Adversarial Soft Prompt Tuning for Cross-Domain Sentiment Analysis
PROCEEDINGS OF THE 60TH ANNUAL MEETING OF THE ASSOCIATION FOR COMPUTATIONAL LINGUISTICS (ACL 2022), VOL 1: (LONG PAPERS)(2022)
Abstract
Cross-domain sentiment analysis has achieved promising results with the help of pre-trained language models. As GPT-3 appears, prompt tuning has been widely explored to enable better semantic modeling in many natural language processing tasks. However, directly using a fixed predefined template for cross-domain research cannot model different distributions of the [MASK] token in different domains, thus making underuse of the prompt tuning technique. In this paper, we propose a novel Adversarial Soft Prompt Tuning method (AdSPT) to better model cross-domain sentiment analysis. On the one hand, AdSPT adopts separate soft prompts instead of hard templates to learn different vectors for different domains, thus alleviating the domain discrepancy of the [MASK] token in the masked language modeling task. On the other hand, AdSPT uses a novel domain adversarial training strategy to learn domain-invariant representations between each source domain and the target domain. Experiments on a publicly available sentiment analysis dataset show that our model achieves new state-of-the-art results for both single-source domain adaptation and multi-source domain adaptation.
MoreTranslated text
AI Read Science
Must-Reading Tree
Example
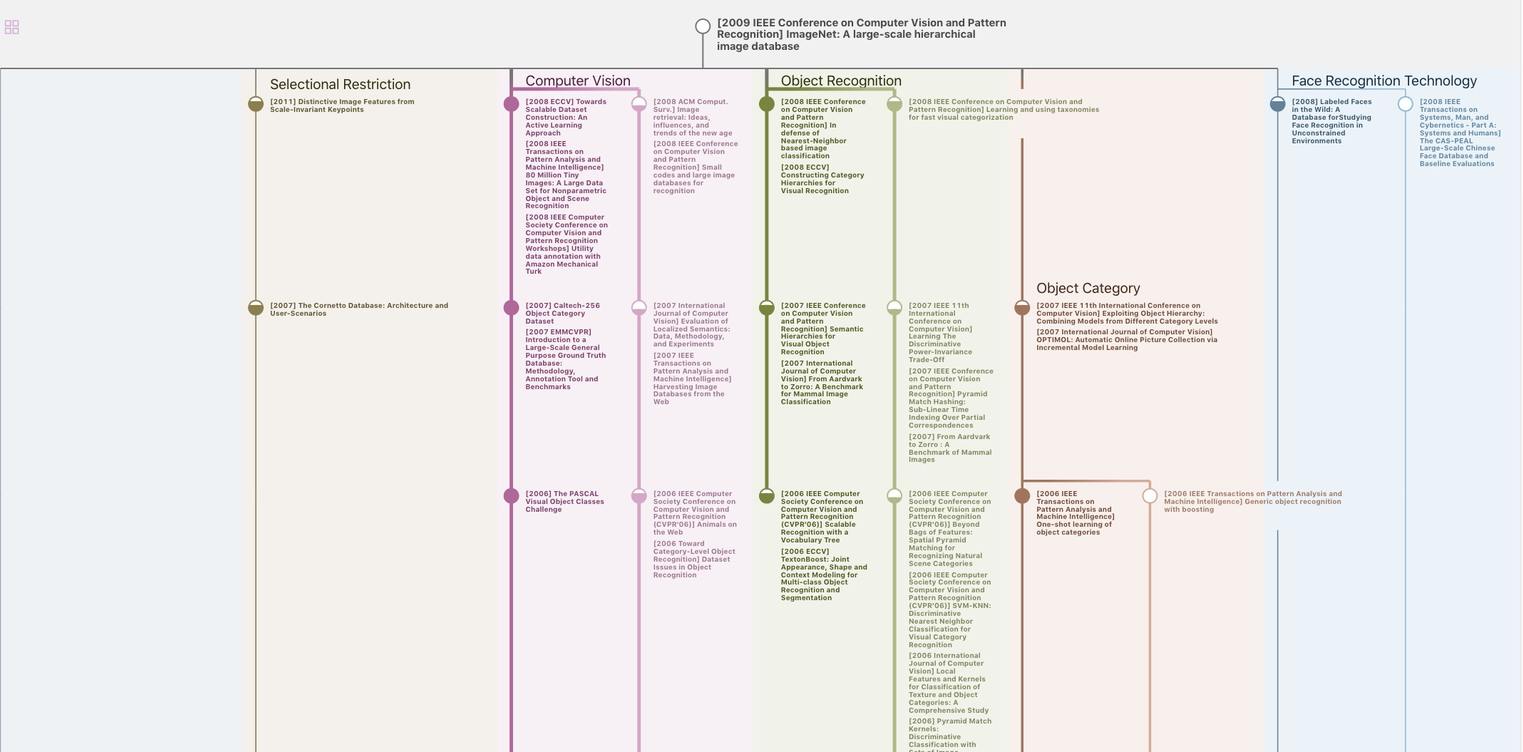
Generate MRT to find the research sequence of this paper
Chat Paper
Summary is being generated by the instructions you defined