VGN: Value Decomposition With Graph Attention Networks for Multiagent Reinforcement Learning
IEEE TRANSACTIONS ON NEURAL NETWORKS AND LEARNING SYSTEMS(2024)
摘要
Although value decomposition networks and the follow on value-based studies factorizes the joint reward function to individual reward functions for a kind of cooperative multiagent reinforcement problem, in which each agent has its local observation and shares a joint reward signal, most of the previous efforts, however, ignored the graphical information between agents. In this article, a new value decomposition with graph attention network (VGN) method is developed to solve the value functions by introducing the dynamical relationships between agents. It is pointed out that the decomposition factor of an agent in our approach can be influenced by the reward signals of all the related agents and two graphical neural network-based algorithms (VGN-Linear and VGN-Nonlinear) are designed to solve the value functions of each agent. It can be proved theoretically that the present methods satisfy the factorizable condition in the centralized training process. The performance of the present methods is evaluated on the StarCraft Multiagent Challenge (SMAC) benchmark. Experiment results show that our method outperforms the state-of-the-art value-based multiagent reinforcement algorithms, especially when the tasks are with very hard level and challenging for existing methods.
更多查看译文
关键词
Mathematical models,Task analysis,Games,Q-learning,Neural networks,Behavioral sciences,Training,Deep learning,graph attention networks (GATs),multiagent systems,reinforcement learning
AI 理解论文
溯源树
样例
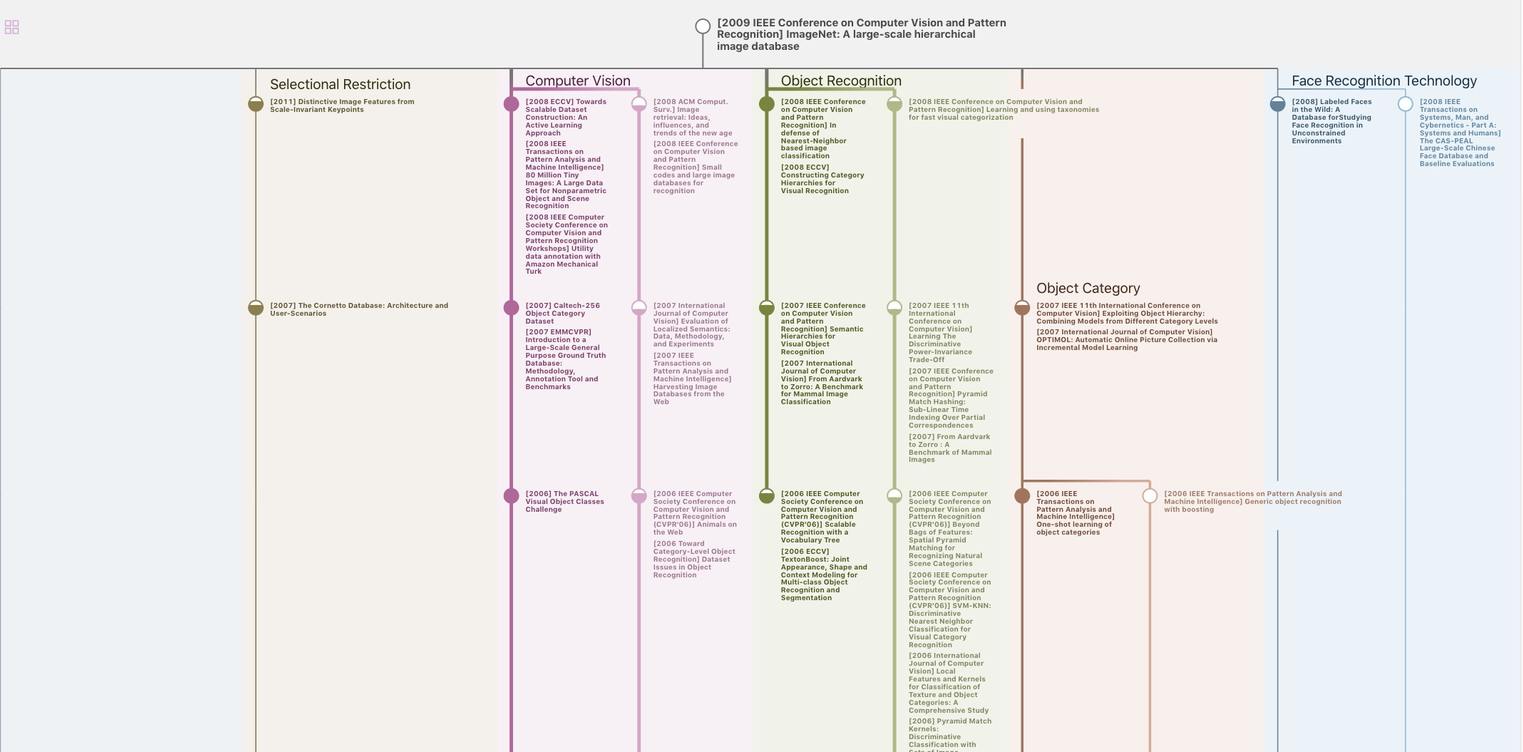
生成溯源树,研究论文发展脉络
Chat Paper
正在生成论文摘要