Inter-annotator Agreement is Not the Ceiling of Machine Learning Performance: Evidence from a Comprehensive Set of Simulations
Proceedings of the 21st Workshop on Biomedical Language Processing(2022)
AI 理解论文
溯源树
样例
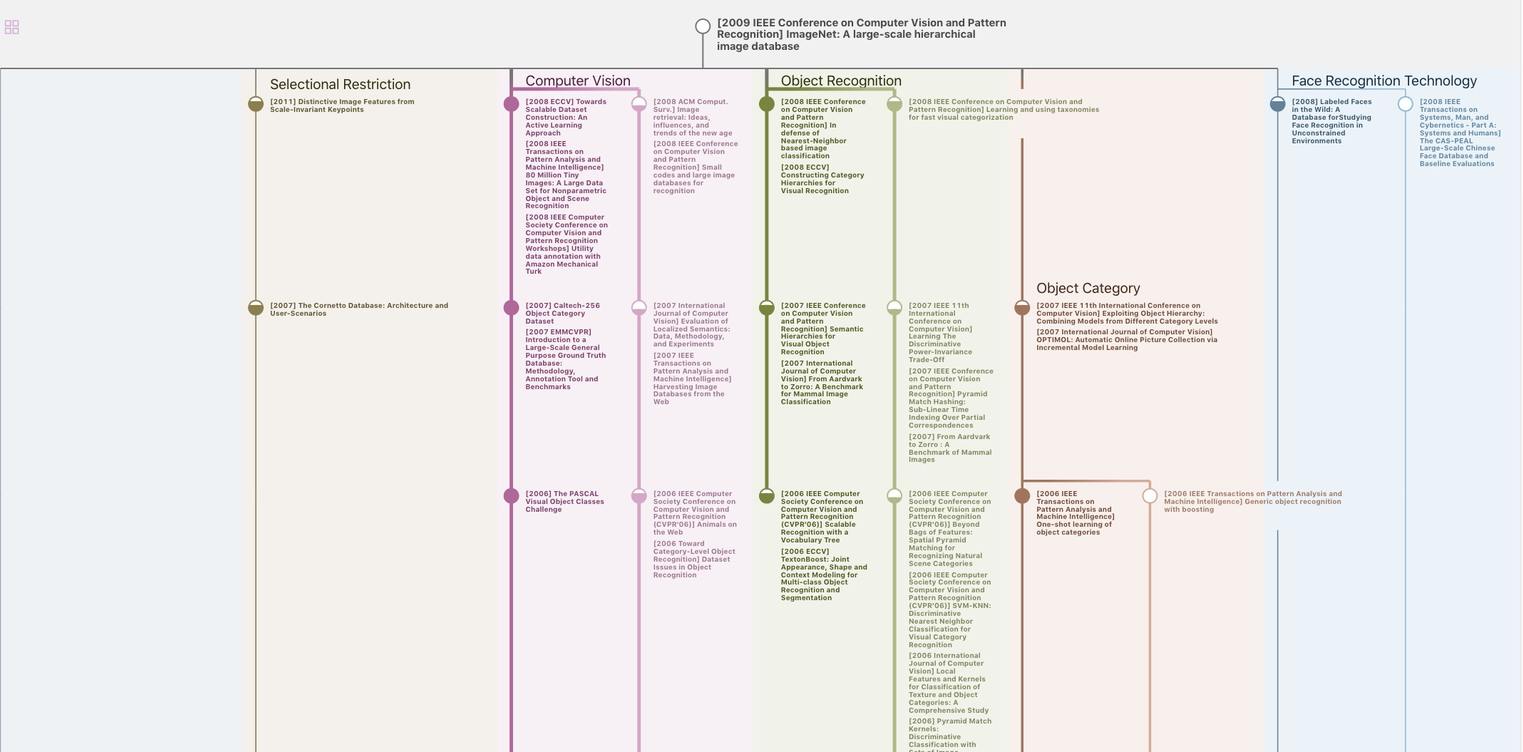
生成溯源树,研究论文发展脉络
Chat Paper
正在生成论文摘要
Proceedings of the 21st Workshop on Biomedical Language Processing(2022)