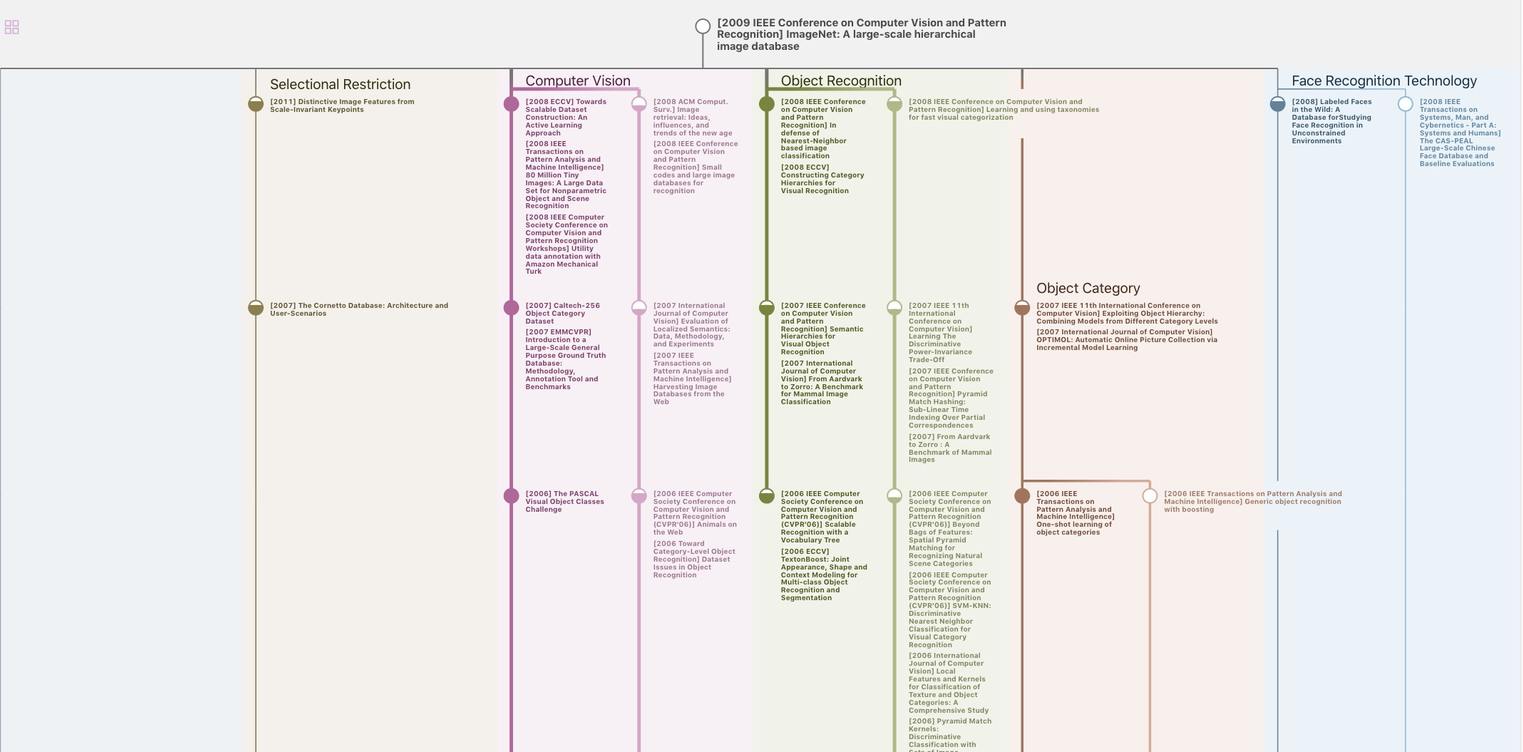
生成溯源树,研究论文发展脉络
Chat Paper
正在生成论文摘要