Keywords and Instances: A Hierarchical Contrastive Learning Framework Unifying Hybrid Granularities for Text Generation
PROCEEDINGS OF THE 60TH ANNUAL MEETING OF THE ASSOCIATION FOR COMPUTATIONAL LINGUISTICS (ACL 2022), VOL 1: (LONG PAPERS)(2022)
Abstract
Contrastive learning has achieved impressive success in generation tasks to militate the "exposure bias" problem and discriminatively exploit the different quality of references. Existing works mostly focus on contrastive learning on the instance-level without discriminating the contribution of each word, while keywords are the gist of the text and dominant the constrained mapping relationships. Hence, in this work, we propose a hierarchical contrastive learning mechanism, which can unify hybrid granularities semantic meaning in the input text. Concretely, we first propose a keyword graph via contrastive correlations of positive-negative pairs to iteratively polish the keyword representations. Then, we construct intra-contrasts within instance-level and keyword-level, where we assume words are sampled nodes from a sentence distribution. Finally, to bridge the gap between independent contrast levels and tackle the common contrast vanishing problem, we propose an inter-contrast mechanism that measures the discrepancy between contrastive keyword nodes respectively to the instance distribution. Experiments demonstrate that our model outperforms competitive baselines on paraphrasing, dialogue generation, and storytelling tasks.
MoreTranslated text
Key words
text generation,hierarchical contrastive learning framework
AI Read Science
Must-Reading Tree
Example
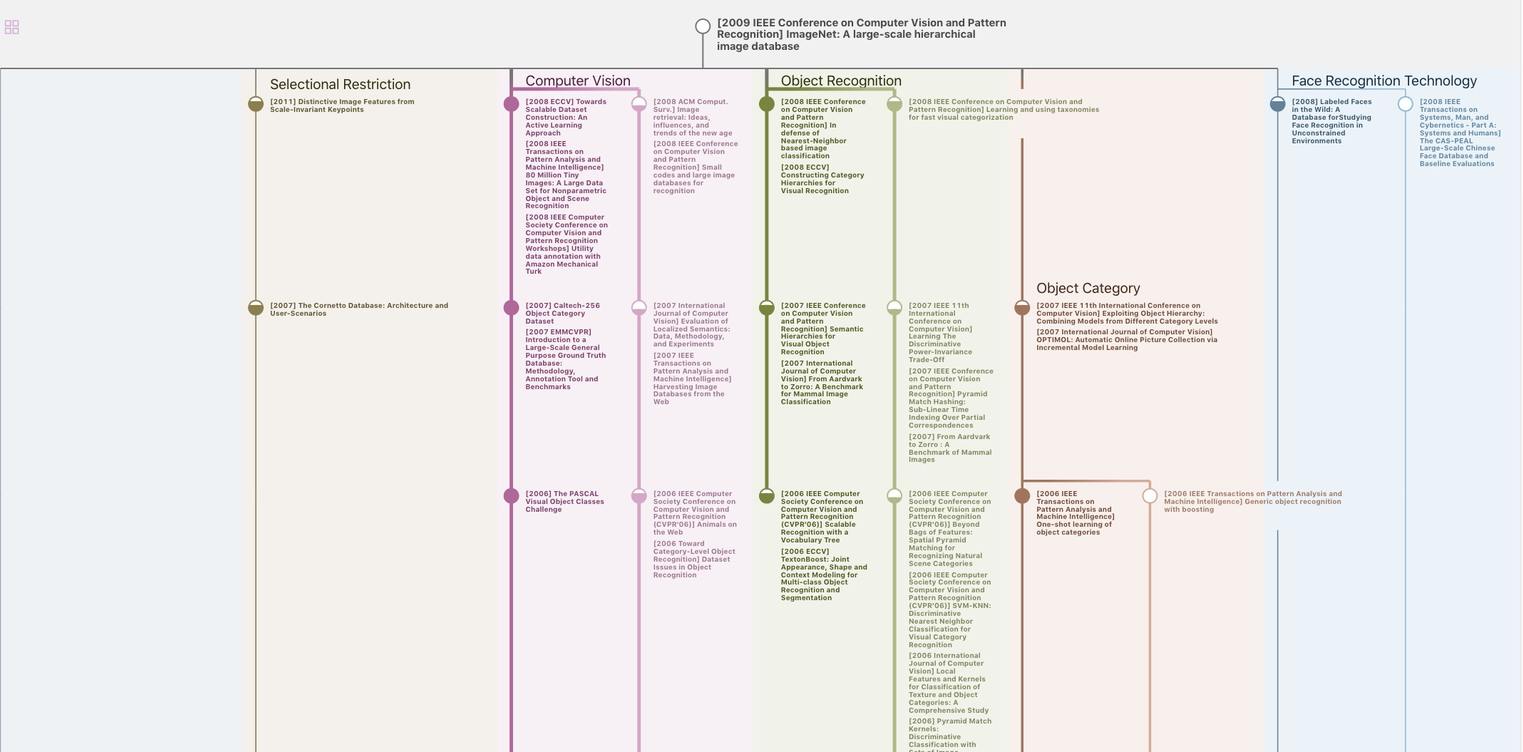
Generate MRT to find the research sequence of this paper
Chat Paper
Summary is being generated by the instructions you defined