OpenBrand: Open Brand Value Extraction from Product Descriptions
PROCEEDINGS OF THE 5TH WORKSHOP ON E-COMMERCE AND NLP (ECNLP 5)(2022)
摘要
Extracting attribute-value information from unstructured product descriptions continue to be of a vital importance in e-commerce applications. One of the most important product attributes is the brand which highly influences customers' purchasing behaviour. Thus, it is crucial to accurately extract brand information dealing with the main challenge of discovering new brand names. Under the open world assumption, several approaches have adopted deep learning models to extract attribute-values using sequence tagging paradigm. However, they did not employ finer grained data representations such as character level embeddings which improve generalizability. In this paper, we introduce OpenBrand, a novel approach for discovering brand names. OpenBrand is a BiLSTM-CRF-Attention model with embeddings at different granularities. Such embeddings are learned using CNN and LSTM architectures to provide more accurate representations. We further propose a new dataset for brand value extraction, with a very challenging task on zero-shot extraction. We have tested our approach, through extensive experiments, and shown that it outperforms state-of-the-art models in brand name discovery.
更多查看译文
AI 理解论文
溯源树
样例
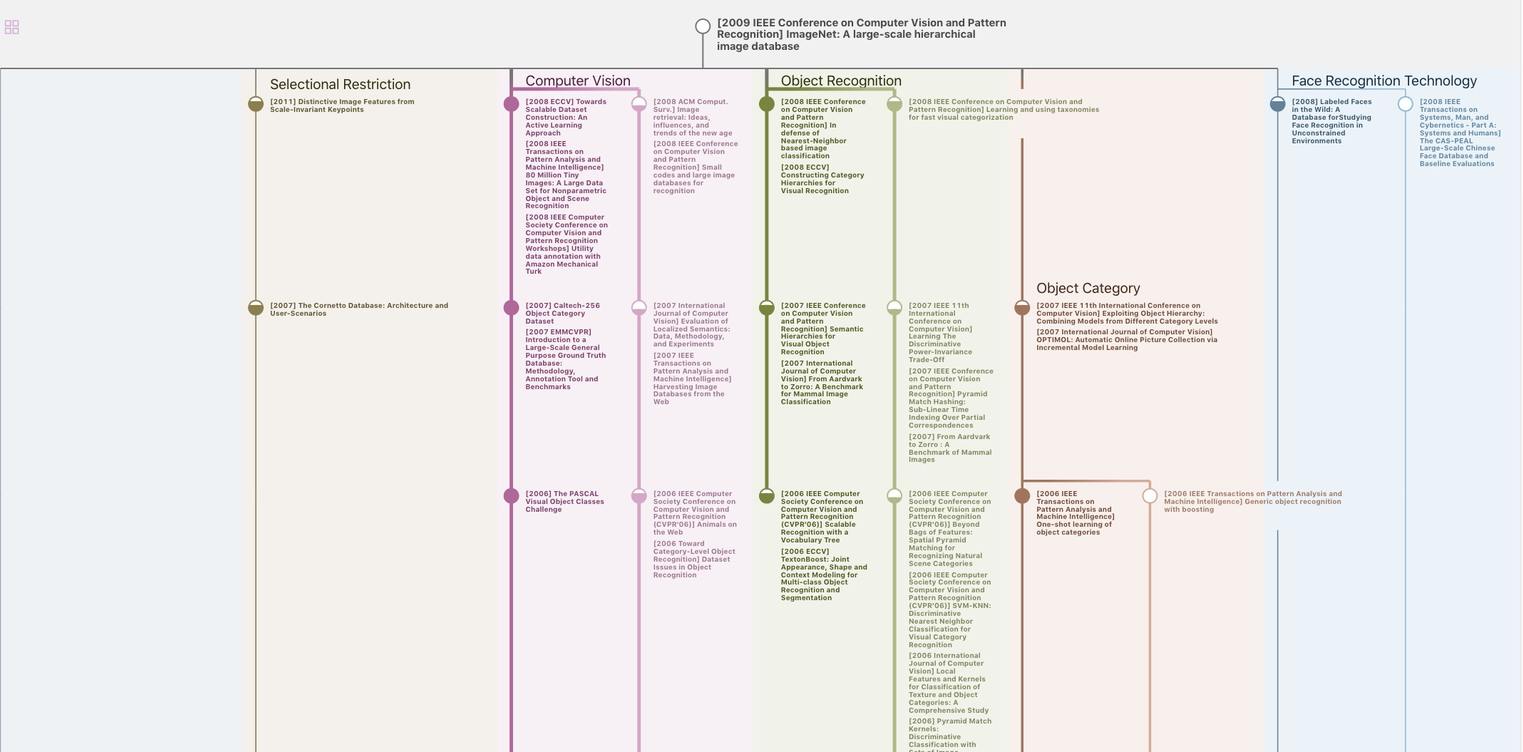
生成溯源树,研究论文发展脉络
Chat Paper
正在生成论文摘要