Spikemax: Spike-based Loss Methods for Classification
IEEE International Joint Conference on Neural Network (IJCNN)(2022)
摘要
Spiking Neural Networks~(SNNs) are a promising research paradigm for low power edge-based computing. Recent works in SNN backpropagation has enabled training of SNNs for practical tasks. However, since spikes are binary events in time, standard loss formulations are not directly compatible with spike output. As a result, current works are limited to using mean-squared loss of spike count. In this paper, we formulate the output probability interpretation from the spike count measure and introduce spike-based negative log-likelihood measure which are more suited for classification tasks especially in terms of the energy efficiency and inference latency. We compare our loss measures with other existing alternatives and evaluate using classification performances on three neuromorphic benchmark datasets: NMNIST, DVS Gesture and N-TIDIGITS18. In addition, we demonstrate state of the art performances on these datasets, achieving faster inference speed and less energy consumption.
更多查看译文
关键词
spike count measure,classification tasks,spike-based loss methods,spiking neural networks,low power edge-based computing,SNN backpropagation,binary events,standard loss formulations,mean-squared loss,output probability interpretation,spike-based negative log-likelihood measure
AI 理解论文
溯源树
样例
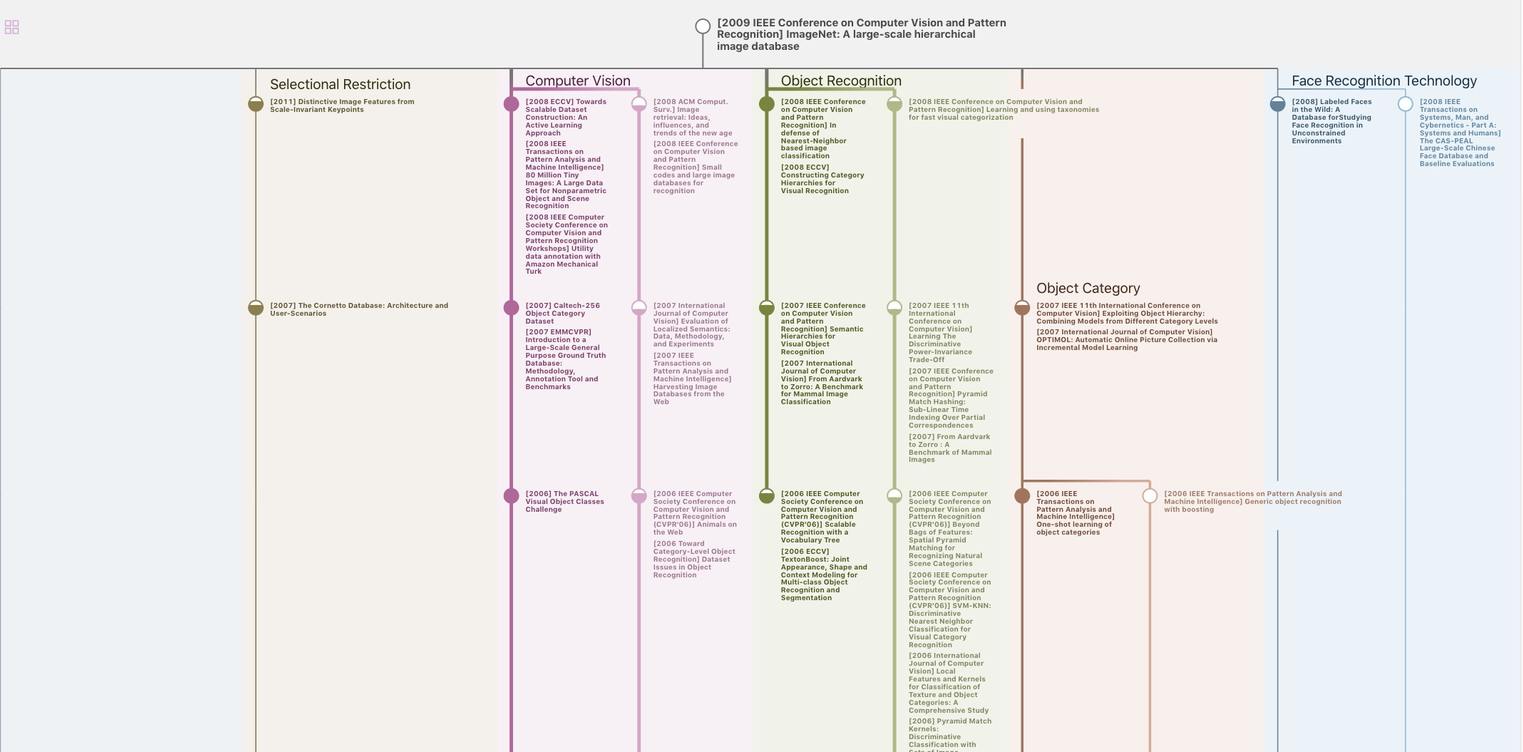
生成溯源树,研究论文发展脉络
Chat Paper
正在生成论文摘要