A Computational Platform Integrating a Mechanistic Model of Crohn’s Disease for Predicting Temporal Progression of Mucosal Damage and Healing
Advances in Therapy(2022)
摘要
Introduction Physicians are often required to make treatment decisions for patients with Crohn’s disease on the basis of limited objective information about the state of the patient’s gastrointestinal tissue while aiming to achieve mucosal healing. Tools to predict changes in mucosal health with treatment are needed. We evaluated a computational approach integrating a mechanistic model of Crohn’s disease with a responder classifier to predict temporal changes in mucosal health. Methods A hybrid mechanistic–statistical platform was developed to predict biomarker and tissue health time courses in patients with Crohn’s disease. Eligible patients from the VERSIFY study ( n = 69) were classified into archetypical response cohorts using a decision tree based on early treatment data and baseline characteristics. A virtual patient matching algorithm assigned a digital twin to each patient from their corresponding response cohort. The digital twin was used to forecast response to treatment using the mechanistic model. Results The responder classifier predicted endoscopic remission and mucosal healing for treatment with vedolizumab over 26 weeks, with overall sensitivities of 80% and 75% and overall specificities of 69% and 70%, respectively. Predictions for changes in tissue damage over time in the validation set ( n = 31), a measure of the overall performance of the platform, were considered good (at least 70% of data points matched), fair (at least 50%), and poor (less than 50%) for 71%, 23%, and 6% of patients, respectively. Conclusion Hybrid computational tools including mechanistic components represent a promising form of decision support that can predict outcomes and patient progress in Crohn’s disease.
更多查看译文
关键词
Computational model, Crohn’s disease, Mucosal healing
AI 理解论文
溯源树
样例
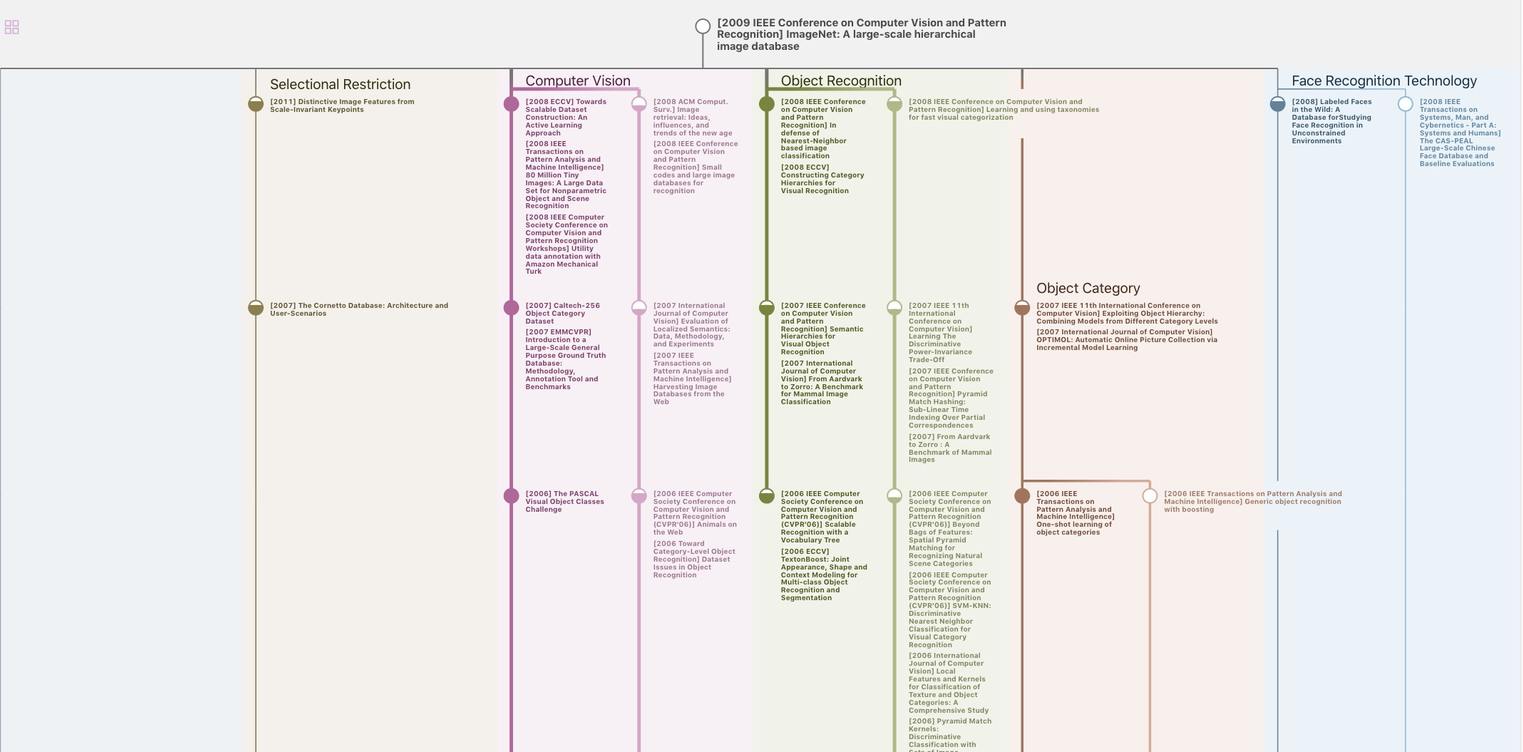
生成溯源树,研究论文发展脉络
Chat Paper
正在生成论文摘要