Guided K-best Selection for Semantic Parsing Annotation
PROCEEDINGS OF THE 60TH ANNUAL MEETING OF THE ASSOCIATION FOR COMPUTATIONAL LINGUISTICS (ACL 2022): PROCEEDINGS OF SYSTEM DEMONSTRATIONS(2022)
摘要
Collecting data for conversational semantic parsing is a time-consuming and demanding process. In this paper we consider, given an incomplete dataset with only a small amount of data, how to build an AI-powered humanin-the-loop process to enable efficient data collection. A guided K-best selection process is proposed, which (i) generates a set of possible valid candidates; (ii) allows users to quickly traverse the set and filter incorrect parses; and (iii) asks users to select the correct parse, with minimal modification when necessary. We investigate how to best support users in efficiently traversing the candidate set and locating the correct parse, in terms of speed and accuracy. In our user study, consisting of five annotators labeling 300 instances each, we find that combining keyword searching, where keywords can be used to query relevant candidates, and keyword suggestion, where representative keywords are automatically generated, enables fast and accurate annotation.(1)
更多查看译文
AI 理解论文
溯源树
样例
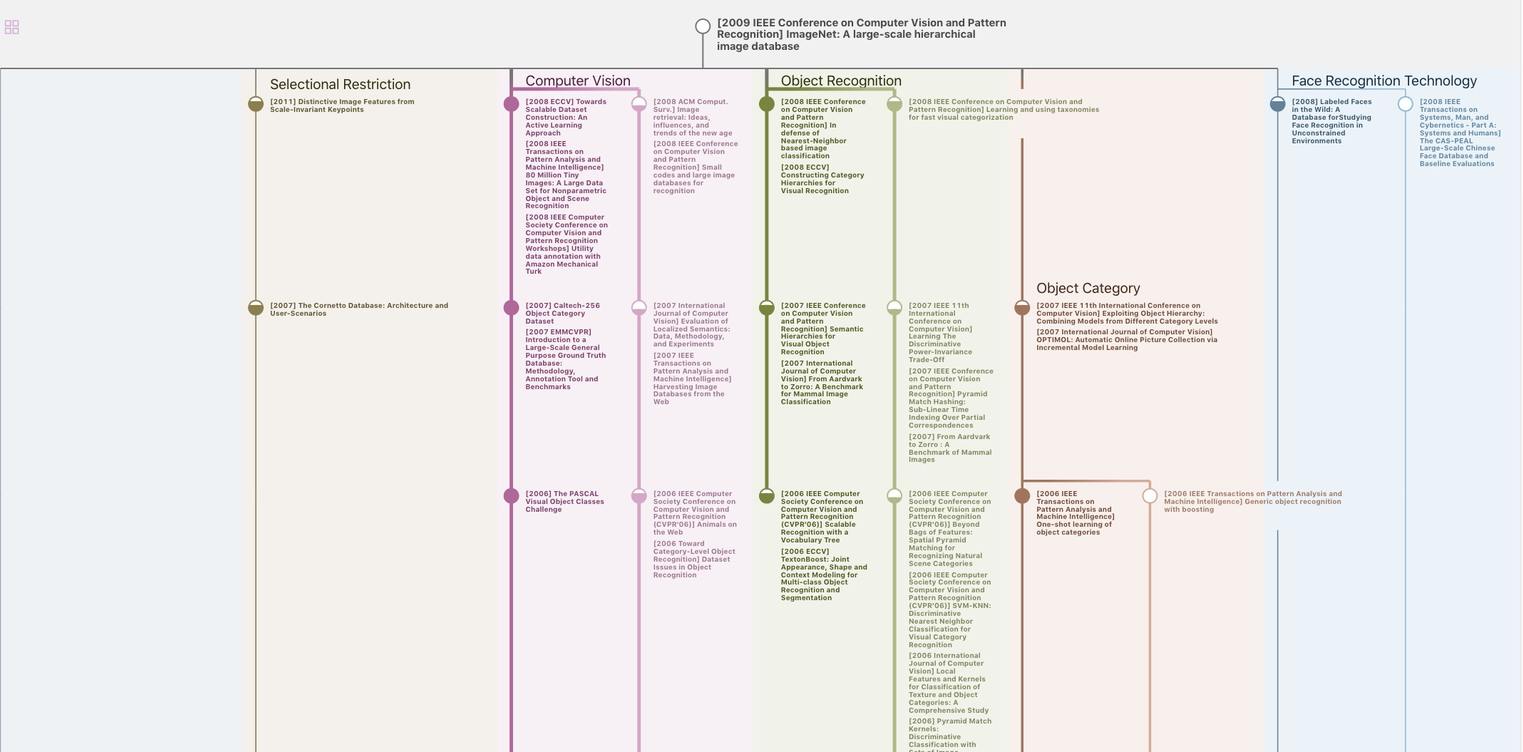
生成溯源树,研究论文发展脉络
Chat Paper
正在生成论文摘要