Efficient Hyper-parameter Search for Knowledge Graph Embedding.
Annual Meeting of the Association for Computational Linguistics(2022)
摘要
While hyper-parameters (HPs) are important for knowledge graph (KG) learning, existing methods fail to search them efficiently. To solve this problem, we first analyze the properties of different HPs and measure the transfer ability from small subgraph to the full graph. Based on the analysis, we propose an efficient two-stage search algorithm KGTuner, which efficiently explores HP configurations on small subgraph at the first stage and transfers the top-performed configurations for fine-tuning on the large full graph at the second stage. Experiments show that our method can consistently find better HPs than the baseline algorithms within the same time budget, which achieves 9.1% average relative improvement for four embedding models on the large-scale KGs in open graph benchmark. Our code is released in https://github. com/AutoML-Research/KGTuner.
更多查看译文
关键词
knowledge graph,search,hyper-parameter
AI 理解论文
溯源树
样例
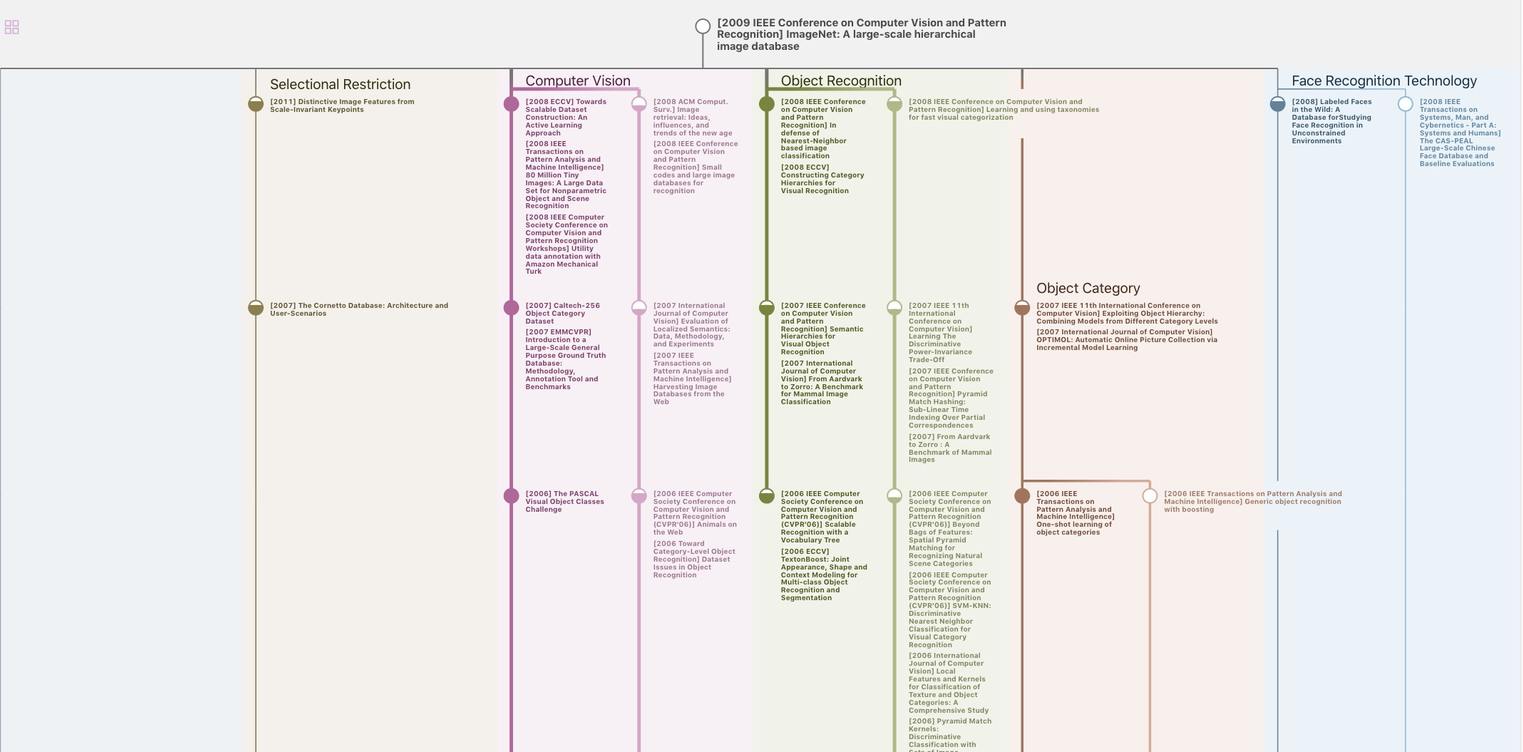
生成溯源树,研究论文发展脉络
Chat Paper
正在生成论文摘要