Deterministic Tensor Network Classifiers
arXiv (Cornell University)(2022)
摘要
We present tensor networks for feature extraction and refinement of classifier performance. These networks can be initialised deterministically and have the potential for implementation on near-term intermediate-scale quantum (NISQ) devices. Feature extraction proceeds through a direct combination and compression of images amplitude-encoded over just $\log N_{\text{pixels}}$ qubits. Performance is refined using `Quantum Stacking', a deterministic method that can be applied to the predictions of any classifier regardless of structure, and implemented on NISQ devices using data re-uploading. These procedures are applied to a tensor network encoding of data, and benchmarked against the 10 class MNIST and fashion MNIST datasets. Good training and test accuracy are achieved without any variational training.
更多查看译文
关键词
network
AI 理解论文
溯源树
样例
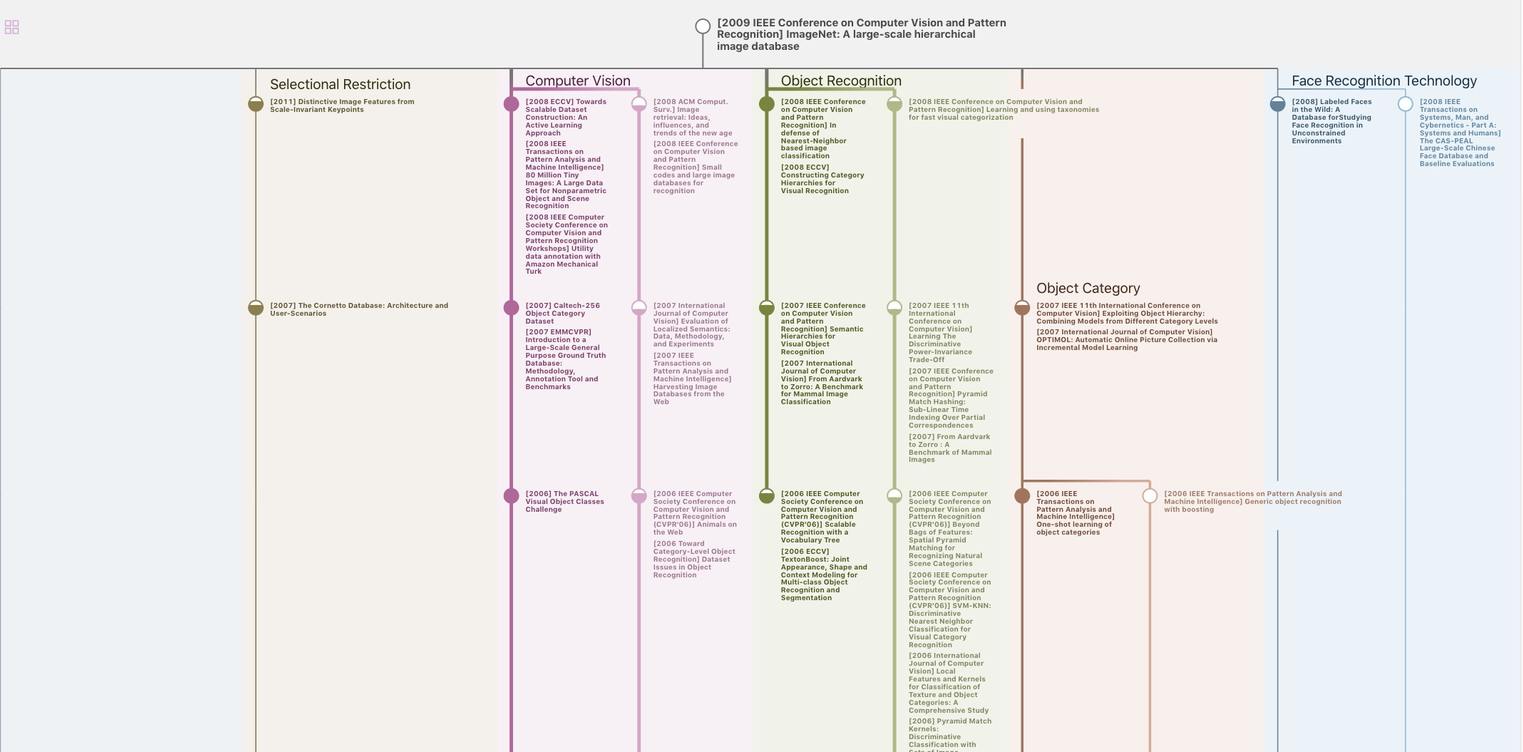
生成溯源树,研究论文发展脉络
Chat Paper
正在生成论文摘要