Unsupervised multiple-choice question generation for out-of-domain Q&A fine-tuning
PROCEEDINGS OF THE 60TH ANNUAL MEETING OF THE ASSOCIATION FOR COMPUTATIONAL LINGUISTICS (ACL 2022): (SHORT PAPERS), VOL 2(2022)
摘要
Pre-trained models have shown very good performances on a number of question answering benchmarks especially when fine-tuned on multiple question answering datasets at once. In this work, we propose an approach for generating a fine-tuning dataset thanks to a rule-based algorithm that generates questions and answers from unannotated sentences. We show that the state-of-the-art model UnifiedQA can greatly benefit from such a system on a multiple-choice benchmark about physics, biology and chemistry it has never been trained on. We further show that improved performances may be obtained by selecting the most challenging distractors (wrong answers), with a dedicated ranker based on a pretrained RoBERTa model.
更多查看译文
关键词
generation,multiple-choice,out-of-domain,fine-tuning
AI 理解论文
溯源树
样例
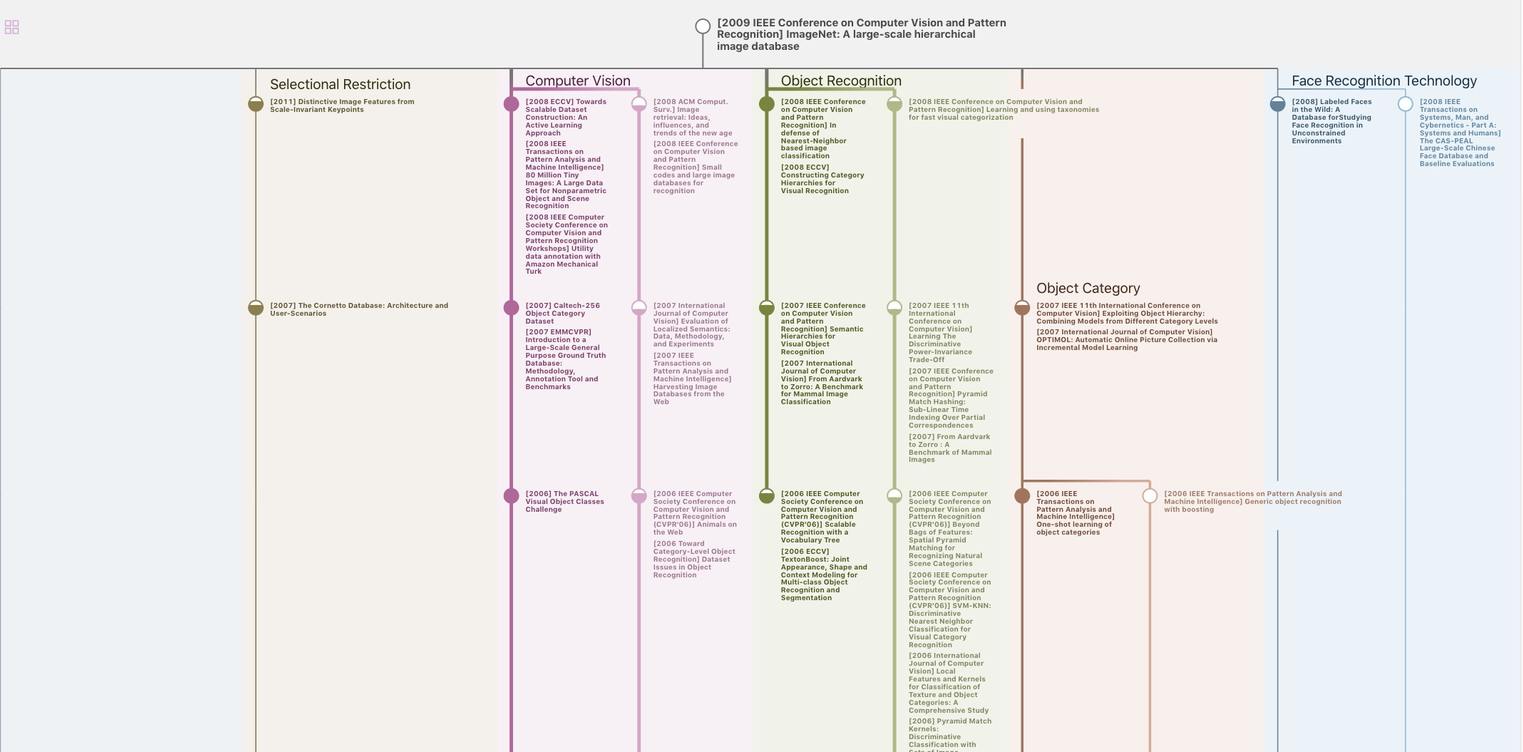
生成溯源树,研究论文发展脉络
Chat Paper
正在生成论文摘要