Nested Named Entity Recognition with Span-level Graphs
PROCEEDINGS OF THE 60TH ANNUAL MEETING OF THE ASSOCIATION FOR COMPUTATIONAL LINGUISTICS (ACL 2022), VOL 1: (LONG PAPERS)(2022)
摘要
Span-based methods with the neural networks backbone have great potential for the nested named entity recognition (NER) problem. However, they face problems such as degenerating when positive instances and negative instances largely overlap. Besides, the generalization ability matters a lot in nested NER, as a large proportion of entities in the test set hardly appear in the training set. In this work, we try to improve the span representation by utilizing retrieval-based span-level graphs, connecting spans and entities in the training data based on n-gram features. Specifically, we build the entity-entity graph and span-entity graph globally based on n-gram similarity to integrate the information of similar neighbor entities into the span representation. To evaluate our method, we conduct experiments on three common nested NER datasets, ACE2004, ACE2005, and GENIA datasets. Experimental results show that our method achieves general improvements on all three benchmarks (+0.30 similar to 0.85 micro-F1), and obtains special superiority on low frequency entities (+0.56 similar to 2.08 recall).
更多查看译文
关键词
entity recognition,graphs,span-level
AI 理解论文
溯源树
样例
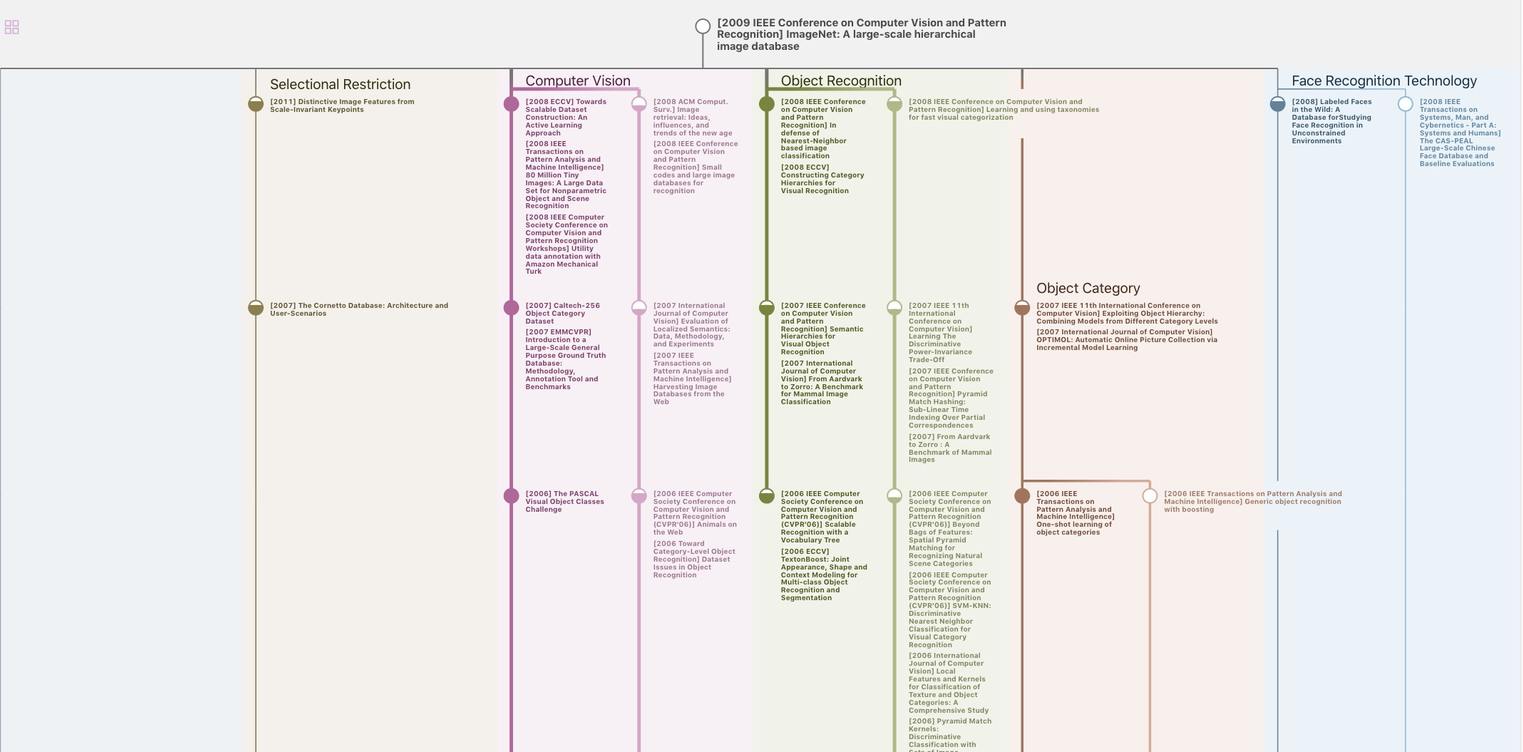
生成溯源树,研究论文发展脉络
Chat Paper
正在生成论文摘要